On the effect of function set to the generalisation of symbolic regression models.
GECCO (Companion)(2018)
摘要
Supervised learning by means of Genetic Programming aims at the evolutionary synthesis of a model that achieves a balance between approximating the target function on the training data and generalising on new data. In this study we benchmark the approximation / generalisation of models evolved using different function set setups, across a range of symbolic regression problems. Results show that Koza's protected division and power should be avoided, and operators such as analytic quotient and sine should be used instead.
更多查看译文
关键词
generalisation,models,function
AI 理解论文
溯源树
样例
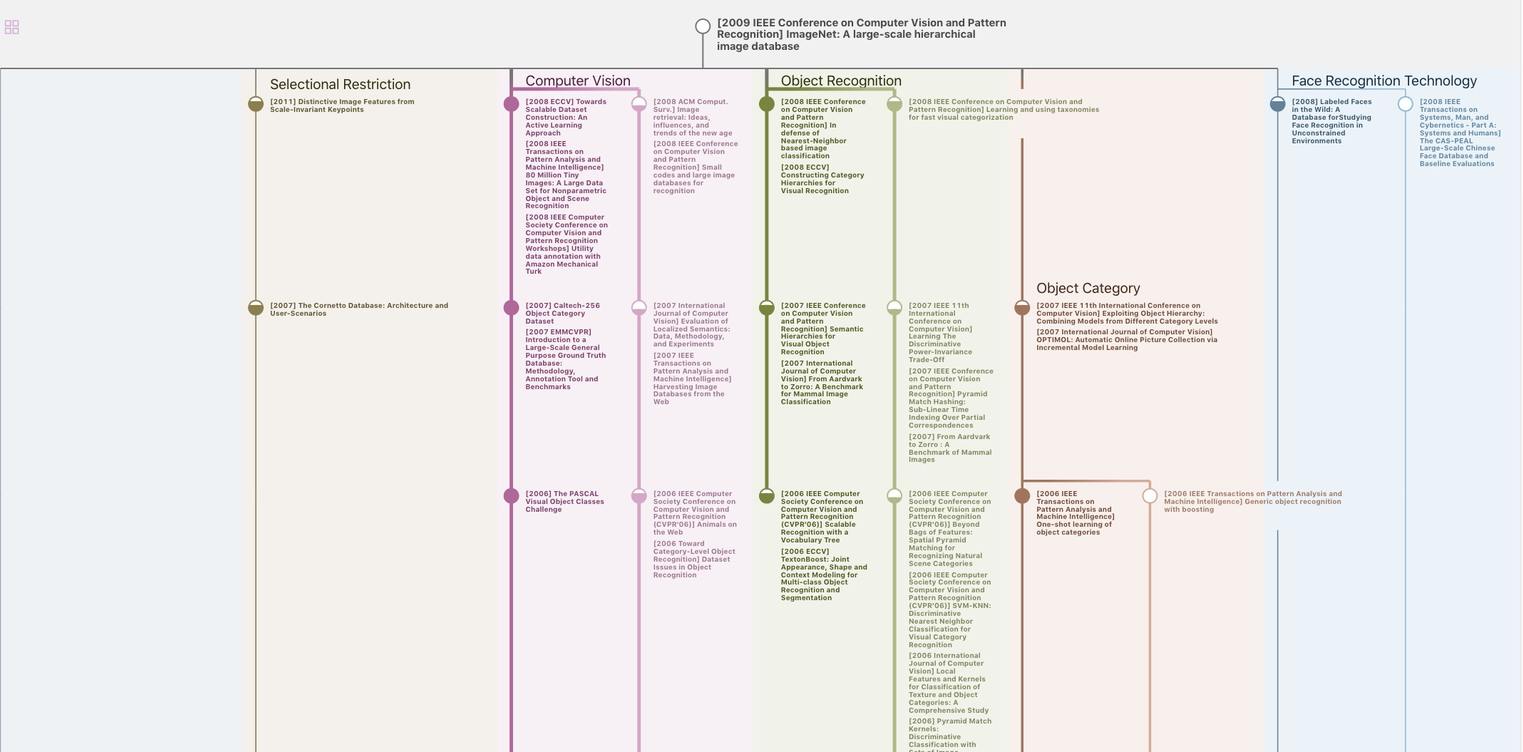
生成溯源树,研究论文发展脉络
Chat Paper
正在生成论文摘要