Semi-Automatic Ontology Population for Online Quality Assessment of Particulate Matter Sensors.
INTELLIGENT ENVIRONMENTS 2018(2018)
摘要
The increasing coverage and heterogeneity of sensor-based systems due to the low acquisition cost, ease of deployment and data collection makes the range of possible applications in the context of smart cities bigger. This makes the process of quality measurement of the generated data as well as anomaly detection some of the toughest challenges to overcome. The absence of a common mean to express general information about the sensor deployment, measurement capabilities, output data and the conditions, under which the sensor could produce anomalies, casts a big shadow over the trustworthiness of the data. The large number of low cost sensors makes the description of their context information even more difficult. We propose an architecture that enables the semi-automatic creation of context information about the sensors through the use of available information about the output data and the available information about the deployment. The definition of the sensor with its relevant context information is done by populating ontology instances for every sensor using the SSN and SWEET ontologies. We take the use case of the luftdaten.info platform with particulate matter sensors. This architecture with its implementation lay the groundwork for further steps such as including anomaly detection rules and quality measurement conditions into the sensor model. The ontology instance produced is the input used for automatic generation of data stream processing queries with data quality assessment.
更多查看译文
关键词
Data Stream Processing,Data Quality,Sensor Networks,Ontologies,Context Modelling
AI 理解论文
溯源树
样例
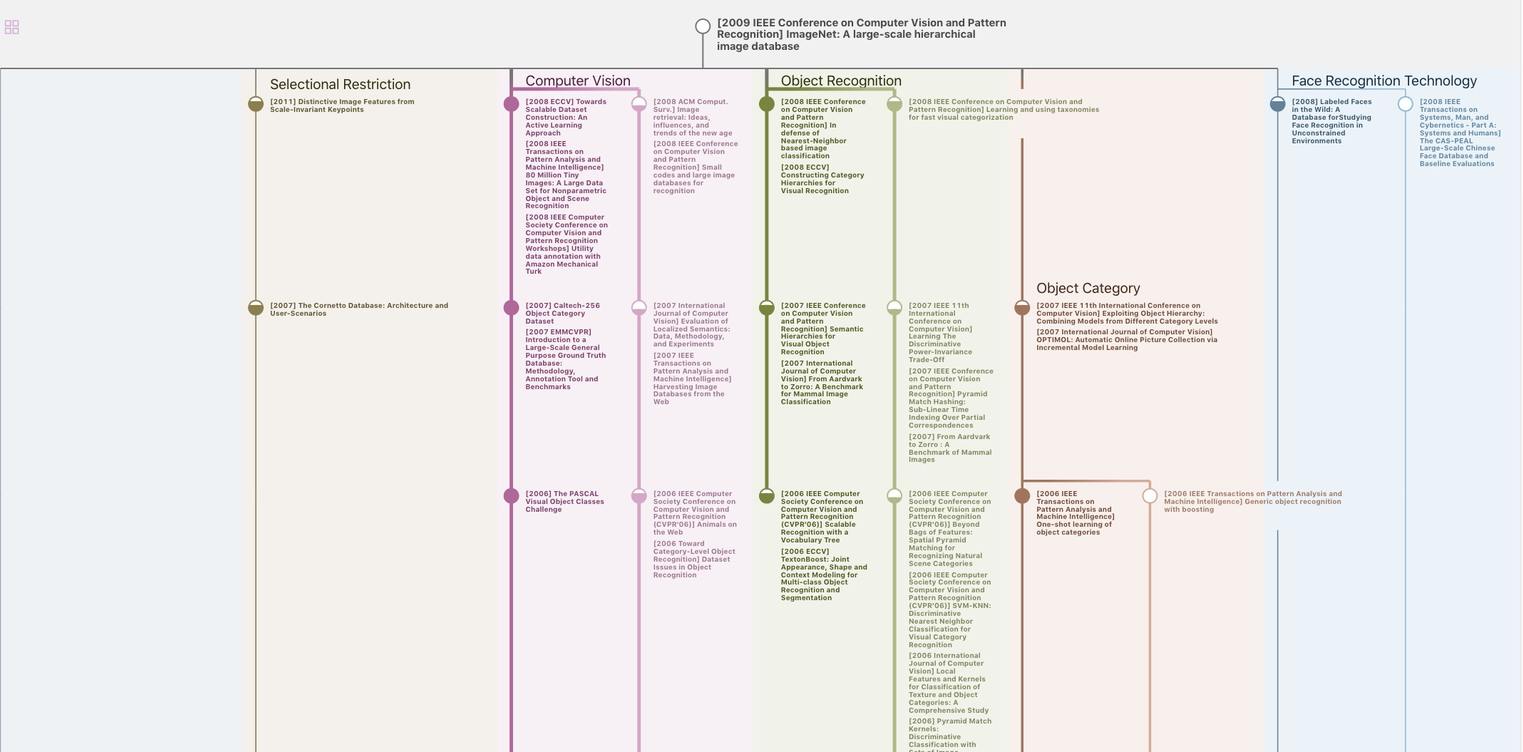
生成溯源树,研究论文发展脉络
Chat Paper
正在生成论文摘要