Stock Price Movement Prediction from Financial News with Deep Learning and Knowledge Graph Embedding
KNOWLEDGE MANAGEMENT AND ACQUISITION FOR INTELLIGENT SYSTEMS (PKAW 2018)(2018)
摘要
As the technology applied to economy develops, more and more investors are paying attention to stock prediction. Therefore, research on stock prediction is becoming a hot area. In this paper, we propose to incorporate a joint model using the TransE model for representation learning and a Convolutional Neural Network (CNN), which extracts features from financial news articles. This joint learning can improve the accuracy of text feature extraction while reducing the sparseness of news headlines. On the other hand, we present a joint feature extraction method which extracts feature vectors from both daily trading data and technical indicators. The approach is evaluated using Support Vector Machines (SVM) as a traditional machine learning method and Long Short-term Memory (LSTM) model as a deep learning method. The proposed model is used to predict Apple’s stock price movement using the Standard & Poor’s 500 index (S&P 500). The experiments show that the accuracy of news sentiment classification for feature selection achieved 97.66% by model of joint learning, the performance of joint learning is better than feature extraction by CNN, the accuracy of stock price movement prediction through deep learning achieved 55.44%, this result is higher than traditional machine learning. This model can give the investors greater decision support.
更多查看译文
关键词
Stock market,Deep learning,Event tuple,Financial news,Knowledge graph embedding
AI 理解论文
溯源树
样例
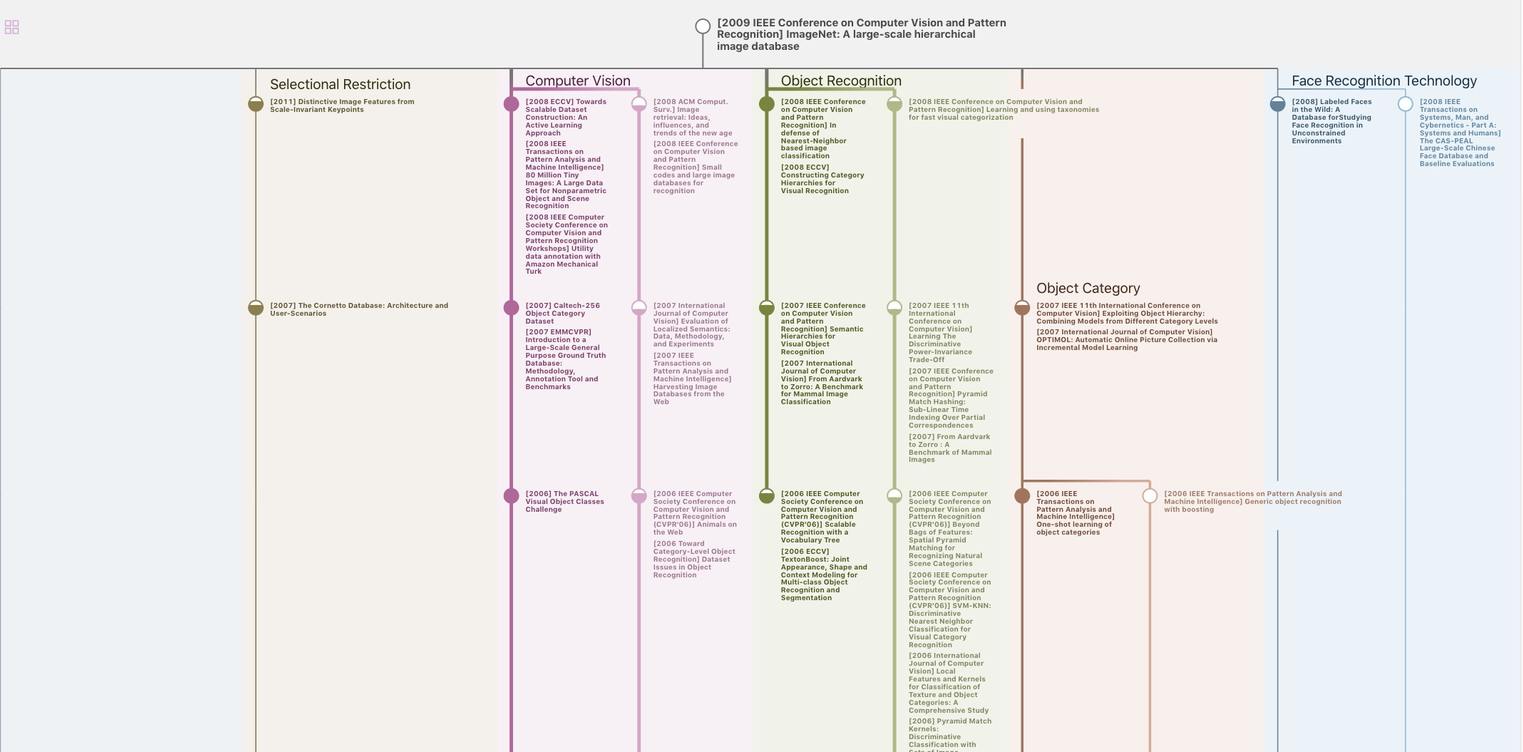
生成溯源树,研究论文发展脉络
Chat Paper
正在生成论文摘要