Attention-Based Recurrent Neural Network For Sequence Labeling
WEB AND BIG DATA (APWEB-WAIM 2018), PT I(2018)
摘要
Sequence labeling is one of the key problems in natural language processing. Recently, Recurrent Neural Network (RNN) and its variations have been widely used for this task. Despite their abilities of encoding information from long distance, in practice, one single hidden layer is still not sufficient for prediction. In this paper, we propose an attention architecture for sequence labeling, which allows RNNs to selectively focus on every useful hidden layers instead of irrelative ones. We conduct experiments on four typical sequence labeling tasks, including Part-Of-Speech Tagging (POS), Chunking, Named Entity Recognition (NER), and Slot Filling for Spoken Language Understanding (SF-SLU). Comprehensive experiments show that our attention architecture provides consistent improvements over different RNN variations.
更多查看译文
AI 理解论文
溯源树
样例
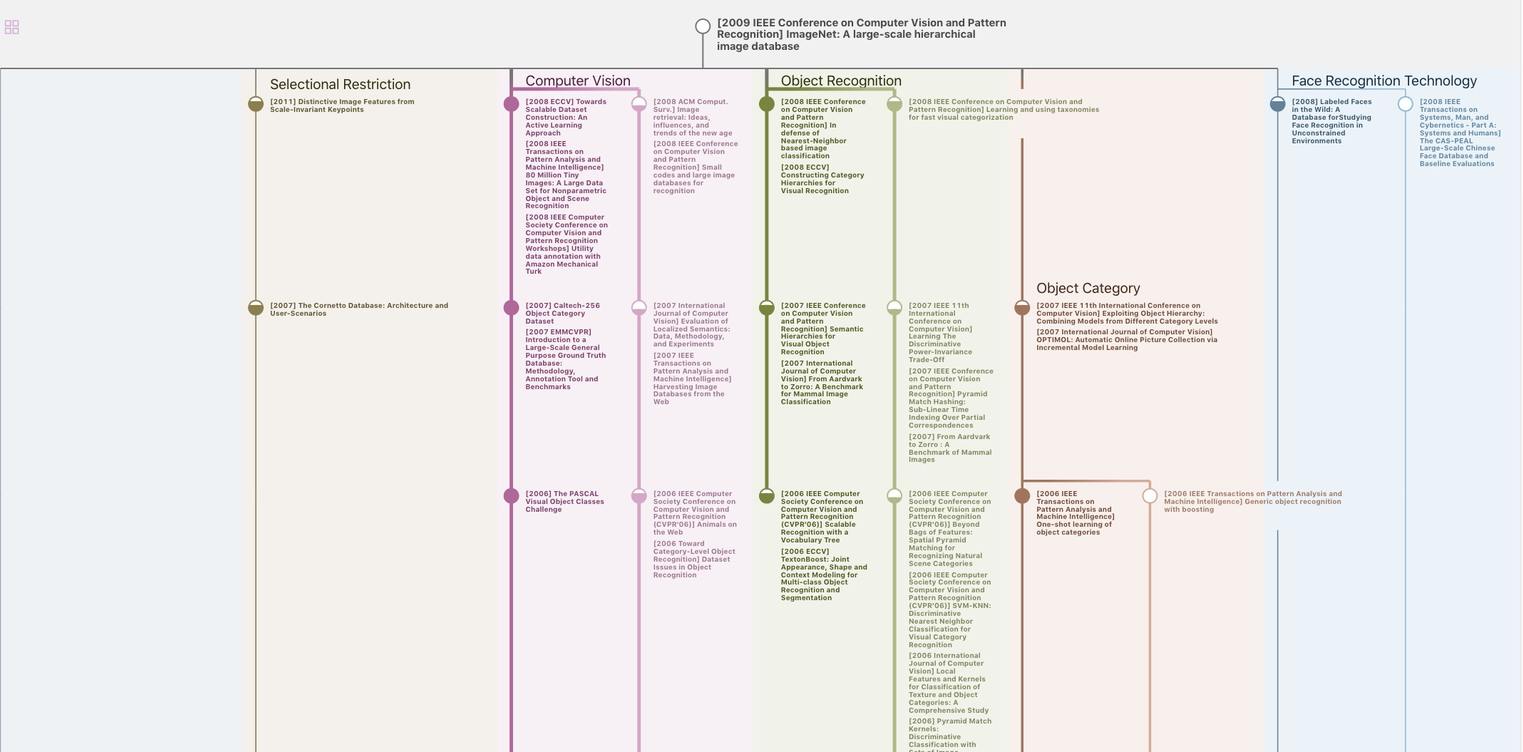
生成溯源树,研究论文发展脉络
Chat Paper
正在生成论文摘要