Classifying and Counting with Recurrent Contexts.
KDD(2018)
摘要
Many real-world applications in the batch and data stream settings with data shift pose restrictions to the access to class labels after the deployment of a classification or quantification model. However, a significant portion of the data stream literature assumes that actual labels are instantaneously available after issuing their corresponding classifications. In this paper, we explore a different set of assumptions without relying on the availability of class labels. We assume that, although the distribution of the data may change over time, it will switch between one of a handful of well-known distributions. Still, we allow the proportions of the classes to vary. In these conditions, we propose the first method that can accurately identify the correct context of data samples and simultaneously estimate the proportion of the positive class. This estimate can be further used to adjust a classification decision threshold and improve classification accuracy. Finally, the method is very efficient regarding time and memory requirements, fitting data stream applications.
更多查看译文
关键词
Classification,Counting,Quantification,Concept Drift,Dataset Shift,Recurrent Contexts
AI 理解论文
溯源树
样例
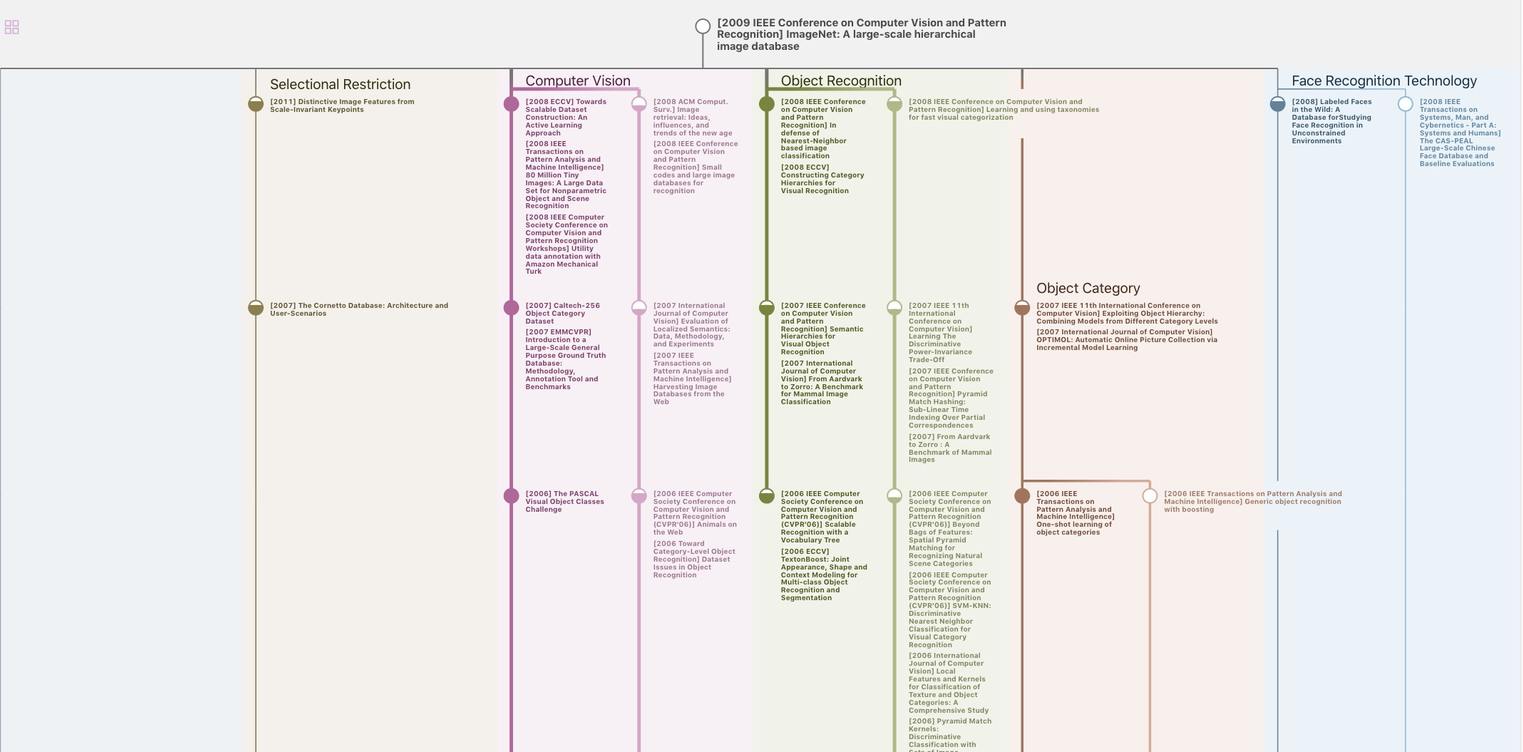
生成溯源树,研究论文发展脉络
Chat Paper
正在生成论文摘要