Using Rule-Based Labels for Weak Supervised Learning: A ChemNet for Transferable Chemical Property Prediction.
KDD(2018)
摘要
With access to large datasets, deep neural networks (DNN) have achieved human-level accuracy in image and speech recognition tasks. However, in chemistry data is inherently small and fragmented. In this work, we develop an approach of using rule-based knowledge for training ChemNet, a transferable and generalizable deep neural network for chemical property prediction that learns in a weak-supervised manner from large unlabeled chemical databases. When coupled with transfer learning approaches to predict other smaller datasets for chemical properties that it was not originally trained on, we show that ChemNet's accuracy outperforms contemporary DNN models that were trained using conventional supervised learning. Furthermore, we demonstrate that the ChemNet pre-training approach is equally effective on both CNN (Chemception) and RNN (SMILES2vec) models, indicating that this approach is network architecture agnostic and is effective across multiple data modalities. Our results indicate a pre-trained ChemNet that incorporates chemistry domain knowledge and enables the development of generalizable neural networks for more accurate prediction of novel chemical properties.
更多查看译文
关键词
Bioinformatics,Cheminformatics,Computer Vision,Natural Language Processing,Transfer Learning,Weak Supervised Learning
AI 理解论文
溯源树
样例
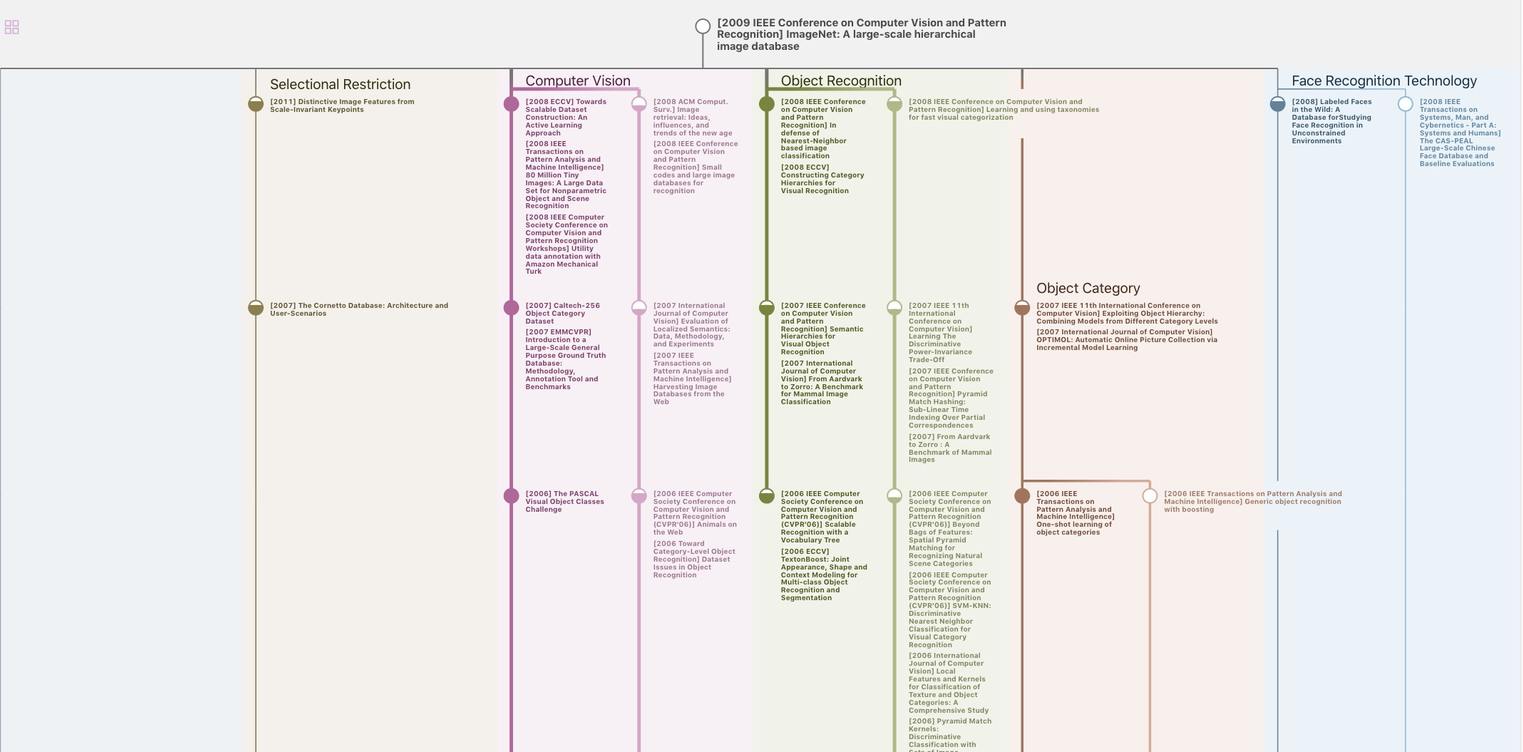
生成溯源树,研究论文发展脉络
Chat Paper
正在生成论文摘要