Variational based Mixed Noise Removal with CNN Deep Learning Regularization.
IEEE transactions on image processing : a publication of the IEEE Signal Processing Society(2020)
摘要
In this paper, the traditional model based variational methods and deep learning based algorithms are naturally integrated to address mixed noise removal, specially for Gaussian mixture noise and Gaussian-impulse noise removal problem. To be different from single type noise (e.g. Gaussian) removal, it is a challenge problem to accurately discriminate noise types and levels for each pixel. We propose a variational method to iteratively estimate the noise parameters, and then the algorithm can automatically classify the noise according to the different statistical parameters. The proposed variational problem can be separated into regularization, synthesis, parameters estimation and noise classification four steps with the operator splitting scheme. Each step is related to an optimization subproblem. To enforce the regularization, the deep learning method is employed to learn the natural images prior. Compared with some model based regularizations, the CNN regularizer can significantly improve the quality of the restored images. Compared with some learning based methods, the synthesis step can produce better reconstructions by analyzing the types and levels of the recognized noise. In our method, the convolution neutral network (CNN) can be regarded as an operator which associated to a variational functional. From this viewpoint, the proposed method can be extended to many image reconstruction and inverse problems. Numerical experiments in the paper show that our method can achieve some state-of-the-art results for Gaussian mixture noise and Gaussian-impulse noise removal.
更多查看译文
关键词
Deep learning,Noise reduction,Image restoration,Learning systems,TV,Data models
AI 理解论文
溯源树
样例
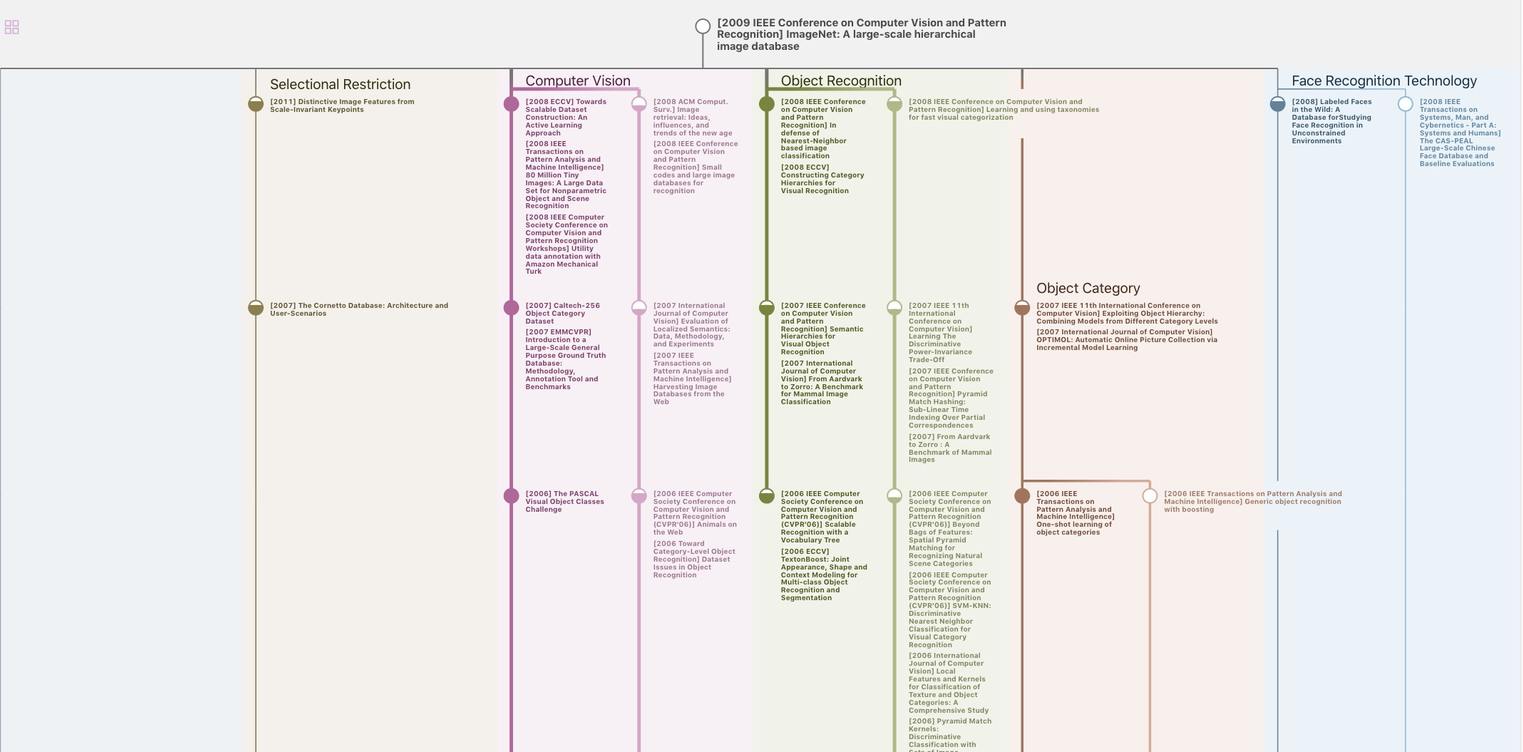
生成溯源树,研究论文发展脉络
Chat Paper
正在生成论文摘要