How Important Is a Neuron?
international conference on learning representations(2019)
摘要
The problem of attributing a deep networku0027s prediction to its emph{input/base} features is well-studied. We introduce the notion of emph{conductance} to extend the notion of attribution to the understanding the importance of emph{hidden} units. Informally, the conductance of a hidden unit of a deep network is the emph{flow} of attribution via this hidden unit. We use conductance to understand the importance of a hidden unit to the prediction for a specific input, or over a set of inputs. We evaluate the effectiveness of conductance in multiple ways, including theoretical properties, ablation studies, and a feature selection task. The empirical evaluations are done using the Inception network over ImageNet data, and a sentiment analysis network over reviews. In both cases, we demonstrate the effectiveness of conductance in identifying interesting insights about the internal workings of these networks.
更多查看译文
AI 理解论文
溯源树
样例
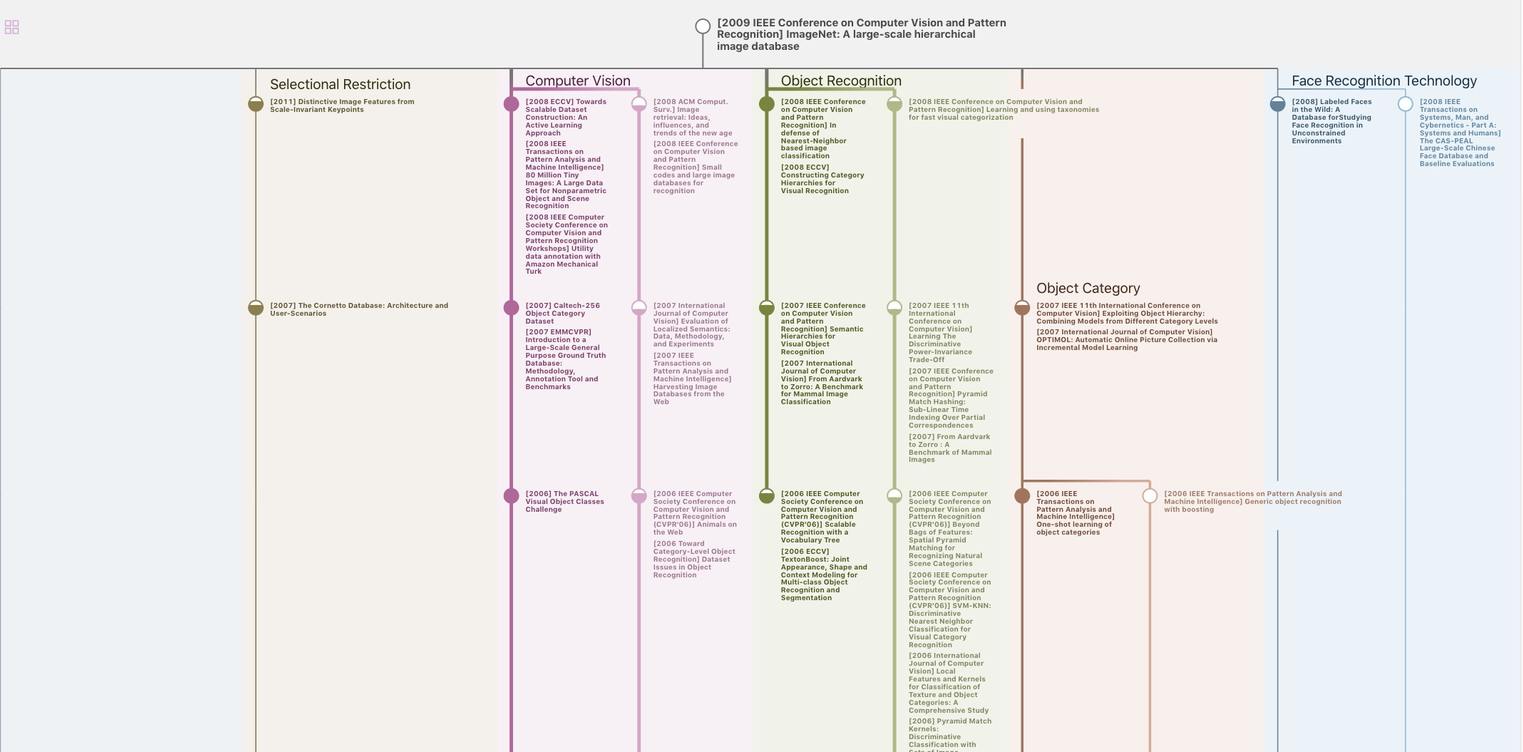
生成溯源树,研究论文发展脉络
Chat Paper
正在生成论文摘要