Does Deeper Network Lead to Better Accuracy: A Case Study on Handwritten Devanagari Characters
2018 13th IAPR International Workshop on Document Analysis Systems (DAS)(2018)
摘要
Deep neural network architectures have been used successfully in various document analysis studies. Its strength in producing human like performance has already been explored in handwritten English numeral recognition task. In this context, a natural question that often arises in a practitioner's mind: does an increase in the depth of the network eventually lead to an improved recognition performance on unknown samples? A goal of the present work is to search for an answer of the same through a case study of a larger class handwriting recognition problem. Here, we have studied recognition of handwritten Devanagari characters. In this study, we have implemented convolutional neural network (CNN) architectures of five different depths. We have also implemented additional neural architectures by adding two Bidirectional Long Short Term Memory (BLSTM) layers between the convolutional stack and the fully connected part of each of these five CNN networks. Simulations have been performed on two different databases of handwritten Devanagari characters consisting of 30408 and 36172 samples and a combined set consisting of 58451 samples. The recognition accuracy obtained in the best case improves significantly the existing state-of-the-art of this handwriting recognition problem. Also, further analysis of our simulation results provides an answer to the above question. Additionally, we have trained a BLSTM network alone using the Histogram of Oriented Gradient (HOG) features. Performance of this architecture failed to compete with the performance of CNN-BLSTM hybrid architecture.
更多查看译文
关键词
CNN,BLSTM,handwriting recognition,Devanagari character recognition
AI 理解论文
溯源树
样例
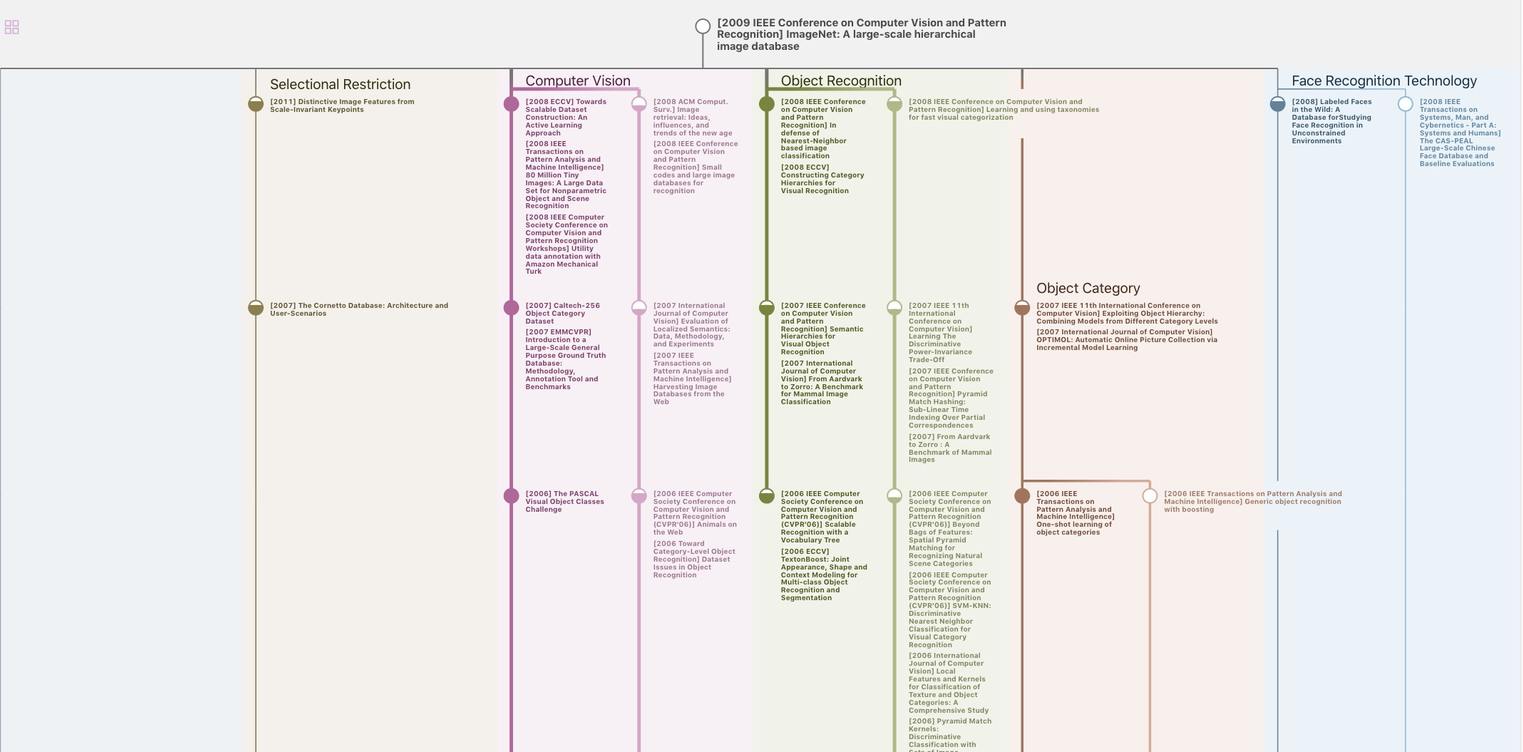
生成溯源树,研究论文发展脉络
Chat Paper
正在生成论文摘要