Episodesupport: A Global Constraint For Mining Frequent Patterns In A Long Sequence Of Events
INTEGRATION OF CONSTRAINT PROGRAMMING, ARTIFICIAL INTELLIGENCE, AND OPERATIONS RESEARCH, CPAIOR 2018(2018)
摘要
The number of applications generating sequential data is exploding. This work studies the discovering of frequent patterns in a large sequence of events, possibly time-stamped. This problem is known as the Frequent Episode Mining (FEM). Similarly to the mining problems recently tackled by Constraint Programming (CP), FEM would also benefit from the modularity offered by CP to accommodate easily additional constraints on the patterns. These advantages do not offer a guarantee of efficiency. Therefore, we introduce two global constraints for solving FEM problems with or without time consideration. The time-stamped version can accommodate gap and span constraints on the matched sequences. Our experiments on real data sets of different levels of complexity show that the introduced constraints is competitive with the state-of-the-art methods in terms of execution time and memory consumption while offering the flexibility of adding constraints on the patterns.
更多查看译文
关键词
Global Constraints, Frequent Episode Mining (FEM), Span Constraint, Opposite Vectors, Cache Position
AI 理解论文
溯源树
样例
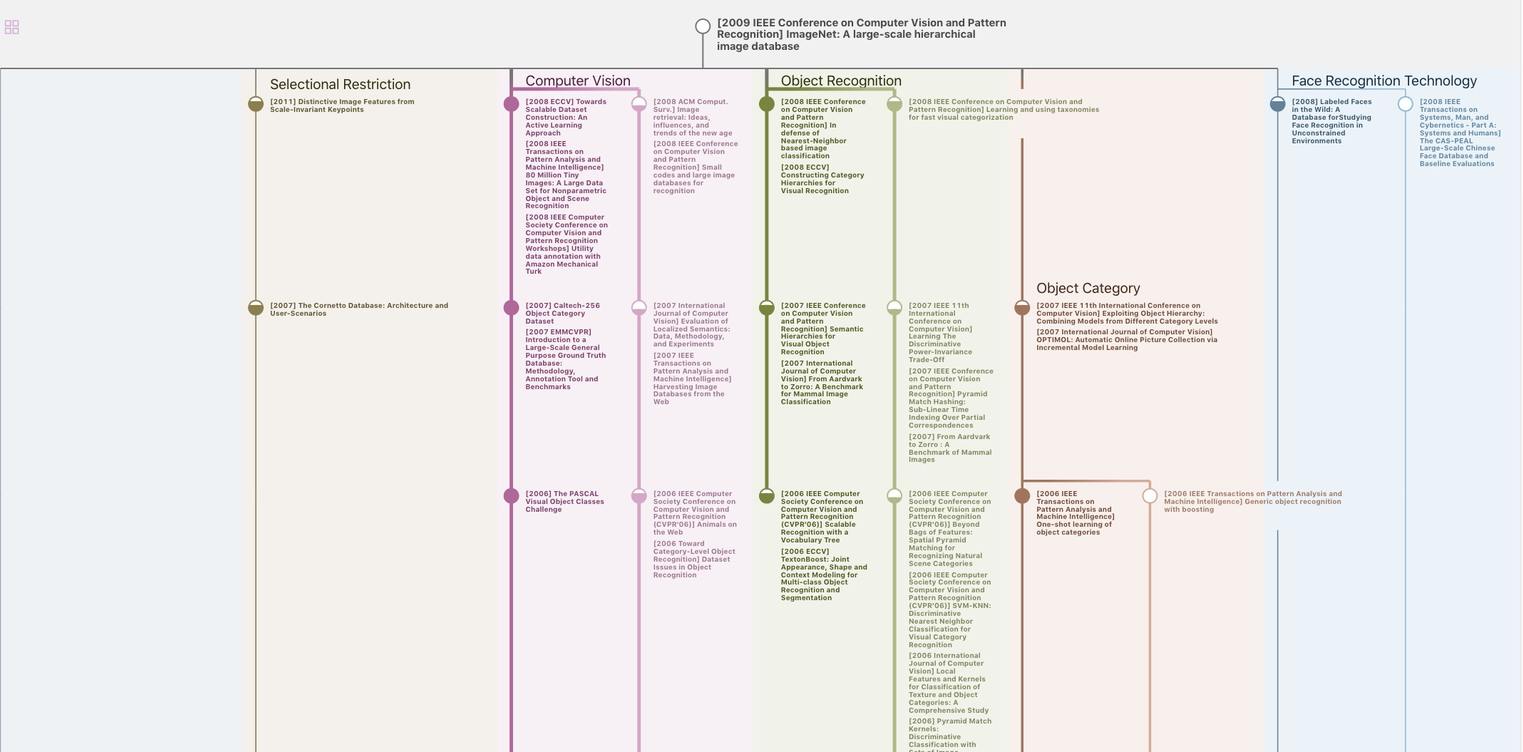
生成溯源树,研究论文发展脉络
Chat Paper
正在生成论文摘要