Rgb-D Object Detection And Semantic Segmentation For Autonomous Manipulation In Clutter
INTERNATIONAL JOURNAL OF ROBOTICS RESEARCH(2018)
摘要
Autonomous robotic manipulation in clutter is challenging. A large variety of objects must be perceived in complex scenes, where they are partially occluded and embedded among many distractors, often in restricted spaces. To tackle these challenges, we developed a deep-learning approach that combines object detection and semantic segmentation. The manipulation scenes are captured with RGB-D cameras, for which we developed a depth fusion method. Employing pretrained features makes learning from small annotated robotic datasets possible. We evaluate our approach on two challenging datasets: one captured for the Amazon Picking Challenge 2016, where our team NimbRo came in second in the Stowing and third in the Picking task; and one captured in disaster-response scenarios. The experiments show that object detection and semantic segmentation complement each other and can be combined to yield reliable object perception.
更多查看译文
关键词
Deep learning, object perception, RGB-D camera, transfer learning, object detection, semantic segmentation
AI 理解论文
溯源树
样例
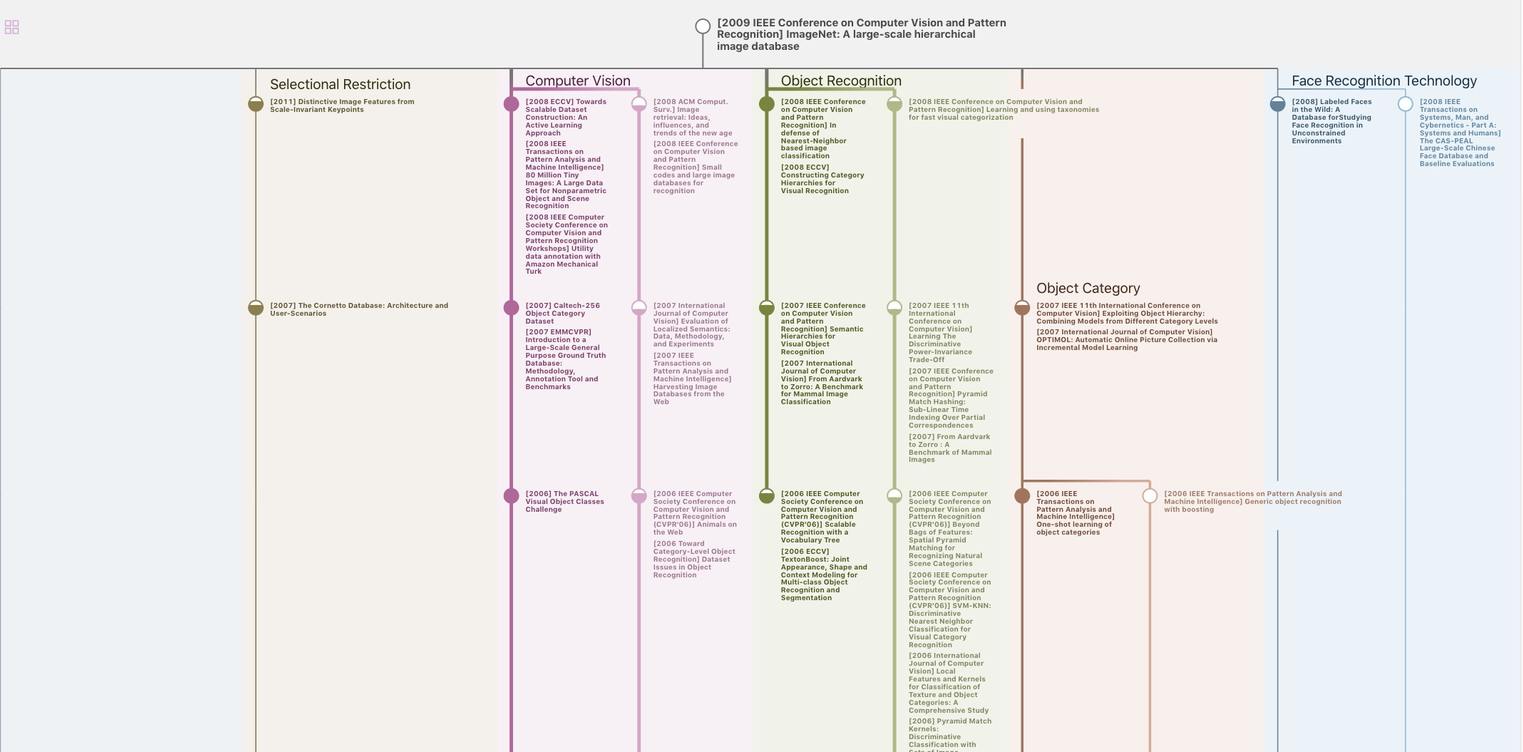
生成溯源树,研究论文发展脉络
Chat Paper
正在生成论文摘要