Generative And Discriminative Sparse Coding For Image Classification Applications
2018 IEEE WINTER CONFERENCE ON APPLICATIONS OF COMPUTER VISION (WACV 2018)(2018)
摘要
This paper presents an enhanced sparse coding method by exploiting both the generative and discriminative information in sparse representation model. Specifically, the proposed generative and discriminative sparse representation (GDSR) method integrates two new criteria, namely a discriminative criterion and a generative criterion, into the conventional sparse representation criterion. The generative criterion reveals the class conditional probability of each dictionary item by using the dictionary distribution coefficients which are derived by representing each dictionary item as a linear combination of the training samples. To further enhance the discriminative ability of the proposed method, a discriminative criterion is also applied using new localized within-class and between-class scatter matrices. Moreover, a novel GDSR based classification (GDSRc) method is proposed by utilizing both the derived sparse representation and the dictionary distribution coefficients. This hybrid method provides new insights, and leads to an effective representation and classification schema for improving the classification performance. The largest step size for learning the sparse representation is theoretically derived to address the convergence issues in the optimization procedure of the GDSR method. Extensive experimental results and analysis on several public classification datasets show the feasibility and effectiveness of the proposed method.
更多查看译文
关键词
GDSR method,image classification applications,enhanced sparse coding method,conventional sparse representation criterion,class conditional probability,dictionary distribution coefficients,between-class scatter matrices,Discriminative Sparse Coding,Generative Sparse Coding
AI 理解论文
溯源树
样例
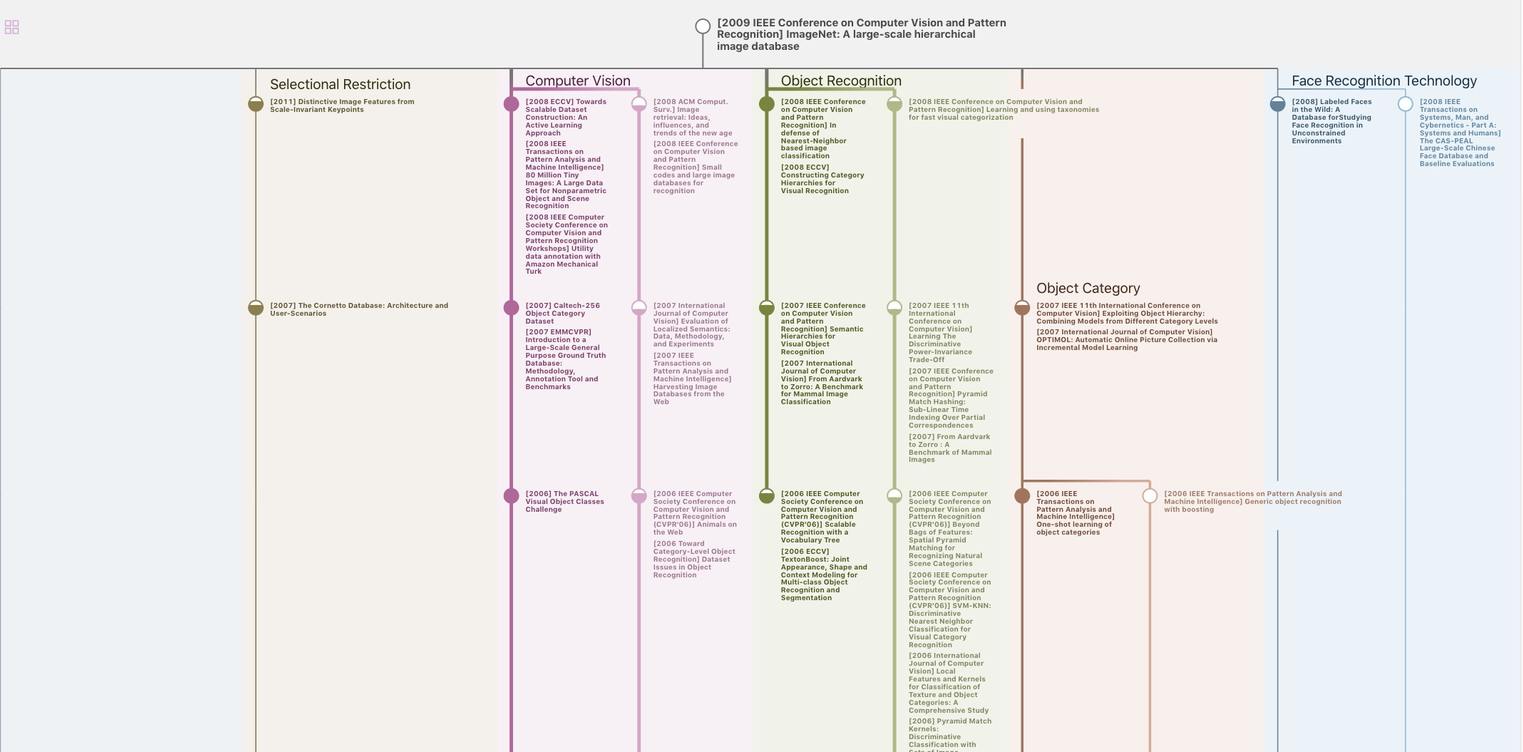
生成溯源树,研究论文发展脉络
Chat Paper
正在生成论文摘要