Learning Dual Preferences With Non-Negative Matrix Tri-Factorization For Top-N Recommender System
DATABASE SYSTEMS FOR ADVANCED APPLICATIONS, DASFAA 2018, PT I(2018)
摘要
In recommender systems, personal characteristic is possessed by not only users but also displaying products. Users have their personal rating patterns while products have different characteristics that attract users. This information can be explicitly exploited from the review text. However, most existing methods only model the review text as a topic preference of products, without considering the perspectives of users and products simultaneously. In this paper, we propose a user-product topic model to capture both user preferences and attractive characteristics of products. Different from conventional collaborative filtering in conjunction with topic models, we use non-negative matrix tri-factorization to jointly reveal the characteristic of users and products. Experiments on two real-world data sets validate the effectiveness of our method in Top-N recommendations.
更多查看译文
关键词
Top-N recommender system, Topic model, Matrix tri-factorization
AI 理解论文
溯源树
样例
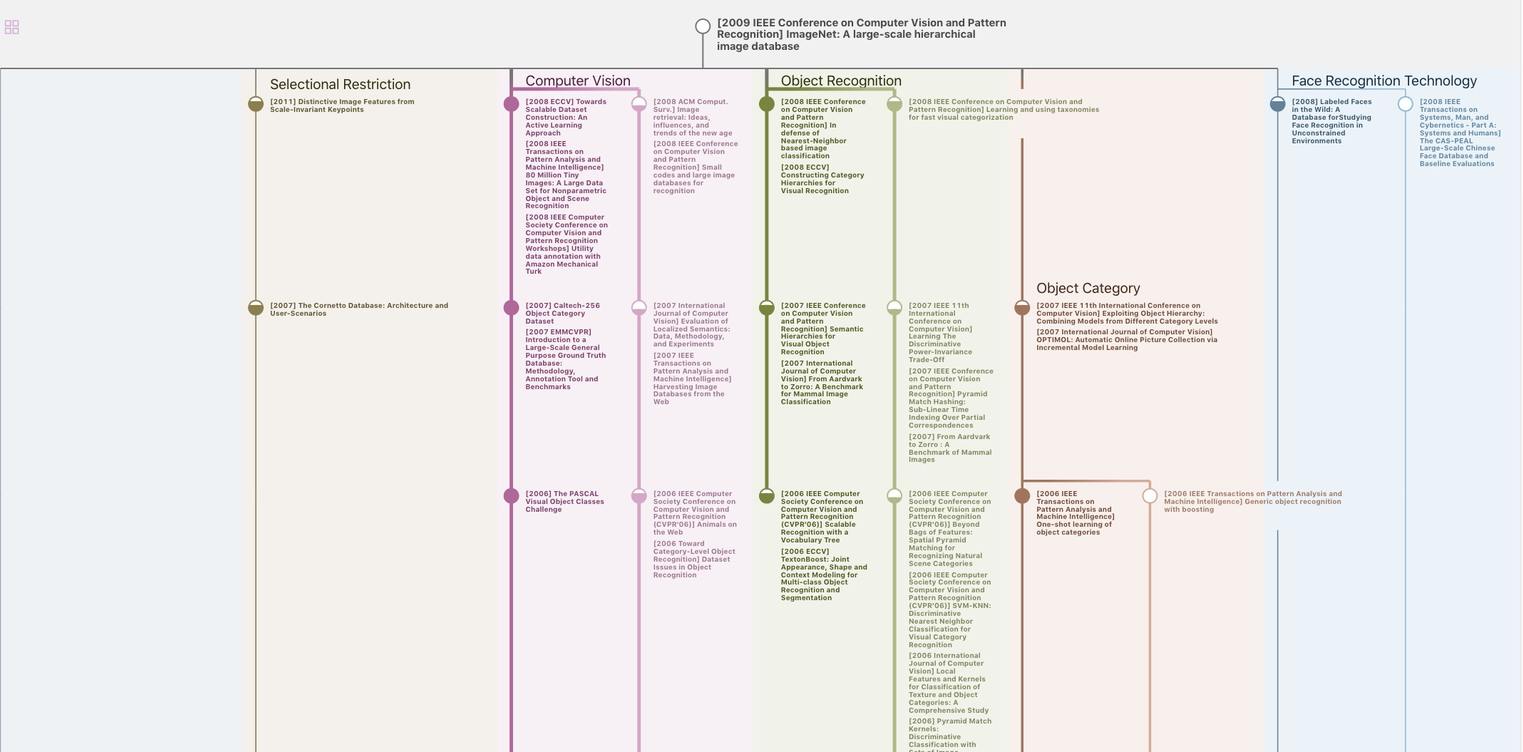
生成溯源树,研究论文发展脉络
Chat Paper
正在生成论文摘要