Undersampling Improves Hypernymy Prototypicality Learning.
LREC(2018)
摘要
This paper focuses on supervised hypernymy detection using distributional representations for unknown word pairs. Levy et al. (2015) demonstrated that supervised hypernymy detection suffers from overfitting hypernyms in training data. We show that the problem of overfitting on this task is caused by a characteristic of datasets, which stems from the inherent structure of the language resources used, hierarchical thesauri. The simple data preprocessing method proposed in this paper alleviates this problem. To be more precise, we demonstrate through experiments that the problem that hypernymy classifiers overfit hypernyms in training data comes from a skewed word frequency distribution brought by the quasi-tree structure of a thesaurus, which is a major resource of lexical semantic relation data, and propose a simple undersampling method based on word frequencies that can effectively alleviate overfitting and improve distributional prototypicality learning for unknown word pairs.
更多查看译文
关键词
learning
AI 理解论文
溯源树
样例
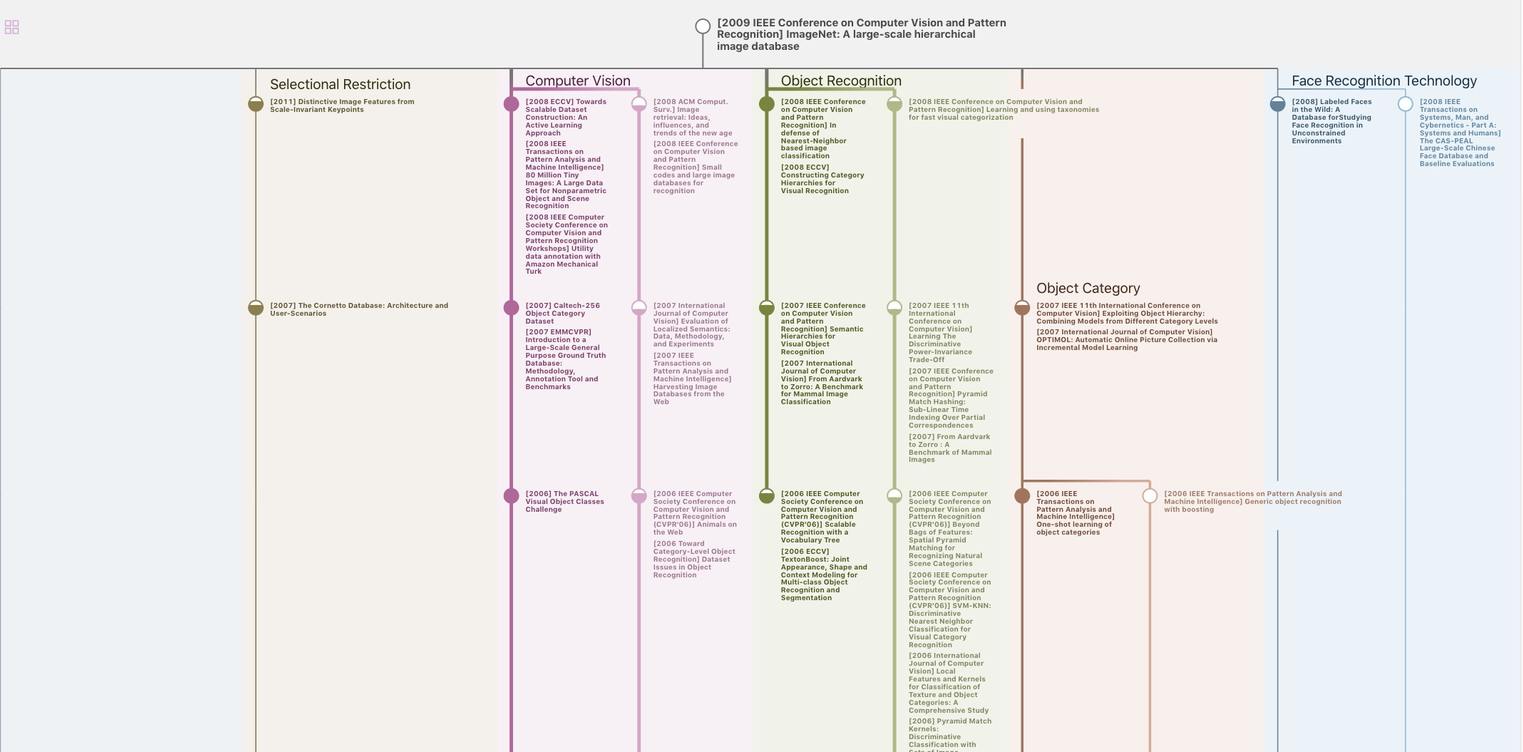
生成溯源树,研究论文发展脉络
Chat Paper
正在生成论文摘要