DUK-SVD: dynamic dictionary updating for sparse representation of a long-time remote sensing image sequence
Soft Comput.(2017)
摘要
Sparse representations of data or signals have drawn considerable attentions in the past decade. In this paper, we focus on the problem of training high-efficacy dictionaries for remote sensing images of massive long-time sequences. By extending the classical K-SVD, we propose a new dictionaries learning algorithm. Different from K-SVD, in the proposed incremental K-SVD algorithm, we selectively train a certain number of atoms when each new batch of sample data are added into the training process; current dictionary are replenished by the selected and enhanced atoms. The new atoms are initialized by information entropy. Meanwhile, we introduce an uncertainty metric to determine whether or not new atoms should be added into the current dictionary. To efficiently and sparsely represent the long-time sequence data set, we also de-correlate the dictionary based on new atoms by introducing a mutual coherence constraint into the atom updating stage. The method presented in this paper aims to adaptively and dynamically train the dictionary from big data. Two other state-of-the-art dictionary learning methods such as online dictionary learning (ODL) and recursive least squares dictionary learning algorithm (RLS-DLA) who also could train the dictionary using relatively large data, are comprehensively compared with the proposed algorithm in both sparse model and error model. In the sparse model, the reconstruction error of the DUK-SVD dictionary was smaller than ODL and RLS-DLA. In the error model, the sparsity of the DUK-SVD was higher than ODL and RLS-DLA. We can also observe that in the sparse model the proposed DUK-SVD often consume fewer computing time than ODL.
更多查看译文
关键词
Long-time sequence,Sparse representation,Dictionary learning,Remote sensing
AI 理解论文
溯源树
样例
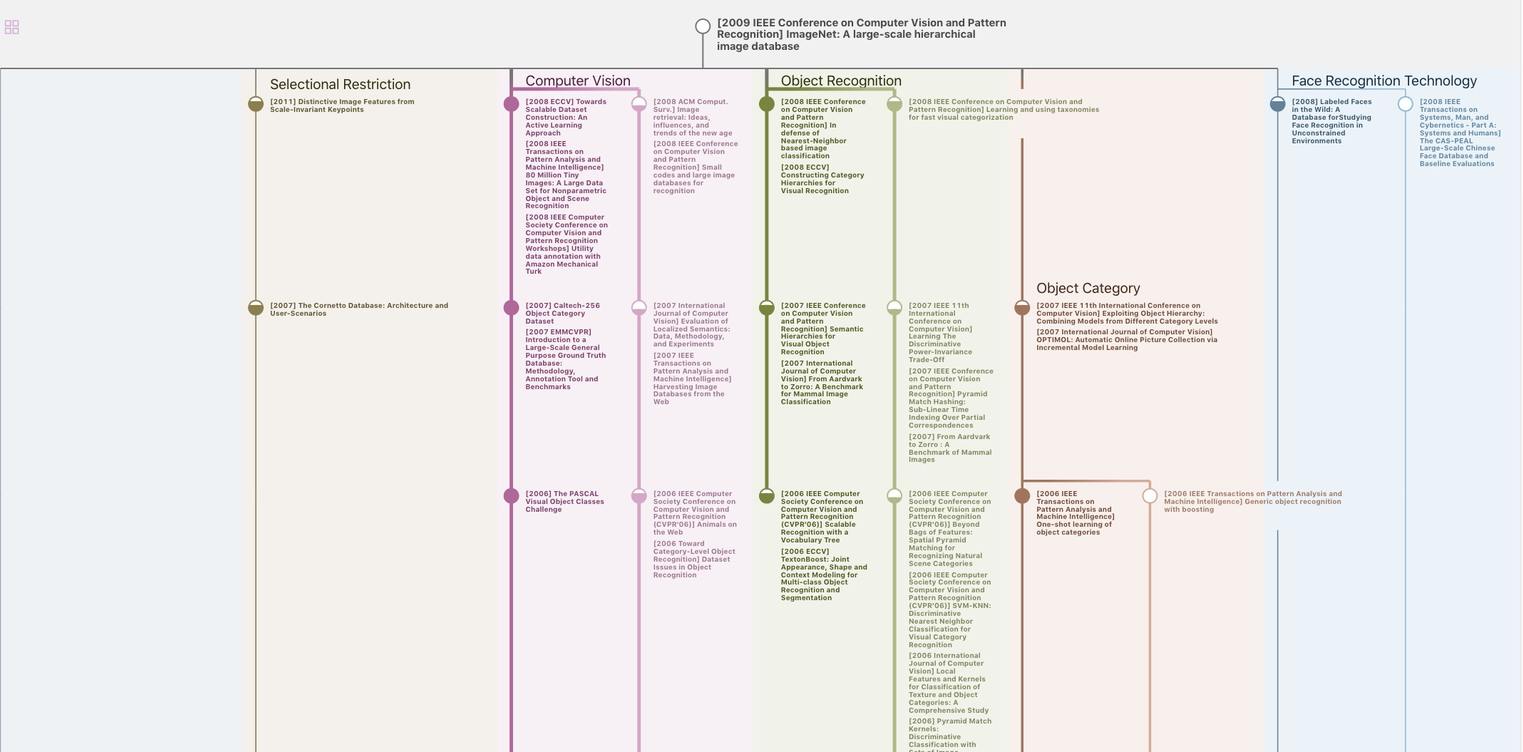
生成溯源树,研究论文发展脉络
Chat Paper
正在生成论文摘要