Dynamic ensemble selection for multi-class imbalanced datasets.
Information Sciences(2018)
摘要
Many real-world classification tasks suffer from the class imbalanced problem, in which some classes are highly underrepresented as compared to other classes. In this paper, we focus on multi-class imbalance problems which are considerably more difficult to address than two-class imbalanced problems. On this account, we develop a novel and effective procedure, called dynamic ensemble selection for multi-class imbalanced datasets (DES-MI), in which the competence of the candidate classifiers are assessed with weighted instances in the neighborhood. The proposed DES-MI consists of two key components: the generation of balanced training datasets and the selection of appropriate classifiers. To do so, we develop a preprocessing procedure to balance the training dataset which relies on random balance. To select the most appropriate classifiers in the scenario of multi-class imbalance problems, we propose a weighting mechanism to highlight the competence of classifiers that are more powerful in classifying examples in the region of underrepresented competence. We develop a thorough experimental study in order to verify the benefits of DES-MI in handling multi-class imbalanced datasets. The obtained results, supported by the proper statistical analysis, indicate that DES-MI is able to improve the classification performance for multi-class imbalanced datasets.
更多查看译文
关键词
Multi-class classification,Imbalanced datasets,Multiple classifier system,Ensemble learning,Binary decomposition,Resampling techniques
AI 理解论文
溯源树
样例
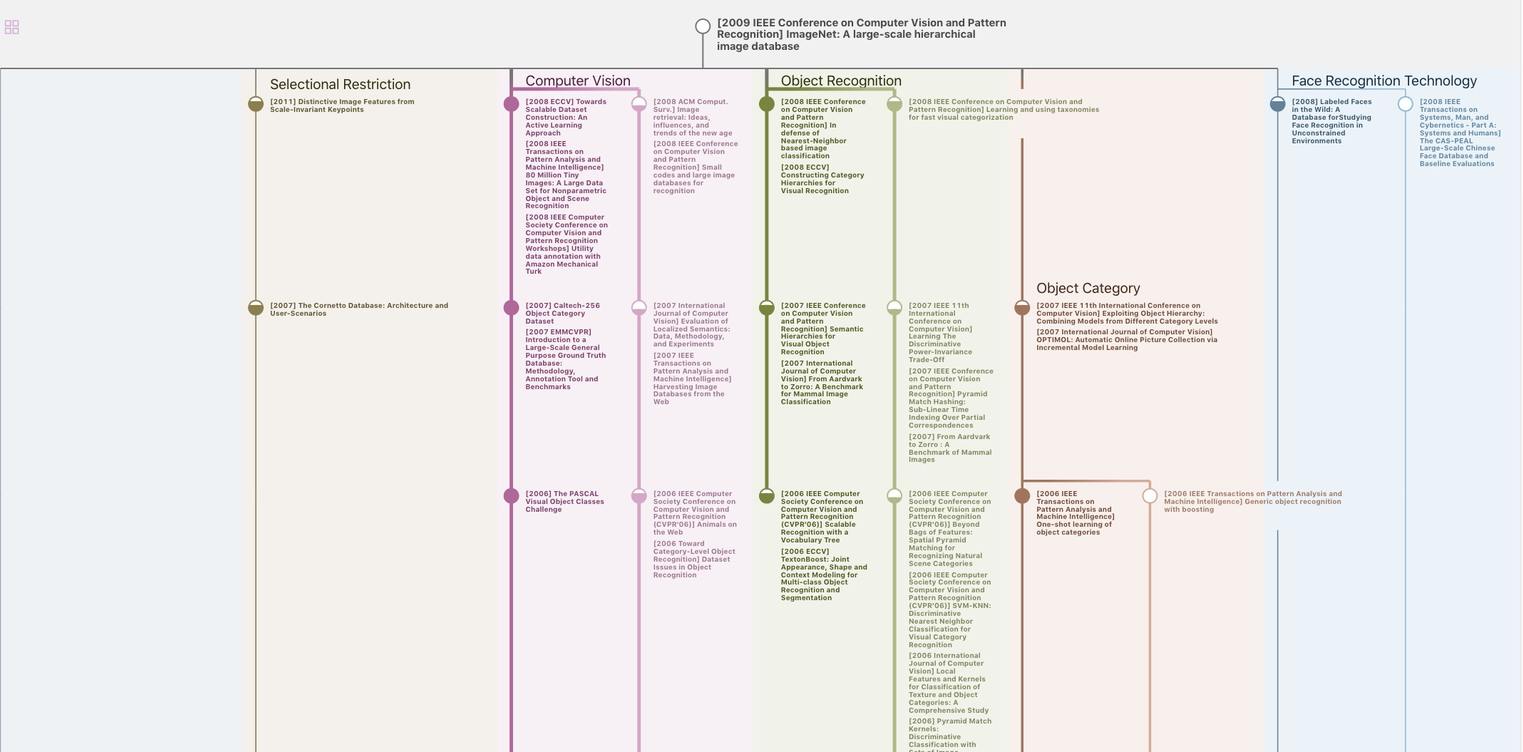
生成溯源树,研究论文发展脉络
Chat Paper
正在生成论文摘要