Classification With Truncated $\ell _{1}$ Distance Kernel.
IEEE Trans. Neural Netw. Learning Syst.(2018)
摘要
This brief proposes a truncated $ell _{1}$ distance (TL1) kernel, which results in a classifier that is nonlinear in the global region but is linear in each subregion. With this kernel, the subregion structure can be trained using all the training data and local linear classifiers can be established simultaneously. The TL1 kernel has good adaptiveness to nonlinearity and is suitable for problems which require different nonlinearities in different areas. Though the TL1 kernel is not positive semidefinite, some classical kernel learning methods are still applicable which means that the TL1 kernel can be directly used in standard toolboxes by replacing the kernel evaluation. In numerical experiments, the TL1 kernel with a pregiven parameter achieves similar or better performance than the radial basis function kernel with the parameter tuned by cross validation, implying the TL1 kernel a promising nonlinear kernel for classification tasks.
更多查看译文
关键词
Kernel,Additives,Training,Learning systems,Training data,Support vector machines,Electronic mail
AI 理解论文
溯源树
样例
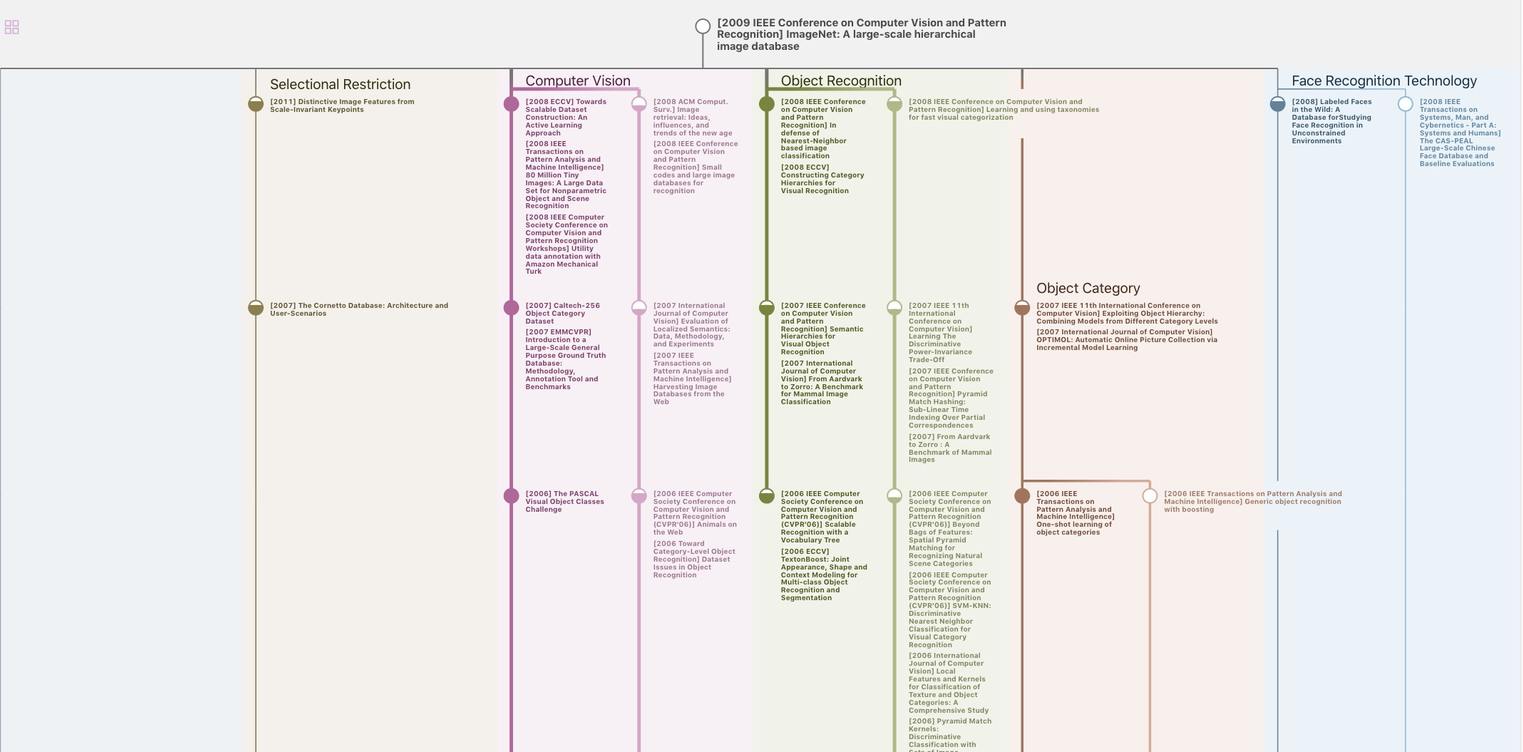
生成溯源树,研究论文发展脉络
Chat Paper
正在生成论文摘要