Classification Of Network Anomalies In Flow Level Network Traffic Using Bayesian Networks
2018 28TH INTERNATIONAL CONFERENCE ON ELECTRONICS, COMMUNICATIONS AND COMPUTERS (CONIELECOMP)(2018)
摘要
Network security is a topical issue today for everyone connected to Internet. However, malicious users try to obtain unauthorized access to network resources, affecting integrity, confidentiality, and availability. As a consequence, researchers, developers and network administrators have created many security mechanisms in order to enhance security. Among the security solutions that we can find in the market, Intrusion Detection Systems monitor inbound and outbound network activity, identifying suspicious traffic. IDS compare typical network activity with daily network activity, searching for anomalous traffic. If the IDS detects anomalous traffic, it sends an alert. In this work, we propose a Bayesian network classifier, which can detect normal or anomalous traffic. Through our Bayesian network model, it is possible to describe the cause-effect relationships that exist between the traffic features. Due to the high dimensionality of the data, and to the widespread use of the networks, we used a flow-level analysis, which saved a considerable computational load. We focus on network worms and brute force attacks, using the datasets of UNB ISCX IDS 2012 and UAN W32. Worms 2008. Results in terms of false positive and true positive rates show that the performance of the model has a high efficiency for classification of normal traffic and the set of selected attacks.
更多查看译文
关键词
Anomaly-based classifier, Argus, Bayesian networks, Intrusion detection systems, Network flows
AI 理解论文
溯源树
样例
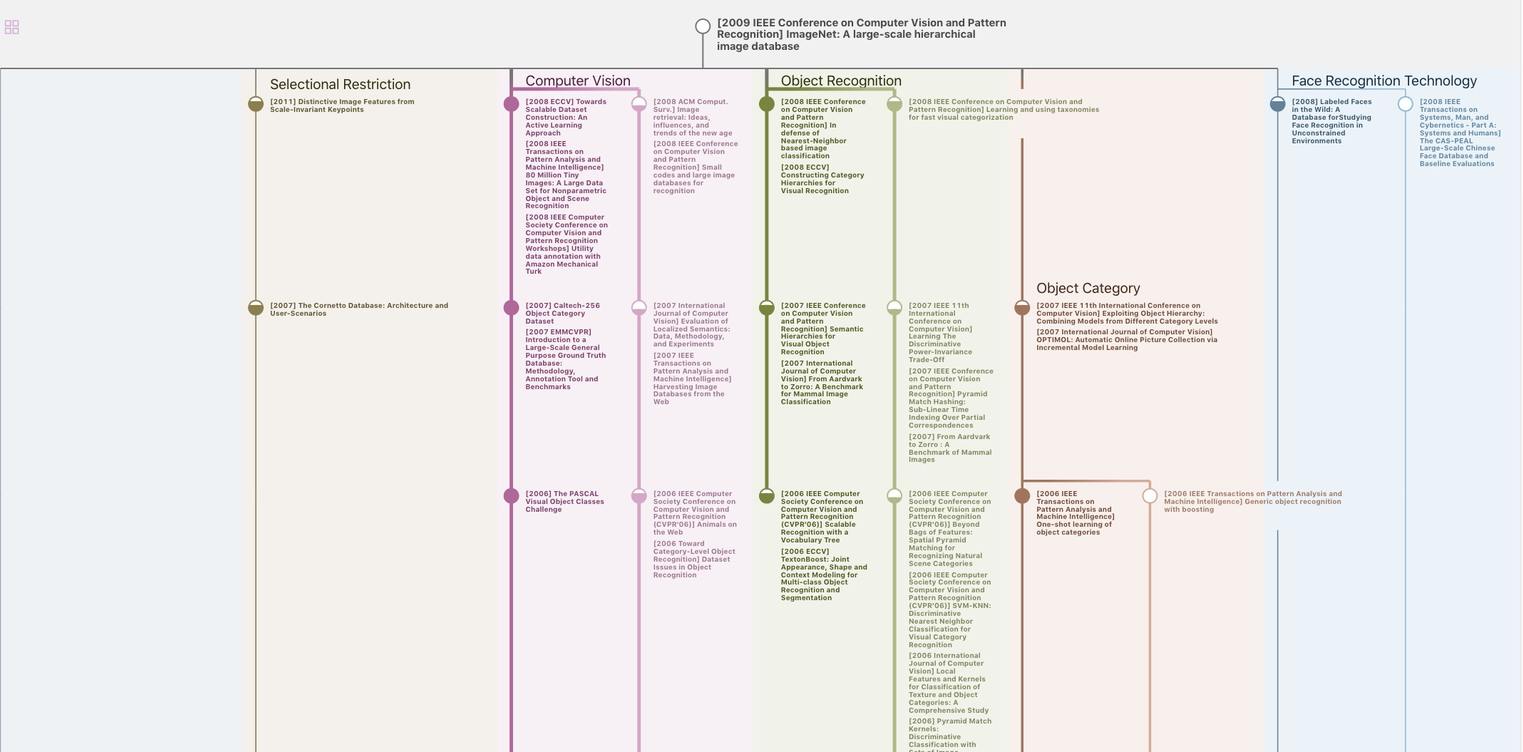
生成溯源树,研究论文发展脉络
Chat Paper
正在生成论文摘要