Fairness of Extractive Text Summarization.
WWW '18: The Web Conference 2018 Lyon France April, 2018(2018)
摘要
We propose to evaluate extractive summarization algorithms from a completely new perspective. Considering that an extractive summarization algorithm selects a subset of the textual units in the input data for inclusion in the summary, we investigate whether this selection is fair. We use several summarization algorithms over datasets that have a sensitive attribute (e.g., gender, political leaning) associated with the textual units, and find that the generated summaries often have very different distributions of the said attribute. Specifically, some classes of the textual units are under-represented in the summaries according to the fairness notion of adverse impact. To our knowledge, this is the first work on fairness of summarization, and is likely to open up interesting research problems.
更多查看译文
关键词
Extractive summarization, fairness, adverse impact
AI 理解论文
溯源树
样例
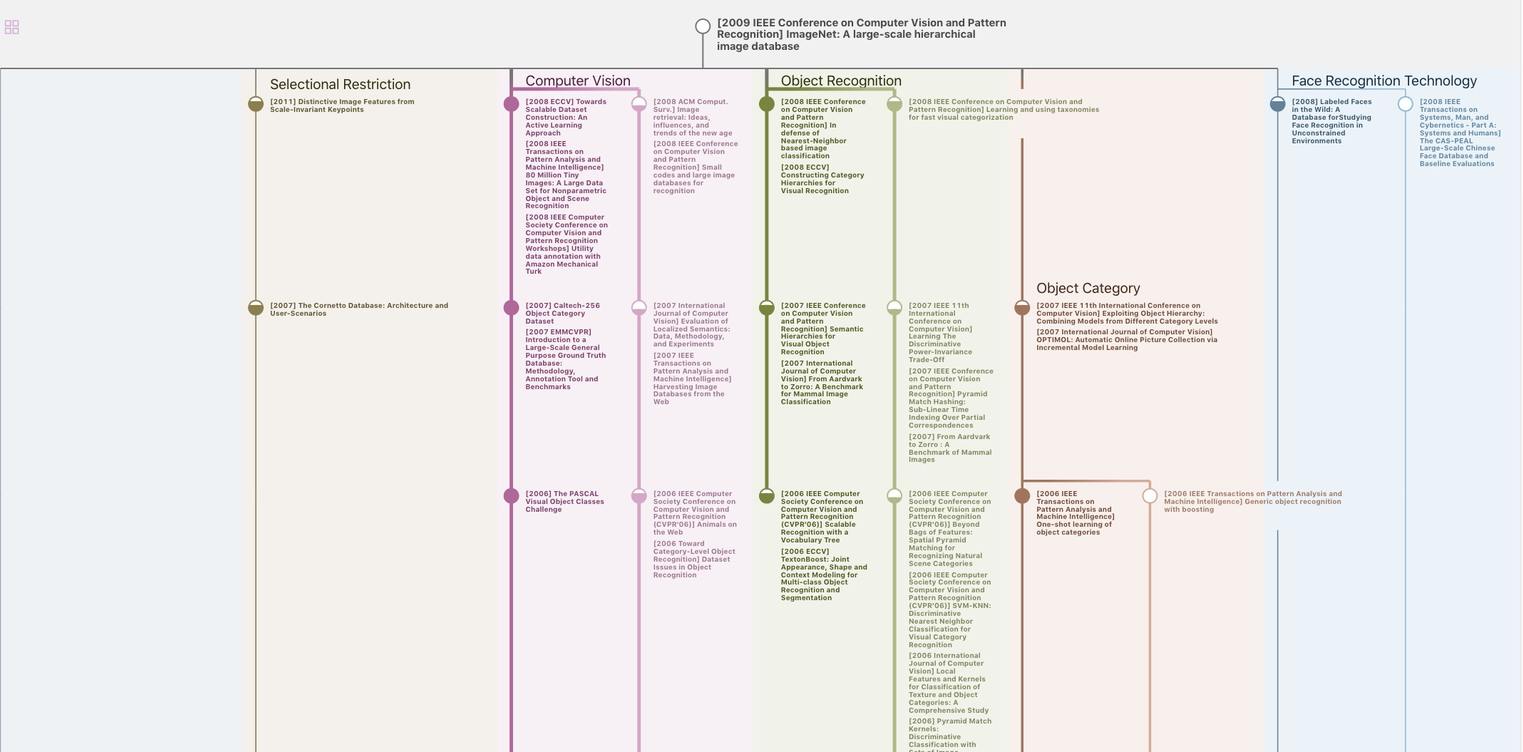
生成溯源树,研究论文发展脉络
Chat Paper
正在生成论文摘要