Few-Shot Generative Modelling With Generative Matching Networks
INTERNATIONAL CONFERENCE ON ARTIFICIAL INTELLIGENCE AND STATISTICS, VOL 84(2018)
摘要
Despite recent advances, the remaining bottlenecks in deep generative models are necessity of extensive training and difficulties with generalization from small number of training examples. We develop a new generative model called Generative Matching Network which is inspired by the recently proposed matching networks for one-shot learning in discriminative tasks. By conditioning on the additional input dataset, our model can instantly learn new concepts that were not available in the training data but conform to a similar generative process. The proposed framework does not explicitly restrict diversity of the conditioning data and also does not require an extensive inference procedure for training or adaptation. Our experiments on the Omniglot dataset demonstrate that Generative Matching Networks significantly improve predictive performance on the fly as more additional data is available and outperform existing state of the art conditional generative models.
更多查看译文
关键词
modelling,networks,few-shot
AI 理解论文
溯源树
样例
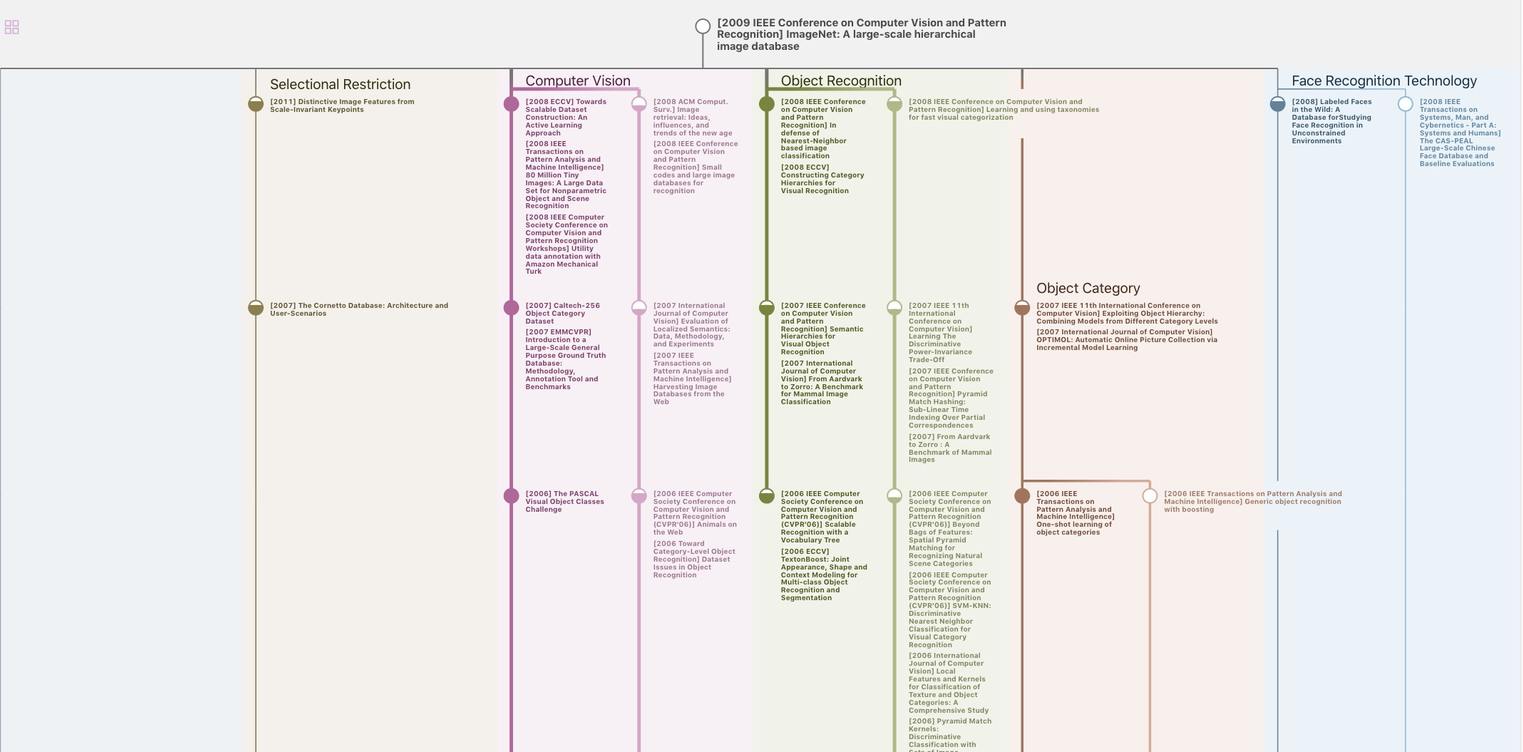
生成溯源树,研究论文发展脉络
Chat Paper
正在生成论文摘要