A Scalable Purchase Intention Prediction System Using Extreme Gradient Boosting Machines With Browsing Content Entropy
2018 IEEE INTERNATIONAL CONFERENCE ON CONSUMER ELECTRONICS (ICCE)(2018)
摘要
Nowadays, a prosperity of electronic commerce (Ecommerce) not only gives more convenience to consumers but brings more new opportunities in online advertising and marketing. Online advertisers can understand more about consumer preferences based on their daily online shopping and browsing behaviors. The development of big data and cloud computing techniques further empower advertisers and marketers to have a data-driven and consumer-specific preference recommendation based on the online browsing histories. In this research, a decision support system is proposed to predict a consumer purchase intention during browsing sessions. The proposed decision support system categorizes online browsing activities into purchase-oriented and general sessions using extreme boosting machines. With the browsing content entropy features, the proposed method achieves 41.81% recall and 34.35% F-1 score. It further shows its strong predictive capability compared to other benchmark algorithms including logistic regression and traditional ensemble models. The proposed method can be implemented in real-time bidding algorithms for online advertising strategies. Ad deliveries on browsing session with potential purchase intention not only improve the effectiveness of advertisements but significantly increase last-touch attributions for campaign performance.
更多查看译文
关键词
purchase intention prediction, data mining, decision trees, extreme gradient boosting machines
AI 理解论文
溯源树
样例
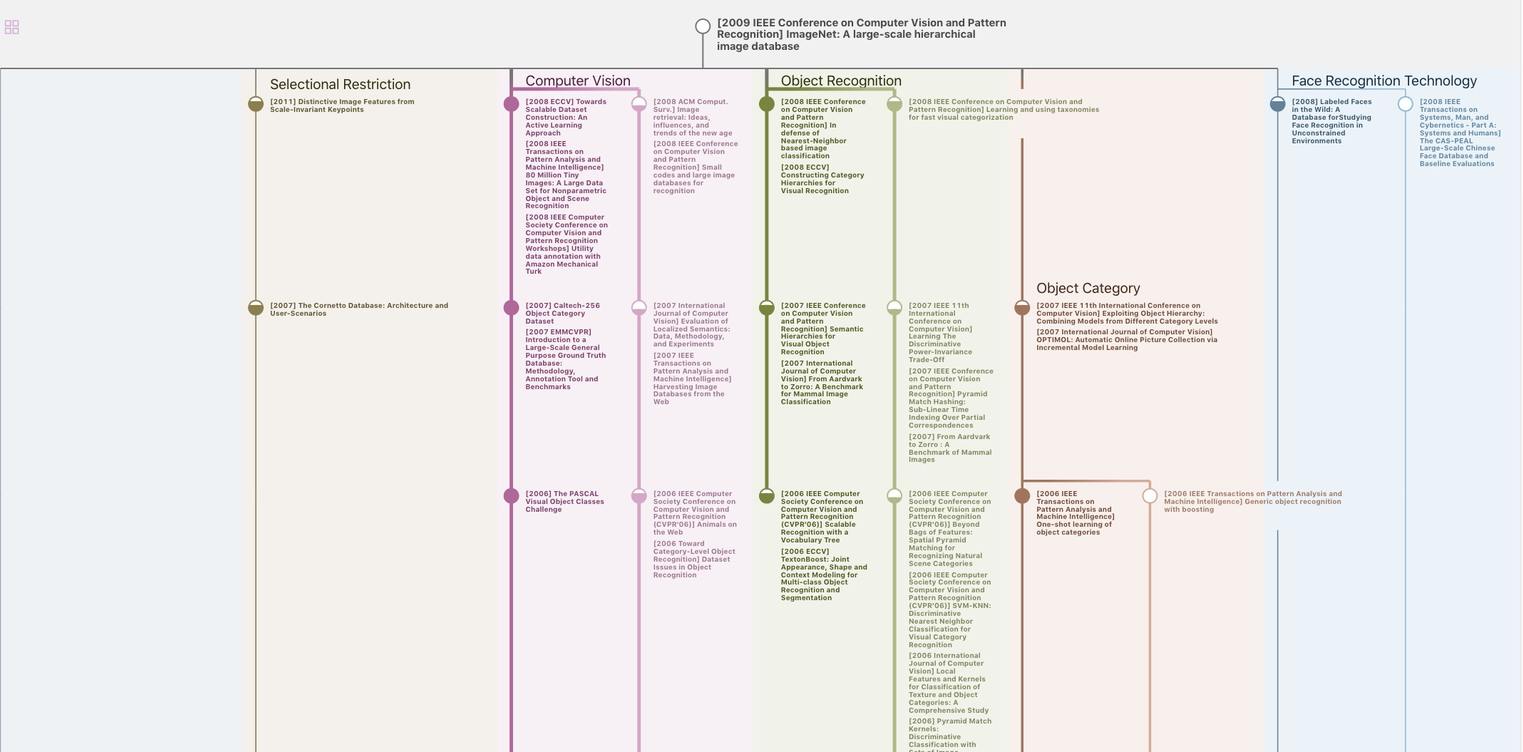
生成溯源树,研究论文发展脉络
Chat Paper
正在生成论文摘要