Domain-Specific Approximation For Object Detection
IEEE MICRO(2018)
摘要
There is growing interest in object detection in advanced driver assistance systems and autonomous robots and vehicles. To enable such innovative systems, we need faster object detection. In this article, we investigate the trade-off between accuracy and speed with domain-specific approximations (DSAs) for two state-of-the-art deep learning-based object detection meta-architectures. We study the effectiveness of applying approximation both statically and dynamically to understand their potential and applicability. By conducting experiments on the ImageNet VID dataset, we show that DSA has great potential to improve the speed of the system without deteriorating the accuracy of object detectors. To this end, we present our insights on harvesting DSA and devise a proof-of-concept runtime, AutoFocus, that exploits dynamic DSA.
更多查看译文
关键词
Object detection,Detectors,Vehicle dynamics,Feature extraction,Motorcycles,Intelligent vehicles,Autonomous automobiles
AI 理解论文
溯源树
样例
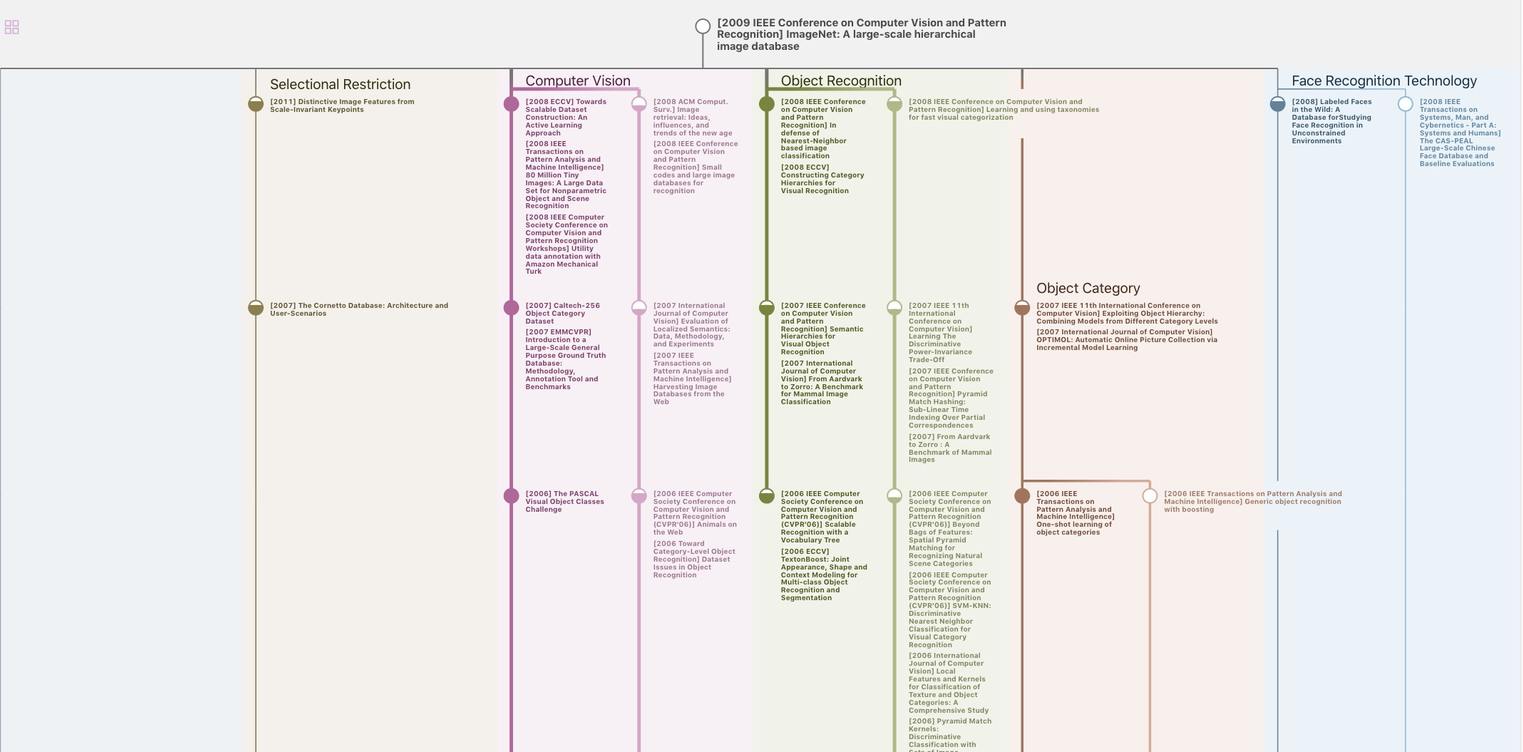
生成溯源树,研究论文发展脉络
Chat Paper
正在生成论文摘要