PrivPfC: differentially private data publication for classification
VLDB J.(2018)
摘要
In this paper, we tackle the problem of constructing a differentially private synopsis for the classification analysis. Several state-of-the-art methods follow the structure of existing classification algorithms and are all iterative, which is suboptimal due to the locally optimal choices and division of the privacy budget among many sequentially composed steps. We propose PrivPfC, a new differentially private method for releasing data for classification. The key idea underlying PrivPfC is to privately select, in a single step, a grid, which partitions the data domain into a number of cells. This selection is done by using the exponential mechanism with a novel quality function, which maximizes the expected number of correctly classified records by a histogram classifier. PrivPfC supports both the binary classification and the multiclass classification. Through extensive experiments on real datasets, we demonstrate PrivPfC ’s superiority over the state-of-the-art methods.
更多查看译文
关键词
Differential privacy,Classification,Privacy preserving data publishing
AI 理解论文
溯源树
样例
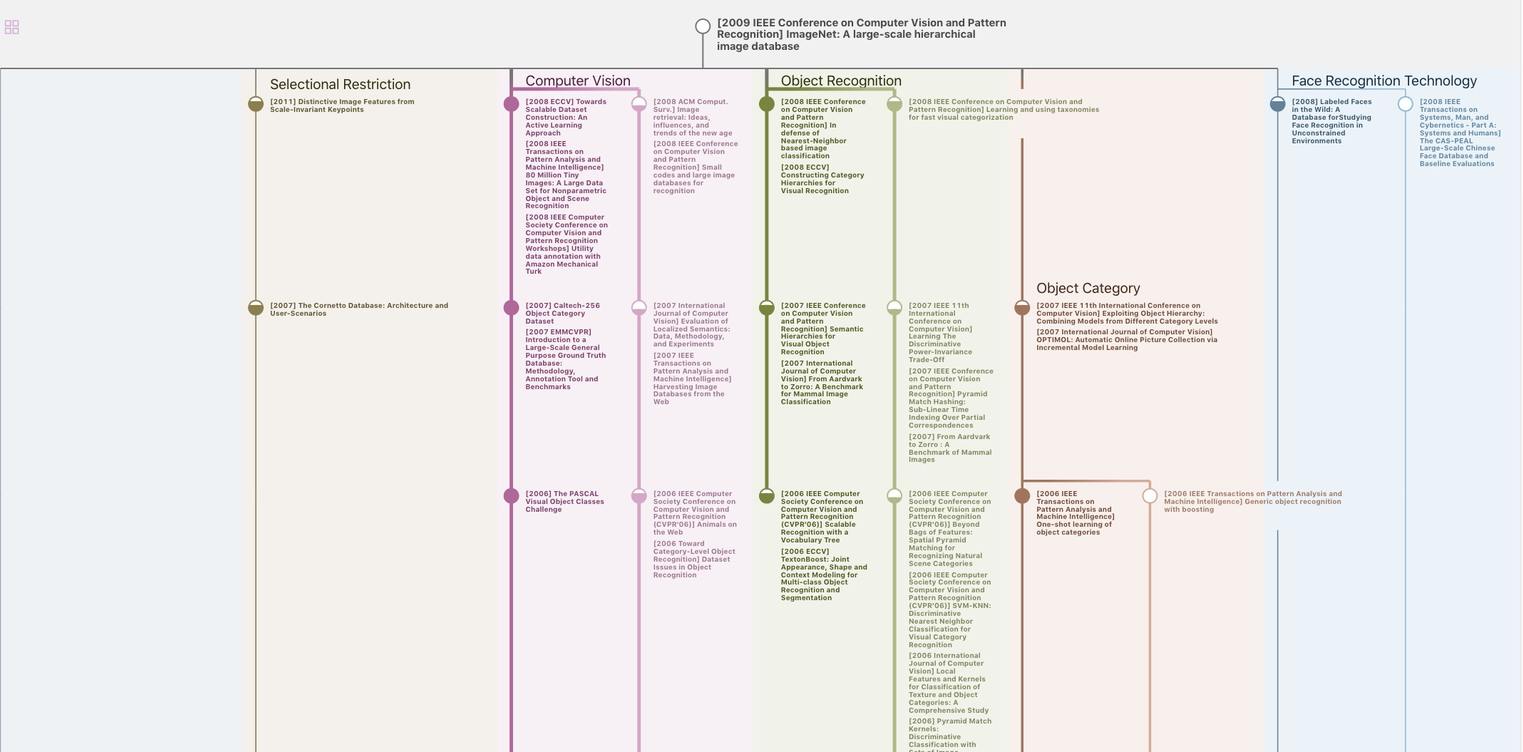
生成溯源树,研究论文发展脉络
Chat Paper
正在生成论文摘要