Automatic Ship Detection of Remote Sensing Images from Google Earth in Complex Scenes Based on Multi-Scale Rotation Dense Feature Pyramid Networks.
Remote Sensing(2018)
摘要
Ship detection has been playing a significant role in the field of remote sensing for a long time, but it is still full of challenges. The main limitations of traditional ship detection methods usually lie in the complexity of application scenarios, the difficulty of intensive object detection, and the redundancy of the detection region. In order to solve these problems above, we propose a framework called Rotation Dense Feature Pyramid Networks (R-DFPN) which can effectively detect ships in different scenes including ocean and port. Specifically, we put forward the Dense Feature Pyramid Network (DFPN), which is aimed at solving problems resulting from the narrow width of the ship. Compared with previous multiscale detectors such as Feature Pyramid Network (FPN), DFPN builds high-level semantic feature-maps for all scales by means of dense connections, through which feature propagation is enhanced and feature reuse is encouraged. Additionally, in the case of ship rotation and dense arrangement, we design a rotation anchor strategy to predict the minimum circumscribed rectangle of the object so as to reduce the redundant detection region and improve the recall. Furthermore, we also propose multiscale region of interest (ROI) Align for the purpose of maintaining the completeness of the semantic and spatial information. Experiments based on remote sensing images from Google Earth for ship detection show that our detection method based on R-DFPN representation has state-of-the-art performance.
更多查看译文
关键词
remote sensing,convolution neural network,ship detection,high-level semantic,rotation region,multiscale detection networks
AI 理解论文
溯源树
样例
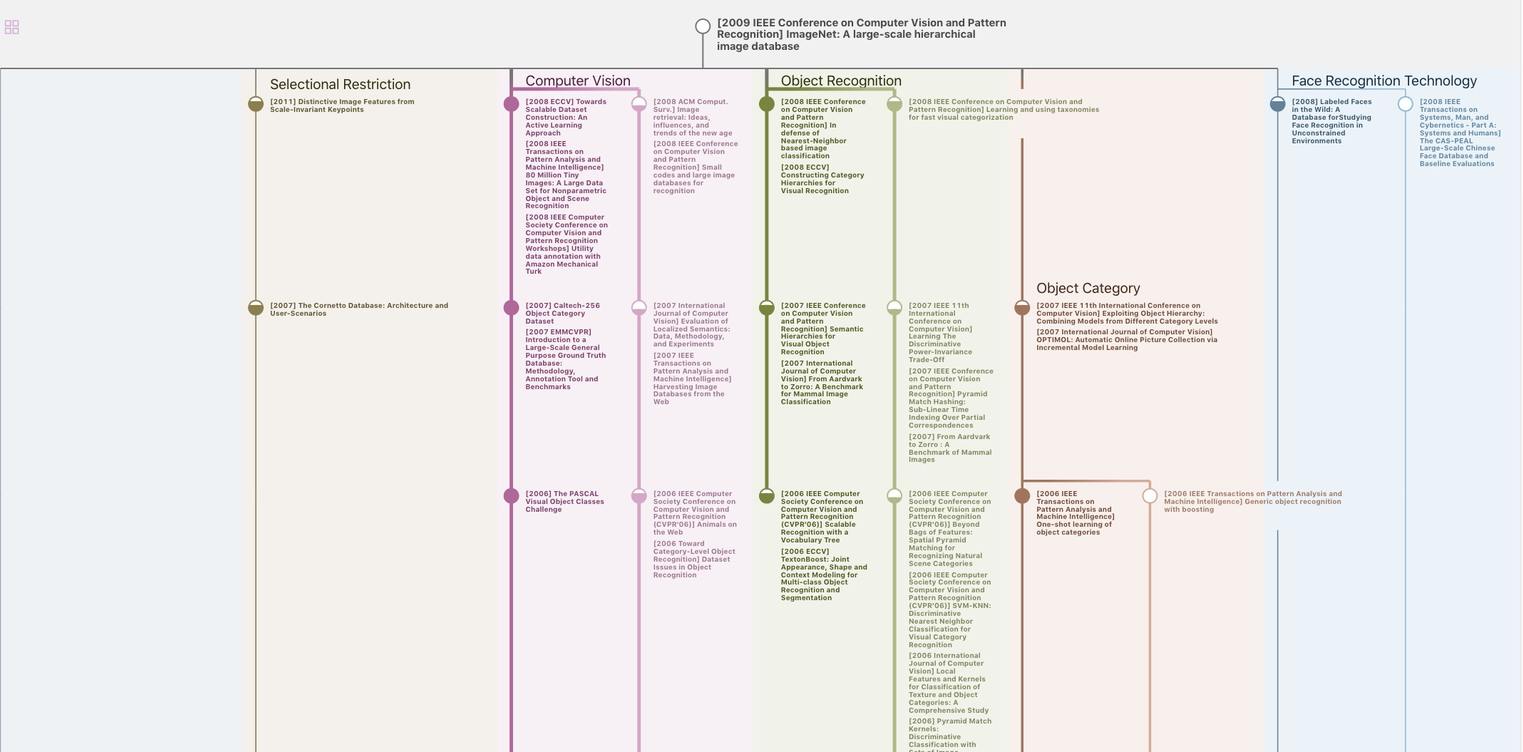
生成溯源树,研究论文发展脉络
Chat Paper
正在生成论文摘要