Bayesian Approach To Multilayer Stochastic Blockmodel And Network Changepoint Detection
NETWORK SCIENCE(2017)
摘要
Network scholars commonly encounter multiple networks, each of which is possibly governed by distinct generation rules while sharing a node group structure. Although the stochastic blockmodeling-detecting such latent group structures with group-specific connection profiles- has been a major topic of recent research, the focus has been given to the assortative group discovery of a single network. Despite its universality, concepts, and techniques for simultaneous characterization of node traits of multilayer networks, constructed by stacking multiple networks into layers, have been limited. Here, we propose a Bayesian multilayer stochastic blockmodeling framework that uncovers layer-common node traits and factors associated with layer-specific network generating functions. Without assuming a priori layerspecific generation rules, our fully Bayesian treatment allows probabilistic inference of latent traits. We extend the approach to detect changes in block structures embedded in temporal layers of network time series. We demonstrate the method using synthetic multilayer networks with assortative, disassortative, core-periphery, and overlapping community structures. Finally, we apply the method to empirical social network datasets, and find that it detects significant latent traits and structural changepoints. In particular, we uncover endogenous historical regimes associated with distinct constellations of states in United States Senate roll call vote similarity patterns.
更多查看译文
关键词
multilayer network, stochastic blockmodel, network changepoint detection, tensor decomposition, network time series, hidden Markov model
AI 理解论文
溯源树
样例
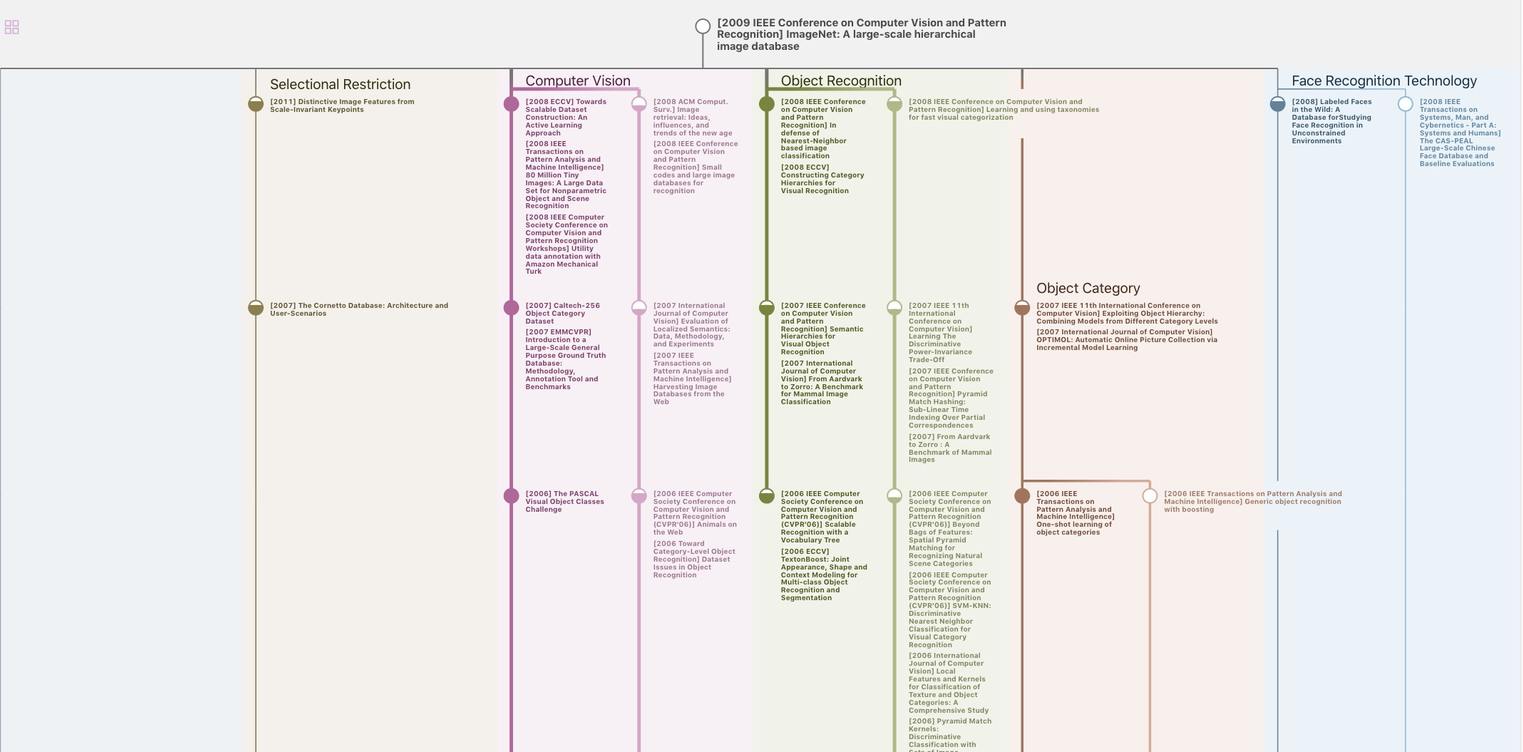
生成溯源树,研究论文发展脉络
Chat Paper
正在生成论文摘要