A 65nm 1mb Nonvolatile Computing-In-Memory Reram Macro With Sub-16ns Multiply-And-Accumulate For Binary Dnn Ai Edge Processors
2018 IEEE INTERNATIONAL SOLID-STATE CIRCUITS CONFERENCE - (ISSCC)(2018)
摘要
Many artificial intelligence (AI) edge devices use nonvolatile memory (NVM) to store the weights for the neural network (trained off-line on an AI server), and require low-energy and fast I/O accesses. The deep neural networks (DNN) used by AI processors [1,2] commonly require p-layers of a convolutional neural network (CNN) and q-layers of a fully-connected network (FCN). Current DNN processors that use a conventional (von-Neumann) memory structure are limited by high access latencies, I/O energy consumption, and hardware costs. Large working data sets result in heavy accesses across the memory hierarchy, moreover large amounts of intermediate data are also generated due to the large number of multiply-and-accumulate (MAC) operations for both CNN and FCN. Even when binary-based DNN [3] are used, the required CNN and FCN operations result in a major memory I/O bottleneck for AI edge devices.
更多查看译文
关键词
binary DNN AI edge processors,artificial intelligence edge devices,nonvolatile memory,neural network,AI server,deep neural networks,fully-connected network,FCN,conventional memory structure,high access latencies,energy consumption,memory hierarchy,multiply-and-accumulate operations,AI edge devices,nonvolatile computing-in-memory ReRAM macro,fast I/O accesses,von-Neumann memory structure,I/O energy consumption,hardware cost,size 65.0 nm,storage capacity 1 Mbit
AI 理解论文
溯源树
样例
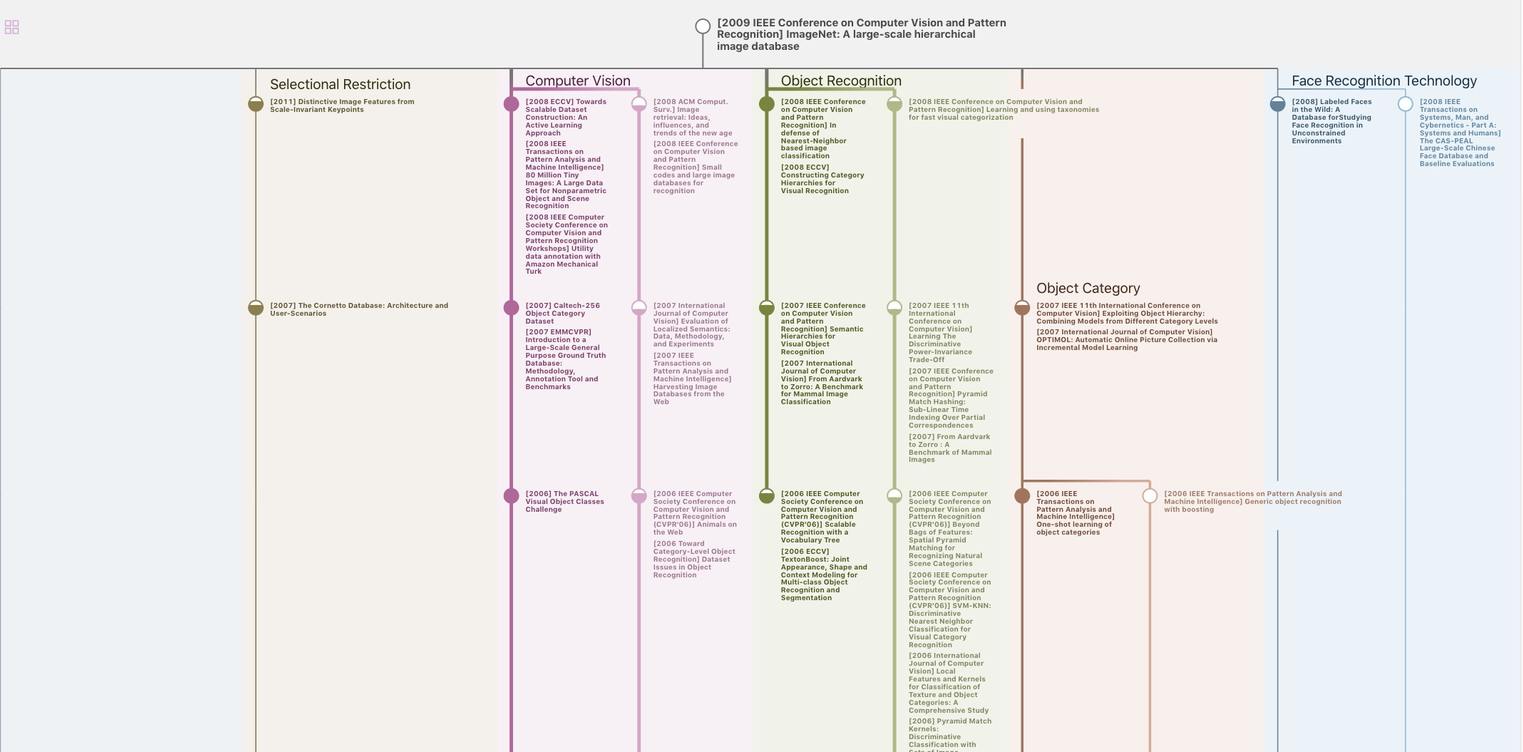
生成溯源树,研究论文发展脉络
Chat Paper
正在生成论文摘要