Bounding the difference between RankRC and RankSVM and application to multi-level rare class kernel ranking
Data Min. Knowl. Discov.(2017)
摘要
Rapid explosion in data accumulation has yielded large scale data mining problems, many of which have intrinsically unbalanced or rare class distributions. Standard classification algorithms, which focus on overall classification accuracy, often perform poorly in these cases. Recently, Tayal et al. (IEEE Trans Knowl Data Eng 27(12):3347–3359, 2015 ) proposed a kernel method called RankRC for large-scale unbalanced learning. RankRC uses a ranking loss to overcome biases inherent in standard classification based loss functions, while achieving computational efficiency by enforcing a rare class hypothesis representation. In this paper we establish a theoretical bound for RankRC by establishing equivalence between instantiating a hypothesis using a subset of training points and instantiating a hypothesis using the full training set but with the feature mapping equal to the orthogonal projection of the original mapping. This bound suggests that it is optimal to select points from the rare class first when choosing the subset of data points for a hypothesis representation. In addition, we show that for an arbitrary loss function, the Nyström kernel matrix approximation is equivalent to instantiating a hypothesis using a subset of data points. Consequently, a theoretical bound for the Nyström kernel SVM can be established based on the perturbation analysis of the orthogonal projection in the feature mapping. This generally leads to a tighter bound in comparison to perturbation analysis based on kernel matrix approximation. To further illustrate computational effectiveness of RankRC, we apply a multi-level rare class kernel ranking method to the Heritage Health Provider Network’s health prize competition problem and compare the performance of RankRC to other existing methods.
更多查看译文
关键词
Rare class,Receiver operator characteristic,AUC,Ranking loss,Scalable computing,Nonlinear kernel,Ranking svm
AI 理解论文
溯源树
样例
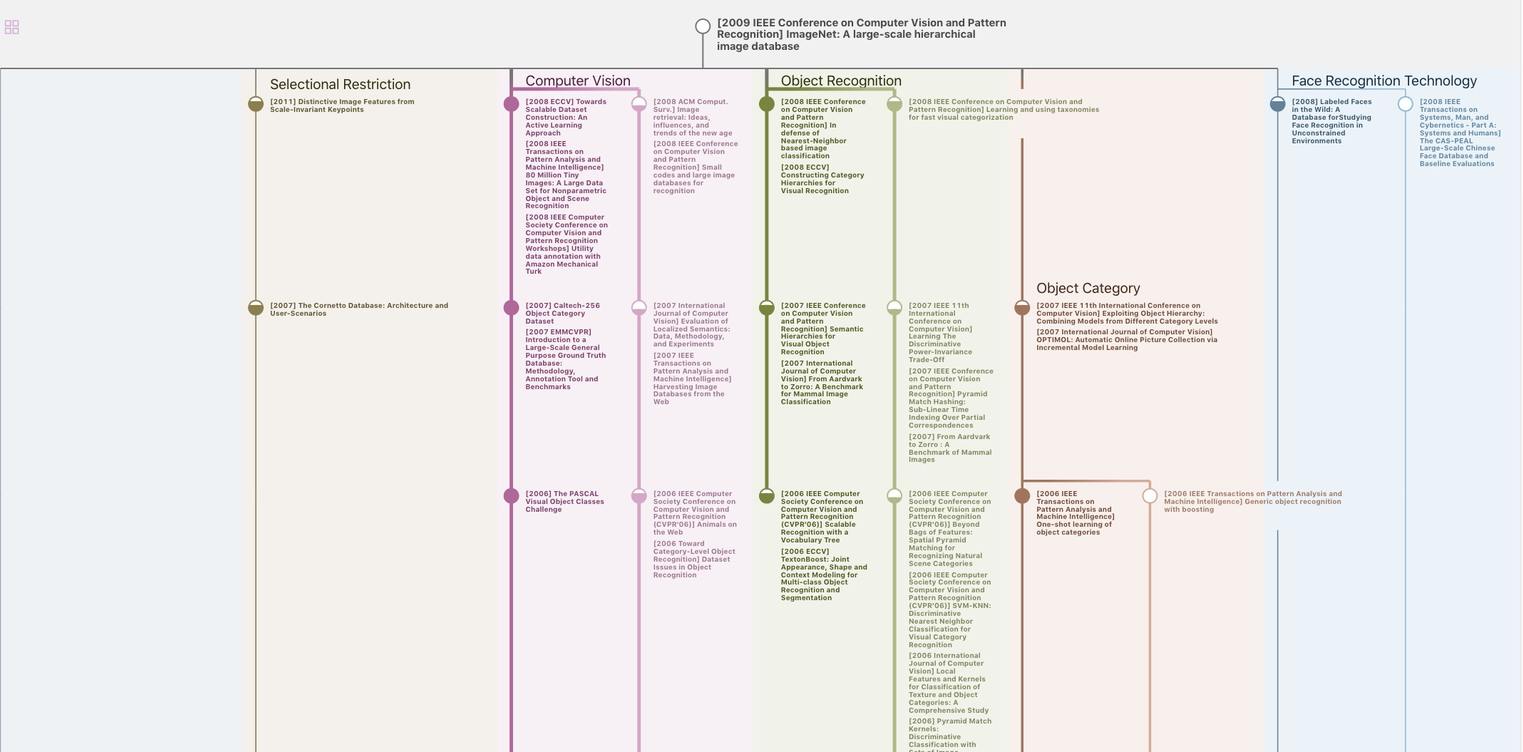
生成溯源树,研究论文发展脉络
Chat Paper
正在生成论文摘要