A Parallel Convolutional Neural Network Architecture For Stereo Vision Estimation
2017 24TH IEEE INTERNATIONAL CONFERENCE ON IMAGE PROCESSING (ICIP)(2017)
摘要
Extracting depth information from the stereo image pair is a commonly used method in 3-D computer vision. For robotics and unmanned vehicle applications that require real-time performance, speed is often more important than accuracy. In recent years, Convolutional Neural Networks (CNNs) have shown great success in many computer vision applications including classification, segmentation, object detection, edge detection, and stereo vision estimation. Existing network architectures for stereo vision estimation predict very little information during the forward pass and are only able to calculate the disparity for one pixel at a time. In this paper, we propose a parallel architecture to speed up disparity map computation by simultaneously processing all pixels on one horizontal line. We train and test our network on five Middlebury datasets. Our parallel architecture achieves at least a 10x speedup compared to existing networks. Its accuracy is also very competitive.
更多查看译文
关键词
Stereo Vision, CNN, Deep Learning, Parallel Architecture
AI 理解论文
溯源树
样例
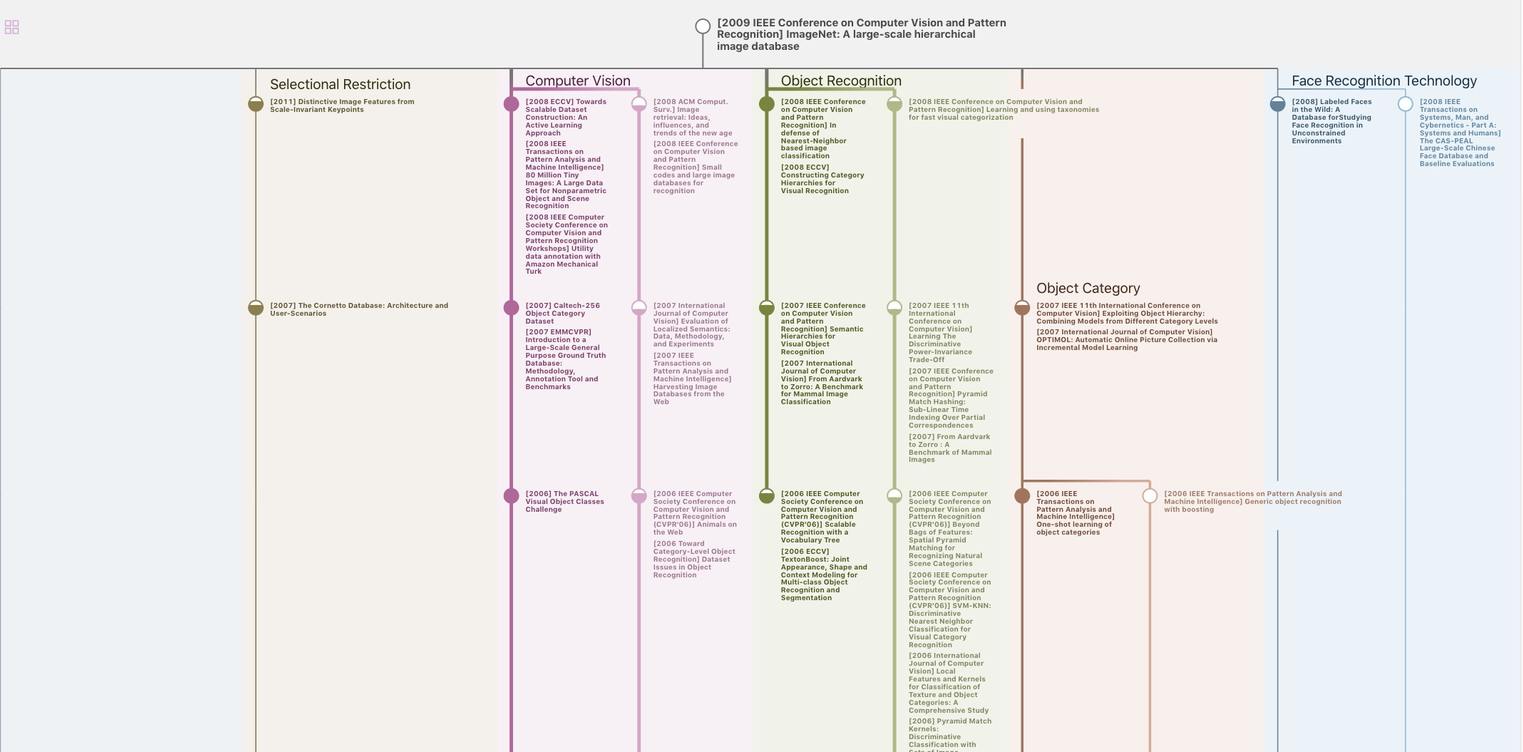
生成溯源树,研究论文发展脉络
Chat Paper
正在生成论文摘要