A level-map approach to transform coefficient coding
2017 24TH IEEE INTERNATIONAL CONFERENCE ON IMAGE PROCESSING (ICIP)(2017)
摘要
Transform coding is widely used in the video and image codec to largely remove the spatial correlation. The magnitude of transform coefficient is weakly correlated to a number of factors, including its frequency band, the neighboring coefficient magnitudes, luma/chroma planes, etc. To exploit such correlations for efficient entropy coding, one would build a probability model conditioned on the available contexts. However, the interaction of these factors creates a high dimensional space, a direct use of which would easily fall into the over-fitting problem. How to construct a compact context set which effectively captures the underlying correlations remains a major challenge in video and image compression. Prior research work primarily relies on bucketizing the previously coded coefficients into a small number of categories as the context model for next coefficient. Certain information loss is inevitable due to the classification process. To fully exploit the available context in a limited model space, a level map approach is proposed in this work. It decomposes the coding of coefficient magnitudes into consecutive runs of binary map coding, each corresponds to whether a coefficient is equal to or greater than the given level. Under the Markov assumption across the levels, nearly all the reference symbols available to each level map can be approximated as binary random variables. It hence allows the context model to account for all the surrounding coefficients information provided by the lower level maps, while retaining a reasonably compact size. Experimental evidence demonstrates that the proposed coding scheme provides considerable compression performance gains consistently over a large test settings.
更多查看译文
关键词
Transform coding, probability context modeling, level map, adaptive coefficient scan
AI 理解论文
溯源树
样例
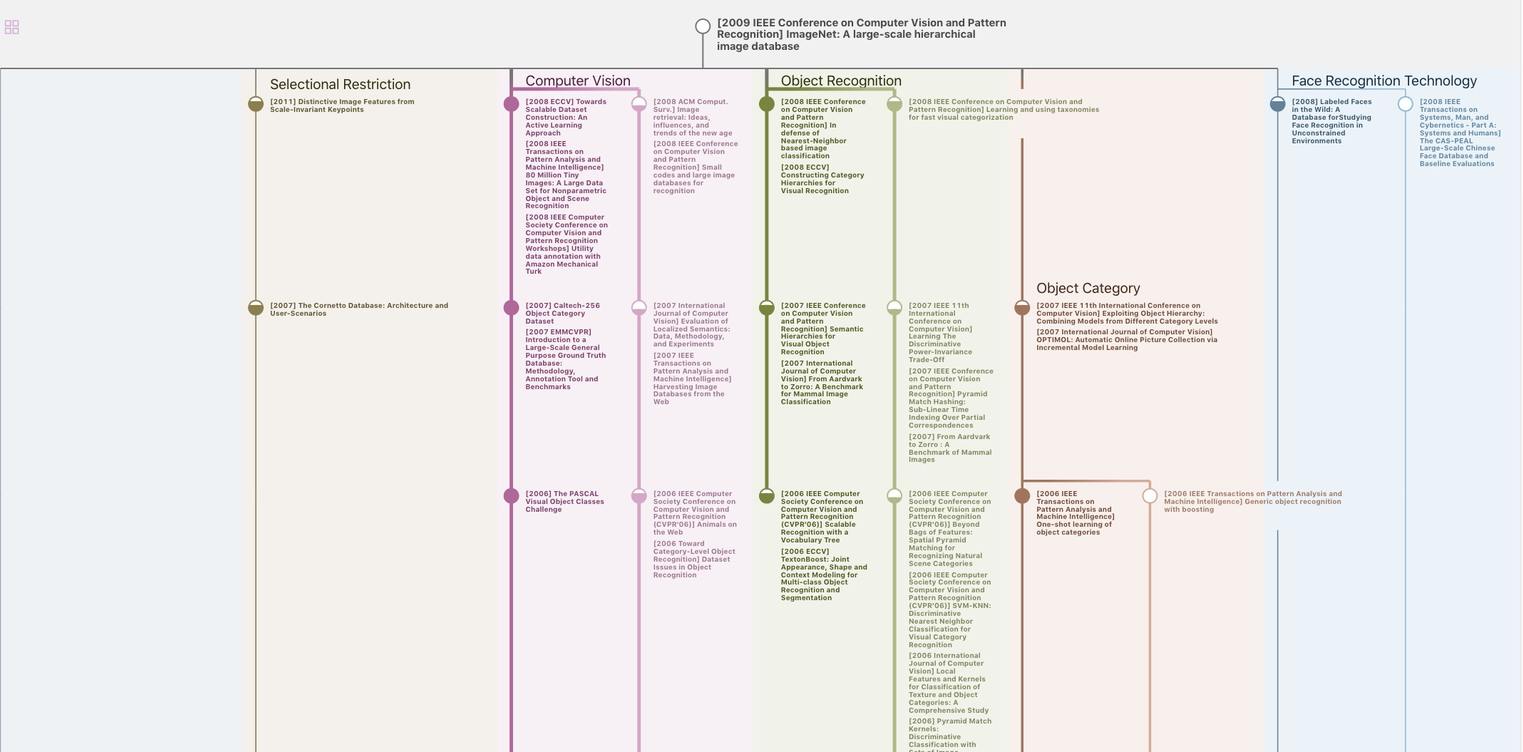
生成溯源树,研究论文发展脉络
Chat Paper
正在生成论文摘要