Learning-Based Computation Offloading for IoT Devices with Energy Harvesting.
IEEE Transactions on Vehicular Technology(2019)
摘要
Internet of Things (IoT) devices can apply mobile edge computing (MEC) and energy harvesting (EH) to provide high-level experiences for computational intensive applications and concurrently to prolong the lifetime of the battery. In this paper, we propose a reinforcement learning (RL) based offloading scheme for an IoT device with EH to select the edge device and the offloading rate according to the current battery level, the previous radio transmission rate to each edge device, and the predicted amount of the harvested energy. This scheme enables the IoT device to optimize the offloading policy without knowledge of the MEC model, the energy consumption model, and the computation latency model. Further, we present a deep RL-based offloading scheme to further accelerate the learning speed. Their performance bounds in terms of the energy consumption, computation latency, and utility are provided for three typical offloading scenarios and verified via simulations for an IoT device that uses wireless power transfer for energy harvesting. Simulation results show that the proposed RL-based offloading scheme reduces the energy consumption, computation latency, and task drop rate, and thus increases the utility of the IoT device in the dynamic MEC in comparison with the benchmark offloading schemes.
更多查看译文
关键词
Computational modeling,Energy consumption,Task analysis,Batteries,Performance evaluation,Vehicle dynamics,Mobile handsets
AI 理解论文
溯源树
样例
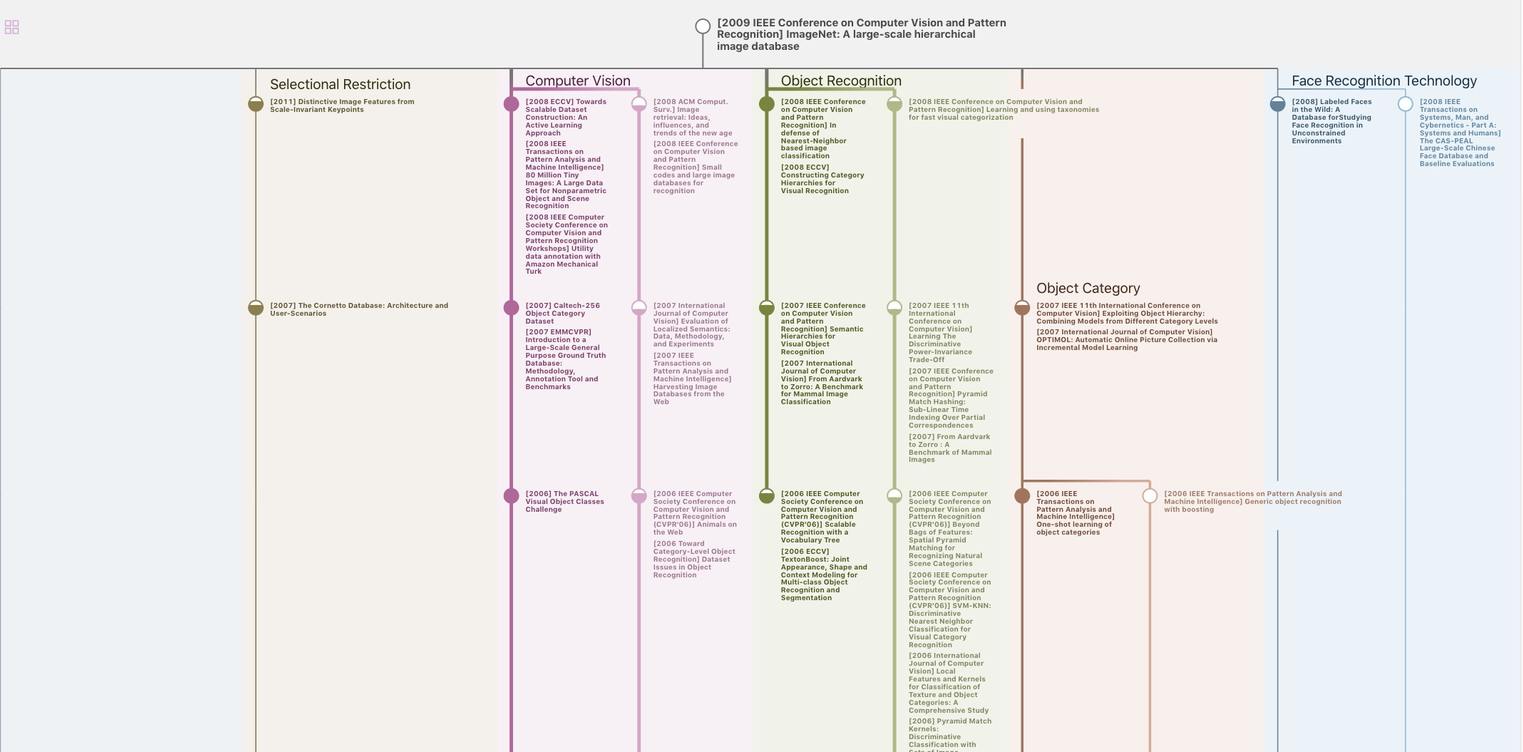
生成溯源树,研究论文发展脉络
Chat Paper
正在生成论文摘要