Recovery of point clouds on surfaces: application to image reconstruction.
arXiv (Cornell University)(2018)
摘要
We introduce a framework for the recovery of points on a smooth surface in high-dimensional space, with application to dynamic imaging. We assume the surface to be the zero-level set of a bandlimited function. We show that the exponential maps of the points on the surface satisfy annihilation relations, implying that they lie in a finite dimensional subspace. We rely on nuclear norm minimization of the maps to recover the points from noisy and undersampled measurements. Since this direct approach suffers from the curse of dimensionality, we introduce an iterative reweighted algorithm that uses the "kernel trick". The resulting algorithm has similarities to iterative algorithms used in graph signal processing (GSP); this framework can be seen as a continuous domain alternative to discrete GSP theory. The use of the algorithm in recovering free breathing and ungated cardiac data shows the potential of this framework in practical applications.
更多查看译文
关键词
denoising,dynamic MRI,kernels,machine learning,superresolution
AI 理解论文
溯源树
样例
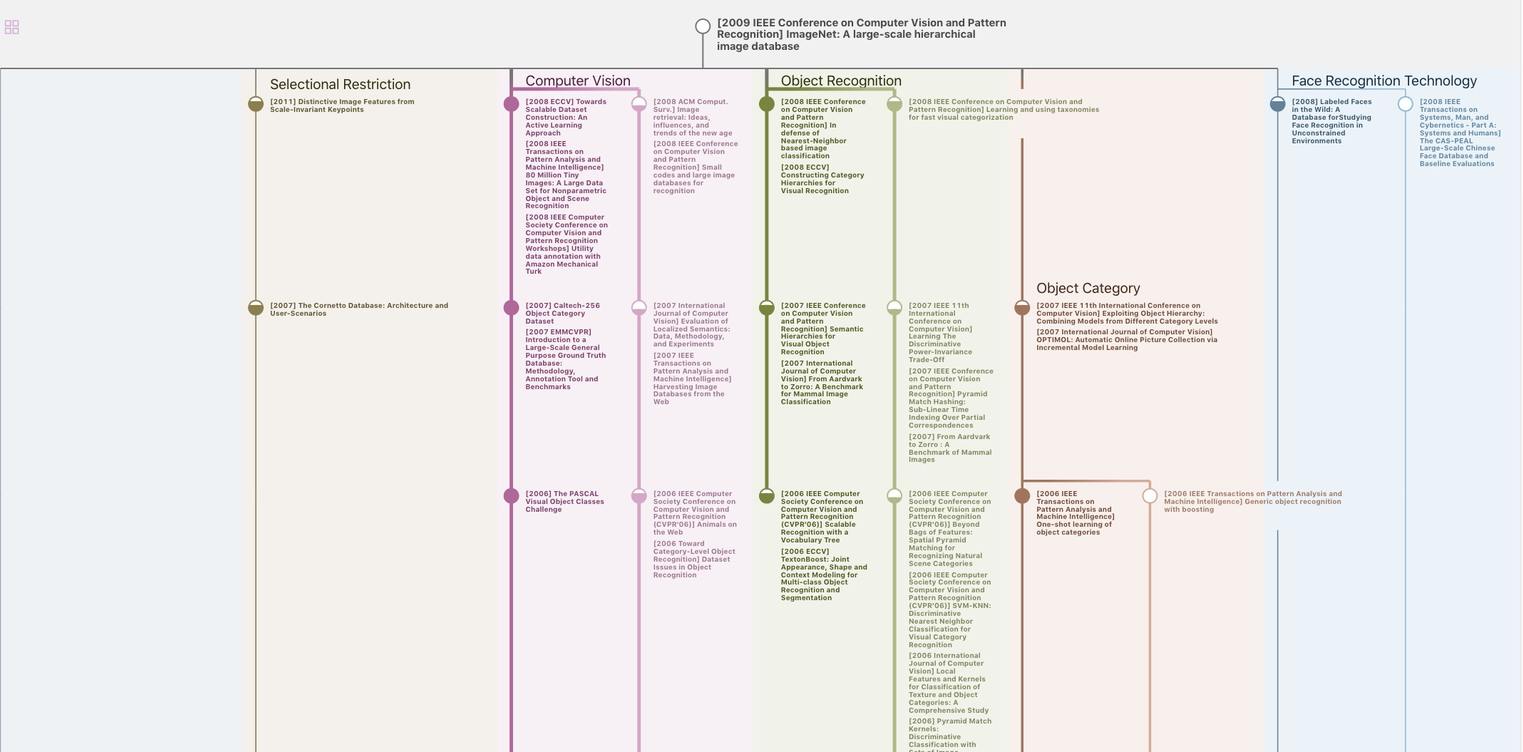
生成溯源树,研究论文发展脉络
Chat Paper
正在生成论文摘要