Learnability of edge cost functions in routing games
2017 IEEE 56TH ANNUAL CONFERENCE ON DECISION AND CONTROL (CDC)(2017)
摘要
We study the learnability of the edge cost functions in routing games from observations of the equilibrium flows, where the learnability is measured as the minimum number of samples needed to maintain a high consistency of the empirical risk, which is a statistical estimator for the quality of the learned model. To provide an upper bound on the minimum sample size, we motivate the analysis of the uniform laws of large numbers on a class of loss functions indexed over the space of parameters we want to estimate. On one hand, leveraging results on the complexity of function classes and in approximation theory, we investigate how the behavior of the empirical risk relates to a notion of complexity of the parameter space. On the other hand, using sensitivity analysis in optimization, we study how variations in the parameter space translate into variations in the class of loss functions. This allows us to show, independently of the sample distribution and of the edge costs parametrization, that the sample size required to maintain a high consistency grows quadratically with the number of edges in the network (or linearly if all the edge cost functions have the same shape), and grows linearly with the Lipschitz constant of the edge cost functions, and quadratically with the inverse of the strong monotonicity constant of the edge cost functions.
更多查看译文
关键词
edge cost functions,learnability,routing games,loss functions,function classes,parameter space,edge costs parametrization,approximation theory,sensitivity analysis,optimization,Lipschitz constant
AI 理解论文
溯源树
样例
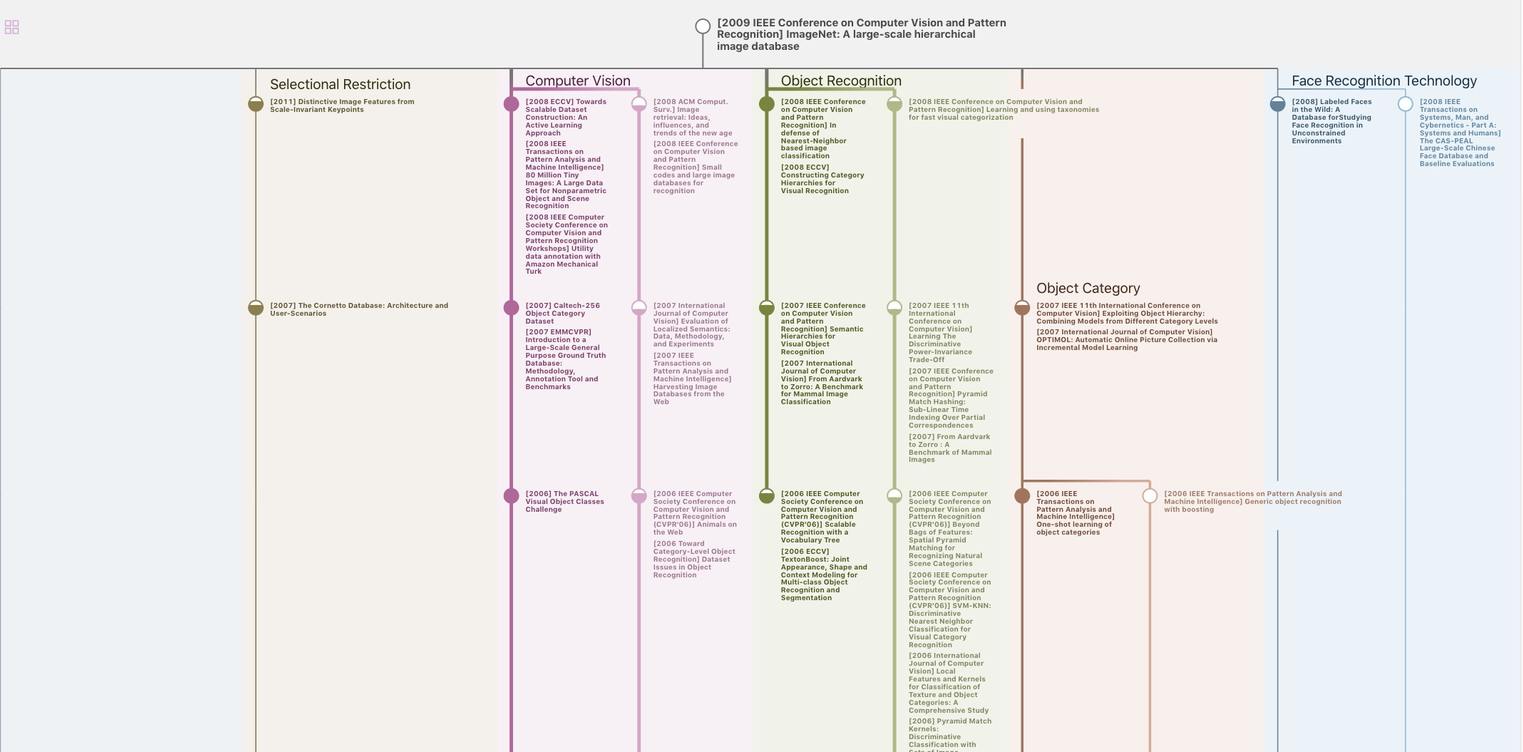
生成溯源树,研究论文发展脉络
Chat Paper
正在生成论文摘要