Multi-RAT Access Based on Multi-Agent Reinforcement Learning.
IEEE Global Communications Conference(2017)
摘要
The integration of multiple Radio Access Technologies (RATs) of licensed or unlicensed bands is considered as a cost-efficient way to greatly increase network capacity of mobile networks. In this paper, we propose a Smart Aggregated RAT Access (SARA) strategy with aim to maximize network throughput while meeting diverse traffic Quality of Service (QoS) requirements. We consider a scenario where users with different QoS requirements access to the Heterogeneous Network (HetNet) with coexisting Cellular-WiFi. In order to maximize network resource utilization in such a complex and dynamic environment, we exploit multi-agent reinforcement learning to perform RAT selection in conjunction with resource allocation for individual users, based on sensing dynamic channel states and traffic characteristics. We first use Nash Q-learning to provide a set of feasible RAT access strategies, and then employ Monte-Carlo Tree Search (MCTS) based Q-learning to perform resource allocation which tries to maximize system throughput while meeting traffic QoS requirements. Numerical results reveal that the network access capacity can be maximized while meeting traffic QoS requirements with limited number of searches by using our proposed SARA. Compared with traditional WiFi offloading schemes, SARA can significantly improve system resource utilization and capacity while guaranteeing QoS requirements of UEs.
更多查看译文
关键词
Licensed band,unlicensed band,access control,reinforcement learning,Monte-Carlo Tree search
AI 理解论文
溯源树
样例
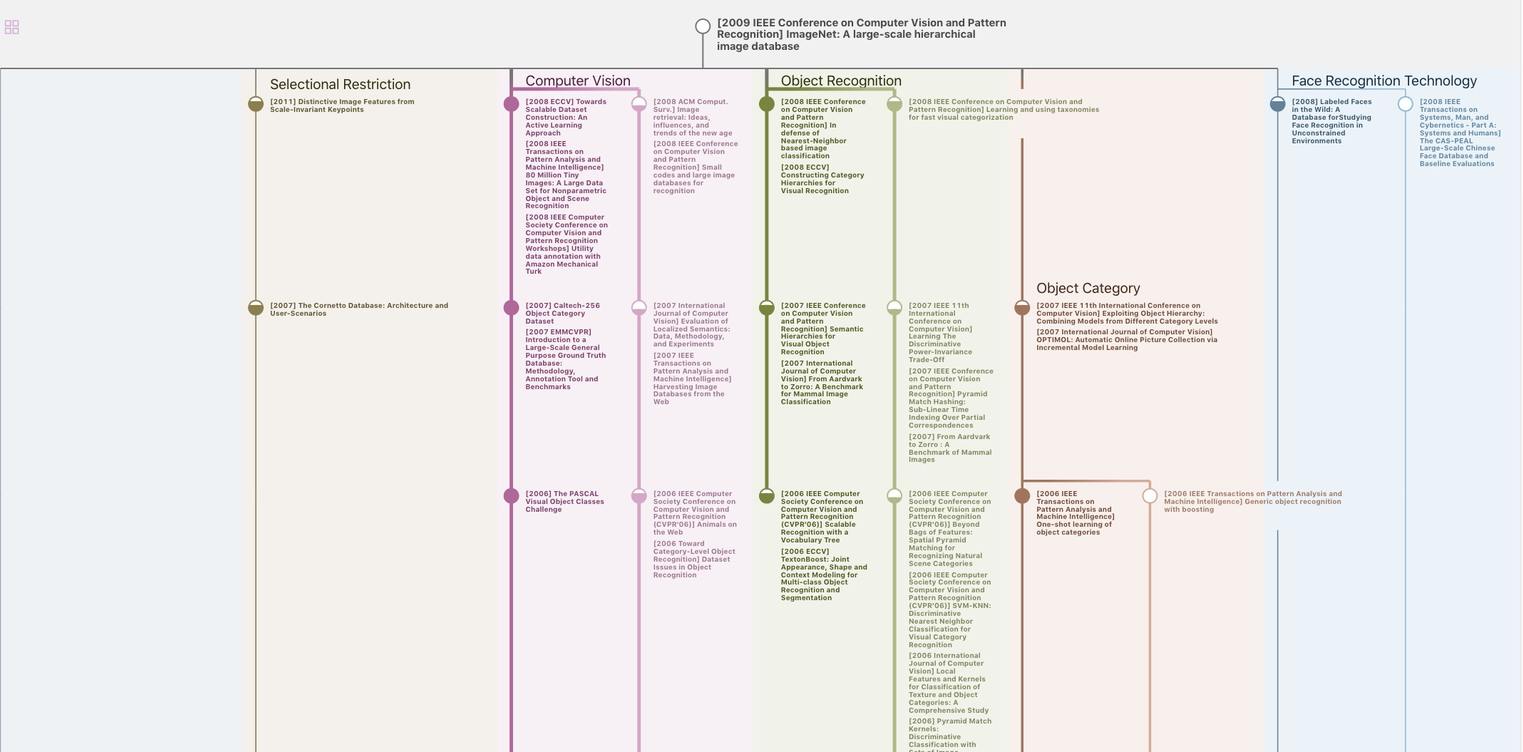
生成溯源树,研究论文发展脉络
Chat Paper
正在生成论文摘要