A Clustering Algorithm Based On Elitist Evolutionary Approach
INTERNATIONAL JOURNAL OF BIO-INSPIRED COMPUTATION(2017)
摘要
The k-means algorithm is a popular clustering algorithm. However, while k-means is convenient to implement, it produces solutions that are locally optimal. It depends on the number of clusters k and initialisation seeds. We introduce a method that can be used directly as a clustering algorithm or as an initialisation of the k-means algorithm based on the cluster number optimisation. The problem is the number of parameters required to find an optimal solution. We propose to apply diversity of population maintained through different evolutionary subpopulations and to apply the elitist strategy to select only the best concurrent solution. We also propose a new mutation strategy according to the neighbourhood search. This cooperative strategy allows us to find the global optimal solution for clustering tasks and optimal cluster seeds. We conduct numerical experiments to evaluate the effectiveness of the proposed algorithms on multi-class datasets, overlapped datasets and large-size datasets.
更多查看译文
关键词
data exploration, optimisation approach, elitist approach, clusters number, clustering, spherical clusters, ellipsoidal clusters
AI 理解论文
溯源树
样例
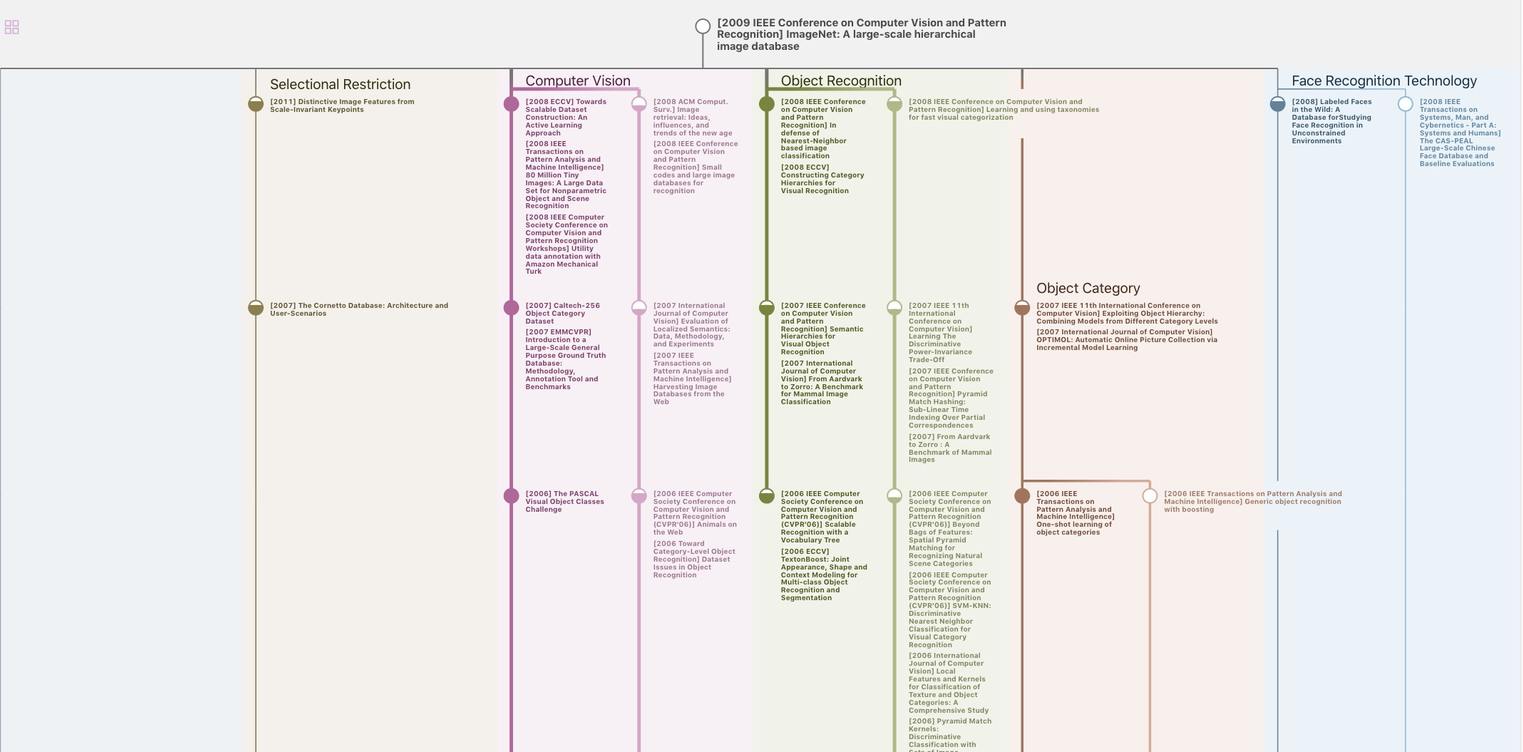
生成溯源树,研究论文发展脉络
Chat Paper
正在生成论文摘要