Annotation modification for fine-grained visual recognition.
Neurocomputing(2018)
摘要
Query modification is an intensively studied and widely used technique in information retrieval, for it helps better understand the intention of the users. In this work, we introduce this idea into fine-grained visual recognition, which is important to ambiguous queries in image retrieval task. Unlike most existing works, which incorporate information about object bounding boxes or parts for extracting discriminative local features, we propose a novel approach from a new viewpoint to solve the fine-grained recognition problem, namely annotation modification. The proposed approach fully exploits the inter-class ambiguity (which is generally regarded as noise) to form active sets of annotations for boosting the fine-grained visual recognition. Specifically, it first obtains some most confusing classes of each image through an easy-to-evaluate classifier, and then modify the annotation of each image using the active set of annotations. To handle the modified annotations, a novel ranking based loss function is further designed to learn effective classification models. We evaluate the proposed approach on three popular fine-grained image datasets (i.e., Oxford-IIIT Pets, Flower-102 and CUB200-2011), and the experimental results clearly demonstrate its effectiveness.
更多查看译文
关键词
Query modification,Annotation modification,Fine-grained visual recognition,Active set,Ranking loss
AI 理解论文
溯源树
样例
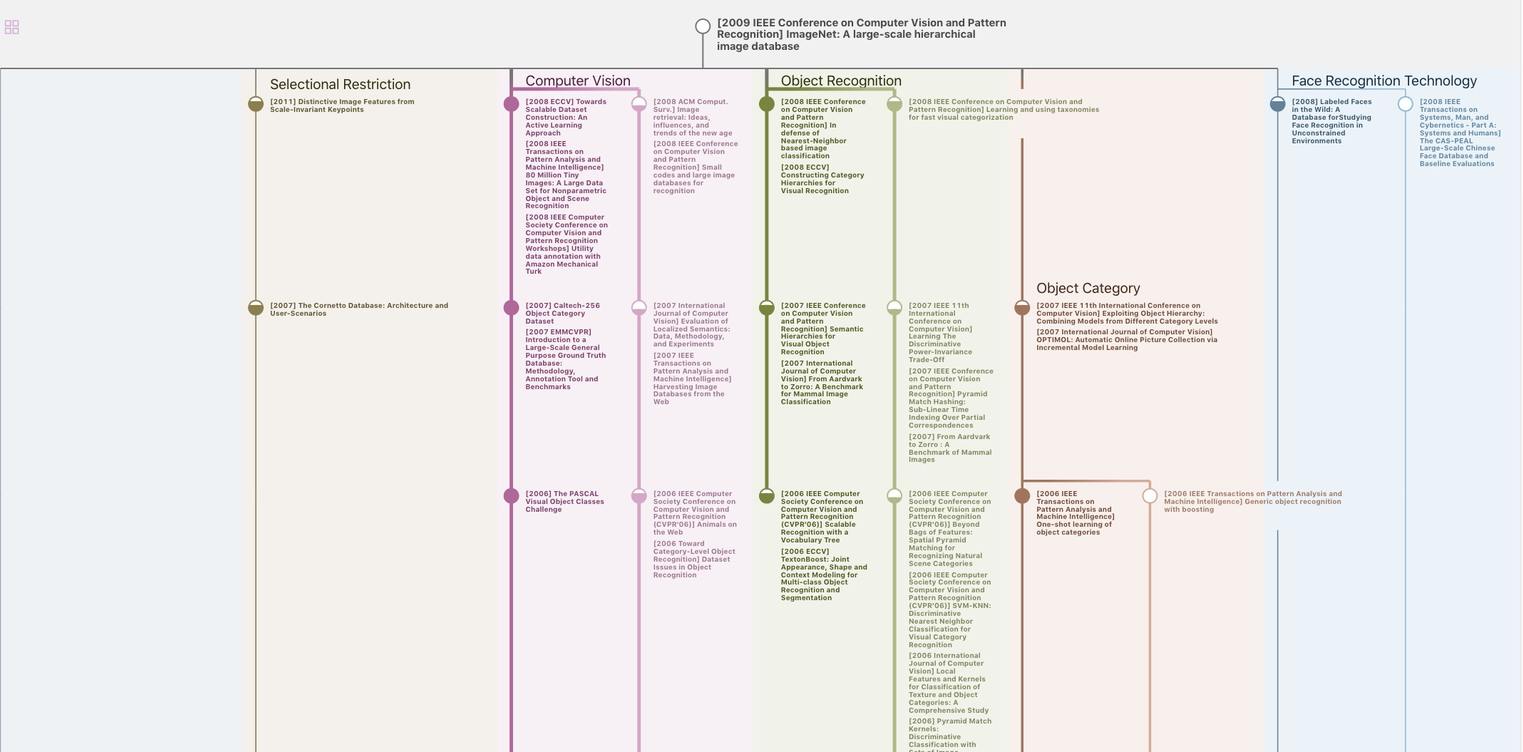
生成溯源树,研究论文发展脉络
Chat Paper
正在生成论文摘要