Cascaded deep decision networks for classification of endoscopic images.
Proceedings of SPIE(2017)
摘要
Both traditional and wireless capsule endoscopes can generate tens of thousands of images for each patient. It is desirable to have the majority of irrelevant images filtered out by automatic algorithms during an offline review process or to have automatic indication for highly suspicious areas during an online guidance. This also applies to the newly invented endomicroscopy, where online indication of tumor classification plays a significant role. Image classification is a standard pattern recognition problem and is well studied in the literature. However, performance on the challenging endoscopic images still has room for improvement. In this paper, we present a novel Cascaded Deep Decision Network (CDDN) to improve image classification performance over standard Deep neural network based methods. During the learning phase, CDDN automatically builds a network which discards samples that are classified with high confidence scores by a previously trained network and concentrates only on the challenging samples which would be handled by the subsequent expert shallow networks. We validate CDDN using two different types of endoscopic imaging, which includes a polyp classification dataset and a tumor classification dataset. From both datasets we show that CDDN can outperform other methods by about 10%. In addition, CDDN can also be applied to other image classification problems.
更多查看译文
关键词
Medical Image Classification,Colonoscopy,Confocal LASER Endoscopy,Deep Learning
AI 理解论文
溯源树
样例
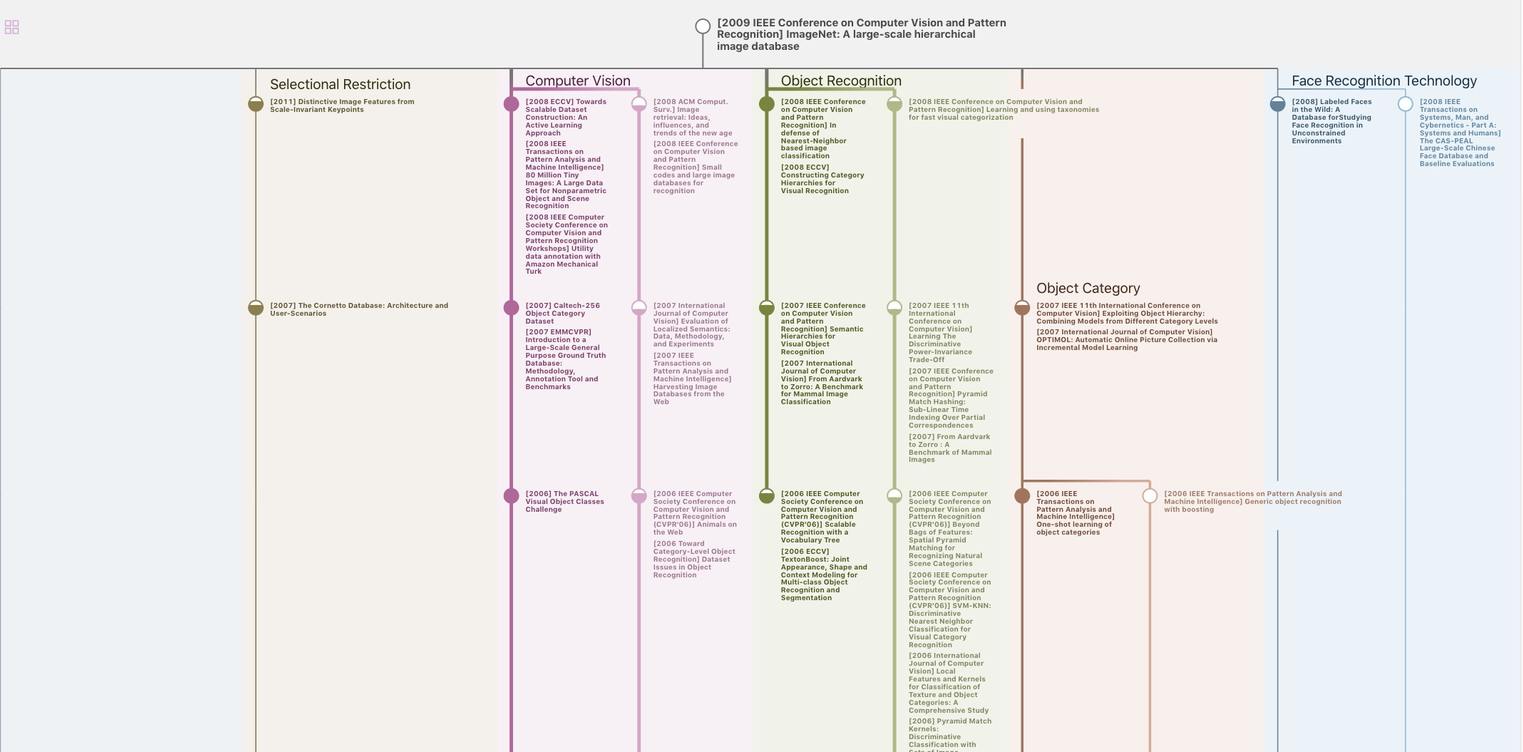
生成溯源树,研究论文发展脉络
Chat Paper
正在生成论文摘要