3D-MICE: integration of cross-sectional and longitudinal imputation for multi-analyte longitudinal clinical data.
JOURNAL OF THE AMERICAN MEDICAL INFORMATICS ASSOCIATION(2018)
摘要
Objective: A key challenge in clinical data mining is that most clinical datasets contain missing data. Since many commonly used machine learning algorithms require complete datasets (no missing data), clinical analytic approaches often entail an imputation procedure to "fill in" missing data. However, although most clinical datasets contain a temporal component, most commonly used imputation methods do not adequately accommodate longitudinal time-based data. We sought to develop a new imputation algorithm, 3-dimensional multiple imputation with chained equations (3D-MICE), that can perform accurate imputation of missing clinical time series data. Methods: We extracted clinical laboratory test results for 13 commonly measured analytes (clinical laboratory tests). We imputed missing test results for the 13 analytes using 3 imputation methods: multiple imputation with chained equations (MICE), Gaussian process (GP), and 3D-MICE. 3D-MICE utilizes both MICE and GP imputation to integrate cross-sectional and longitudinal information. To evaluate imputation method performance, we randomly masked selected test results and imputed these masked results alongside results missing from our original data. We compared predicted results to measured results for masked data points. Results: 3D-MICE performed significantly better than MICE and GP-based imputation in a composite of all 13 analytes, predicting missing results with a normalized root-mean-square error of 0.342, compared to 0.373 for MICE alone and 0.358 for GP alone. Conclusions: 3D-MICE offers a novel and practical approach to imputing clinical laboratory time series data. 3D-MICE may provide an additional tool for use as a foundation in clinical predictive analytics and intelligent clinical decision support.
更多查看译文
关键词
machine learning,imputation,missing data,electronic health record,EHR,multiple imputation with chained equations,Gaussian process,computational pathology,data mining
AI 理解论文
溯源树
样例
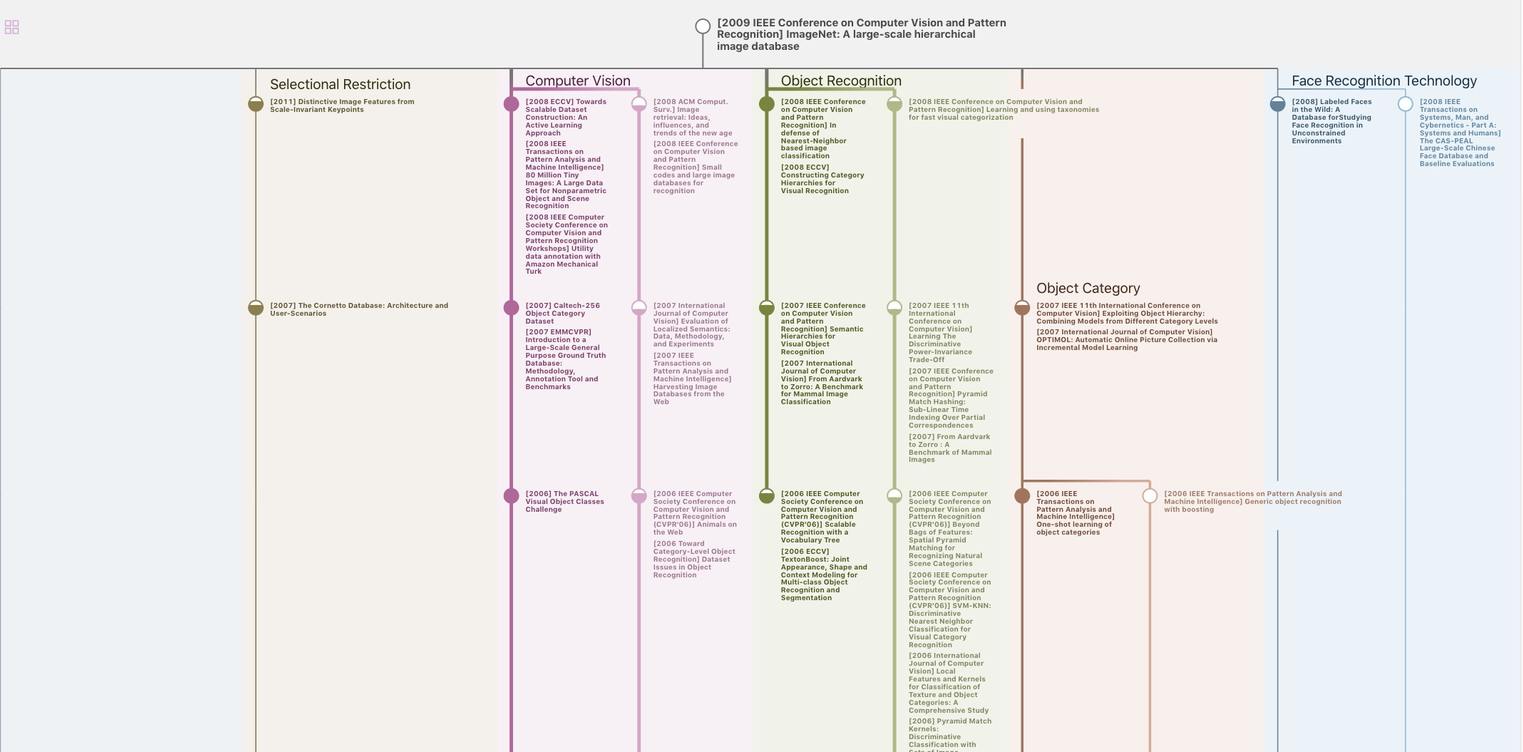
生成溯源树,研究论文发展脉络
Chat Paper
正在生成论文摘要