Updating the silent speech challenge benchmark with deep learning.
Speech Communication(2018)
摘要
The term “Silent Speech Interface” was introduced almost a decade ago to describe speech communication systems using only non-acoustic sensors, such as electromyography, ultrasound tongue imaging, or electromagnetic articulography. Although the use of specialized sensors in speech processing is challenging, silent speech research remains an active field that can often profit from new developments in traditional acoustic speech processing – for example recent advances in Deep Learning. After an overview of Silent Speech Interfaces and their special challenges, the article presents new results in which a 2010 benchmark study, called the Silent Speech Challenge, is updated with a Deep Learning strategy, using the same input features and decoding strategy as in the original Challenge article. A Word Error Rate of 6.4% is obtained with the new method, compared to the published benchmark value of 17.4%. Additional results comparing new auto-encoder-based features with the original features at reduced dimensionality, as well as decoding scenarios on two different language models, are also presented. The Silent Speech Challenge archive has furthermore been updated to contain both the original and the new auto-encoder features, in addition to the original raw data.
更多查看译文
关键词
Silent speech interface,Multimodal speech recognition,Deep learning,Language model
AI 理解论文
溯源树
样例
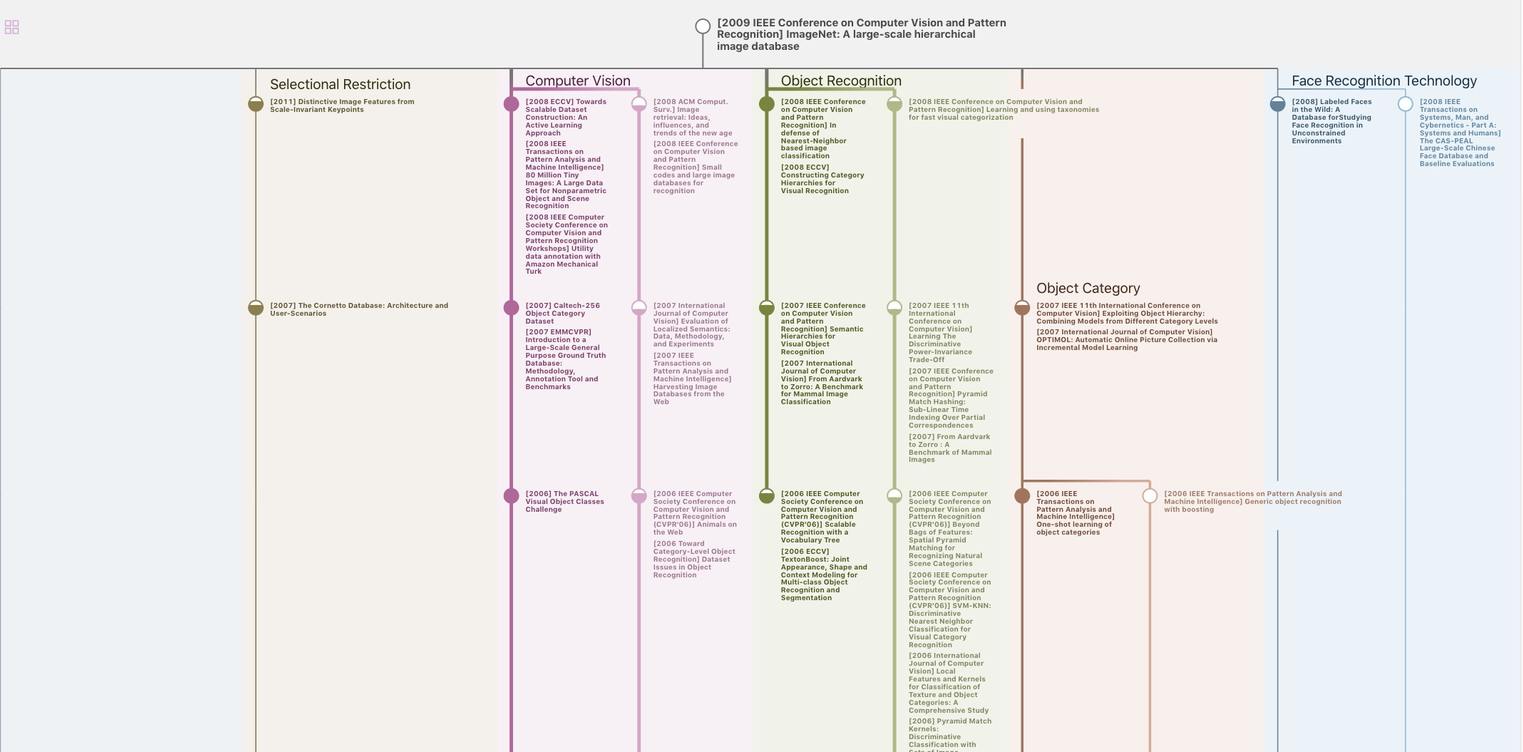
生成溯源树,研究论文发展脉络
Chat Paper
正在生成论文摘要