E2BoWs: An End-to-End Bag-of-Words Model via Deep Convolutional Neural Network for Image Retrieval
arXiv: Computer Vision and Pattern Recognition(2020)
摘要
Traditional Bag-of-Words (BoWs) model is commonly generated with many steps, including local feature extraction, codebook generation and feature quantization, etc. Those steps are relatively independent with each other and are hard to be jointly optimized. Moreover, the dependency on hand-crafted local feature makes BoWs model not effective in conveying high-level semantics. These issues largely hinder the performance of BoWs model in large-scale image applications. To conquer these issues, we propose an End-to-End BoWs (E2BoWs) model based on Deep Convolutional Neural Network (DCNN). Our model takes an image as input, then identifies and separates semantic objects in it, and finally outputs visual words with high semantic discriminative power. Specifically, our model firstly generates Semantic Feature Maps (SFMs) corresponding to different object categories through convolutional layers, then introduces Bag-of-Words Layers (BoWL) to generate visual words from each individual feature map. We also introduce a novel learning algorithm to reinforce the sparsity of the generated E2BoWs model, which further ensures the time and memory efficiency. We evaluate the proposed E2BoWs model on several image search datasets including MNIST, SVHN, CIFAR-10, CIFAR-100, MIRFLICKR-25K and NUS-WIDE. Experimental results show that our method achieves promising accuracy and efficiency compared with recent deep learning based retrieval works.
更多查看译文
关键词
Large-scale image retrieval,Bag-of-Words Model,Deep convolutional neural network
AI 理解论文
溯源树
样例
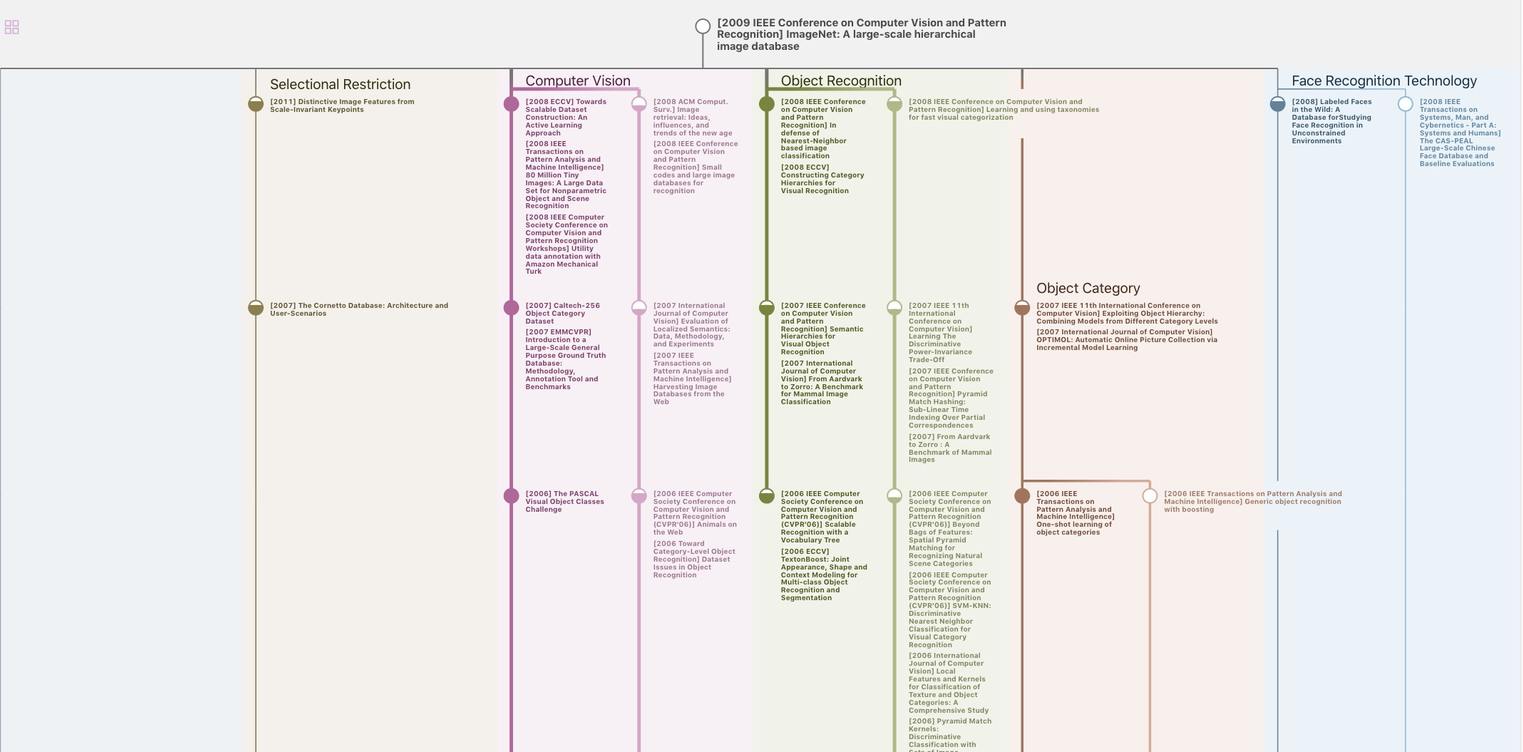
生成溯源树,研究论文发展脉络
Chat Paper
正在生成论文摘要