Large-scale user modeling with recurrent neural networks for music discovery on multiple time scales
Multimedia Tools and Applications(2017)
摘要
The amount of content on online music streaming platforms is immense, and most users only access a tiny fraction of this content. Recommender systems are the application of choice to open up the collection to these users. Collaborative filtering has the disadvantage that it relies on explicit ratings, which are often unavailable, and generally disregards the temporal nature of music consumption. On the other hand, item co-occurrence algorithms, such as the recently introduced word2vec-based recommenders, are typically left without an effective user representation. In this paper, we present a new approach to model users through recurrent neural networks by sequentially processing consumed items, represented by any type of embeddings and other context features. This way we obtain semantically rich user representations, which capture a user’s musical taste over time. Our experimental analysis on large-scale user data shows that our model can be used to predict future songs a user will likely listen to, both in the short and long term.
更多查看译文
关键词
Recommender systems,Machine learning,Recurrent neural networks,Deep learning,Word2vec,Music information retrieval,Representation learning
AI 理解论文
溯源树
样例
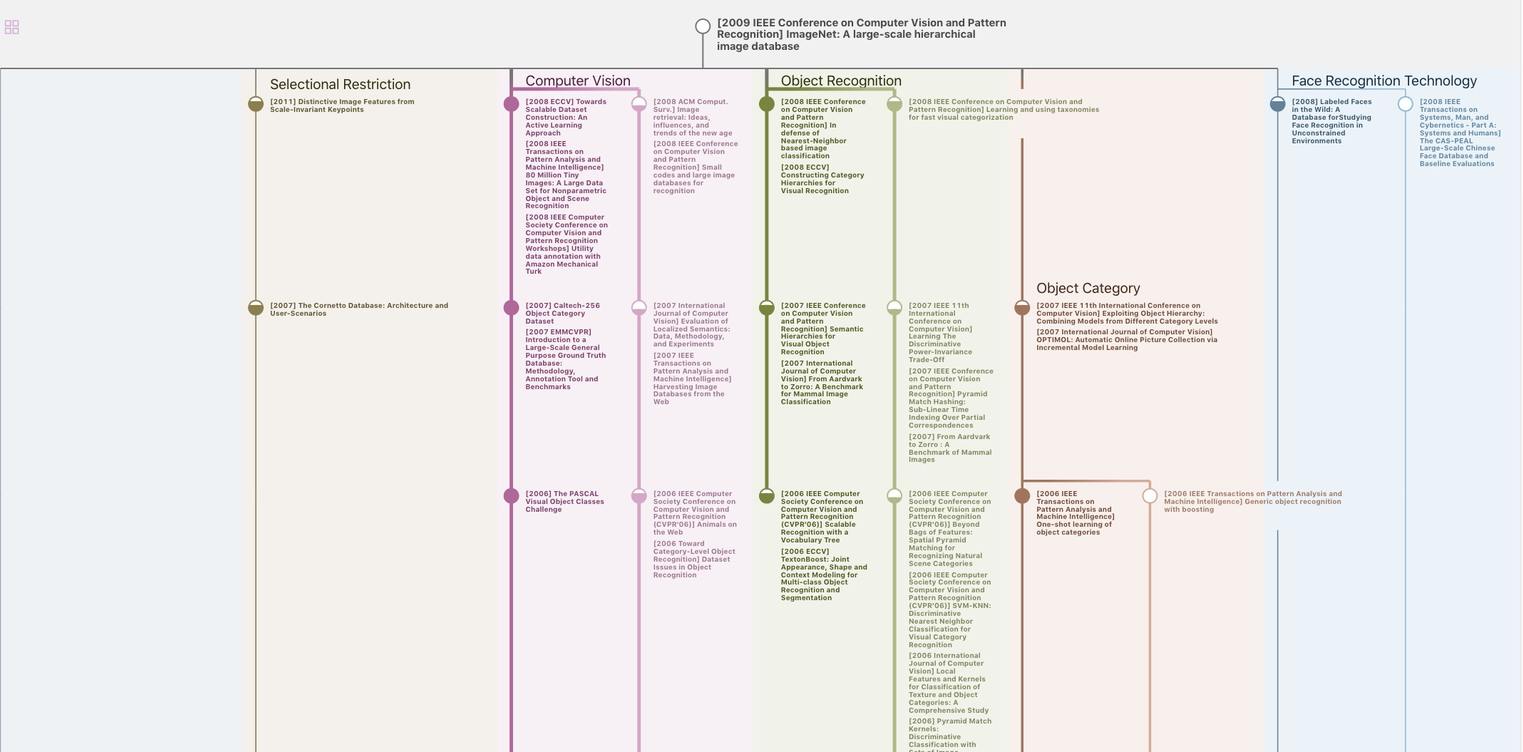
生成溯源树,研究论文发展脉络
Chat Paper
正在生成论文摘要