Squeeze-SegNet: A new fast Deep Convolutional Neural Network for Semantic Segmentation.
Proceedings of SPIE(2018)
摘要
The recent researches in Deep Convolutional Neural Network have focused their attention on improving accuracy that provide significant advances. However, if they were limited to classification tasks, nowadays with contributions from Scientific Communities who are embarking in this field, they have become very useful in higher level tasks such as object detection and pixel-wise semantic segmentation. Thus, brilliant ideas in the field of semantic segmentation with deep learning have completed the state of the art of accuracy, however this architectures become very difficult to apply in embedded systems as is the case for autonomous driving. We present a new Deep fully Convolutional Neural Network for pixel-wise semantic segmentation which we call Squeeze-SegNet. The architecture is based on Encoder-Decoder style. We use a SqueezeNet-like encoder and a decoder formed by our proposed squeeze-decoder module and upsample layer using downsample indices like in SegNet and we add a deconvolution layer to provide final multi-channel feature map. On datasets like Camvid or City-states, our net gets SegNet-level accuracy with less than 10 times fewer parameters than SegNet.
更多查看译文
关键词
Deep learning,semantic segmentation,autonomous driving,SegNet,SqueezeNet,encoder-decoder,city scenes
AI 理解论文
溯源树
样例
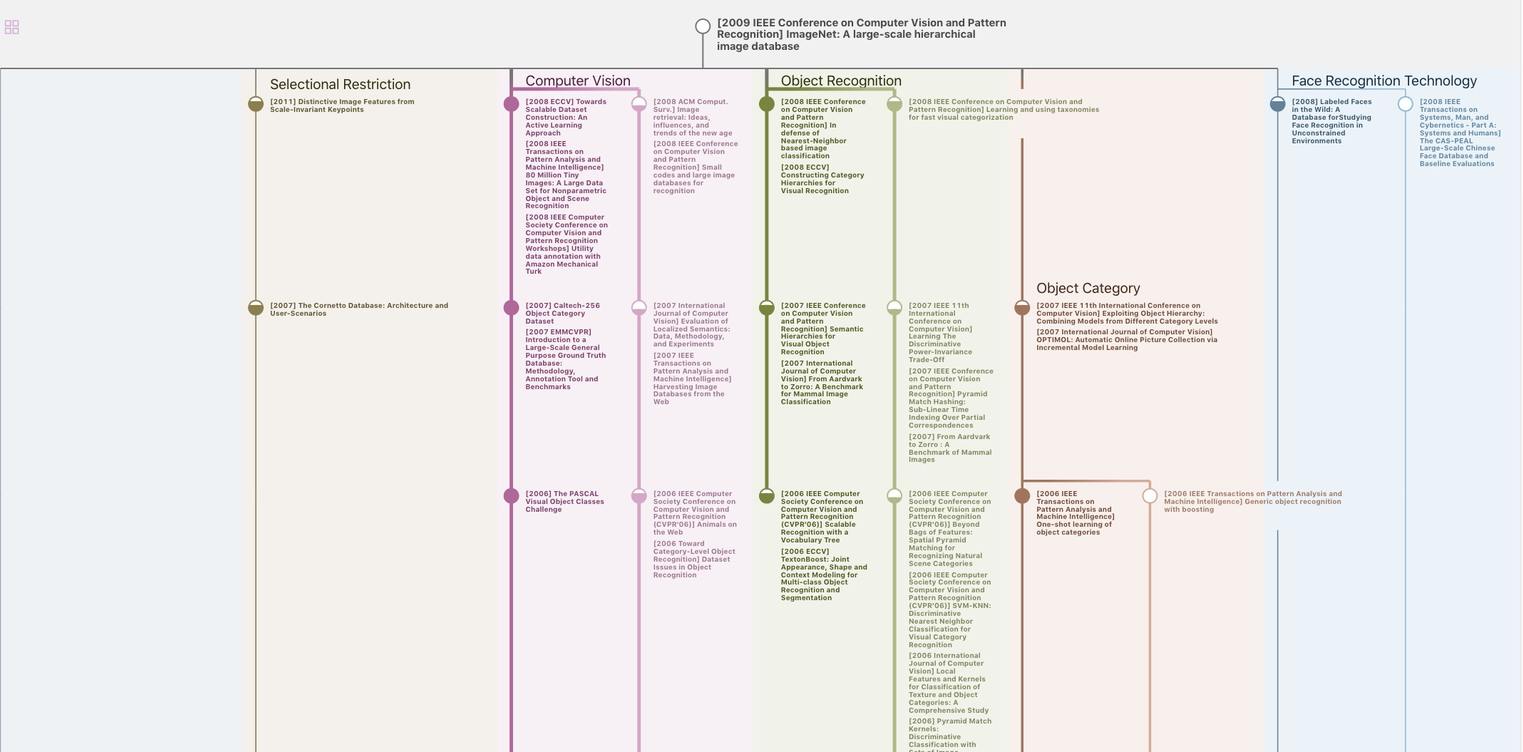
生成溯源树,研究论文发展脉络
Chat Paper
正在生成论文摘要