Differentially Private Query Learning: From Data Publishing To Model Publishing
2017 IEEE International Conference on Big Data (Big Data)(2017)
摘要
As one of the most influential privacy definitions, differential privacy provides a rigorous and provable privacy guarantee for data publishing. However, the curator has to release a large number of queries in a batch or a synthetic dataset in the Big Data era. Two challenges need to be tackled: one is how to decrease the correlation between large sets of queries, while the other is how to predict on fresh queries. This paper transfers the data publishing problem to a machine learning problem, in which queries are considered as training samples and a prediction model will be released rather than query results or synthetic datasets. When the model is published, it can be used to answer current submitted queries and predict results for fresh queries from the public. Compared with the traditional method, the proposed prediction model enhances the accuracy of query results for non-interactive publishing. We prove that learning model can successfully retain the utility of published queries while preserving privacy.
更多查看译文
关键词
differentially private query learning,model publishing,differential privacy,rigorous privacy guarantee,provable privacy guarantee,Big Data era,data publishing problem,machine learning problem,noninteractive publishing,influential privacy,Privacy Preserving Data Publishing,PPDP
AI 理解论文
溯源树
样例
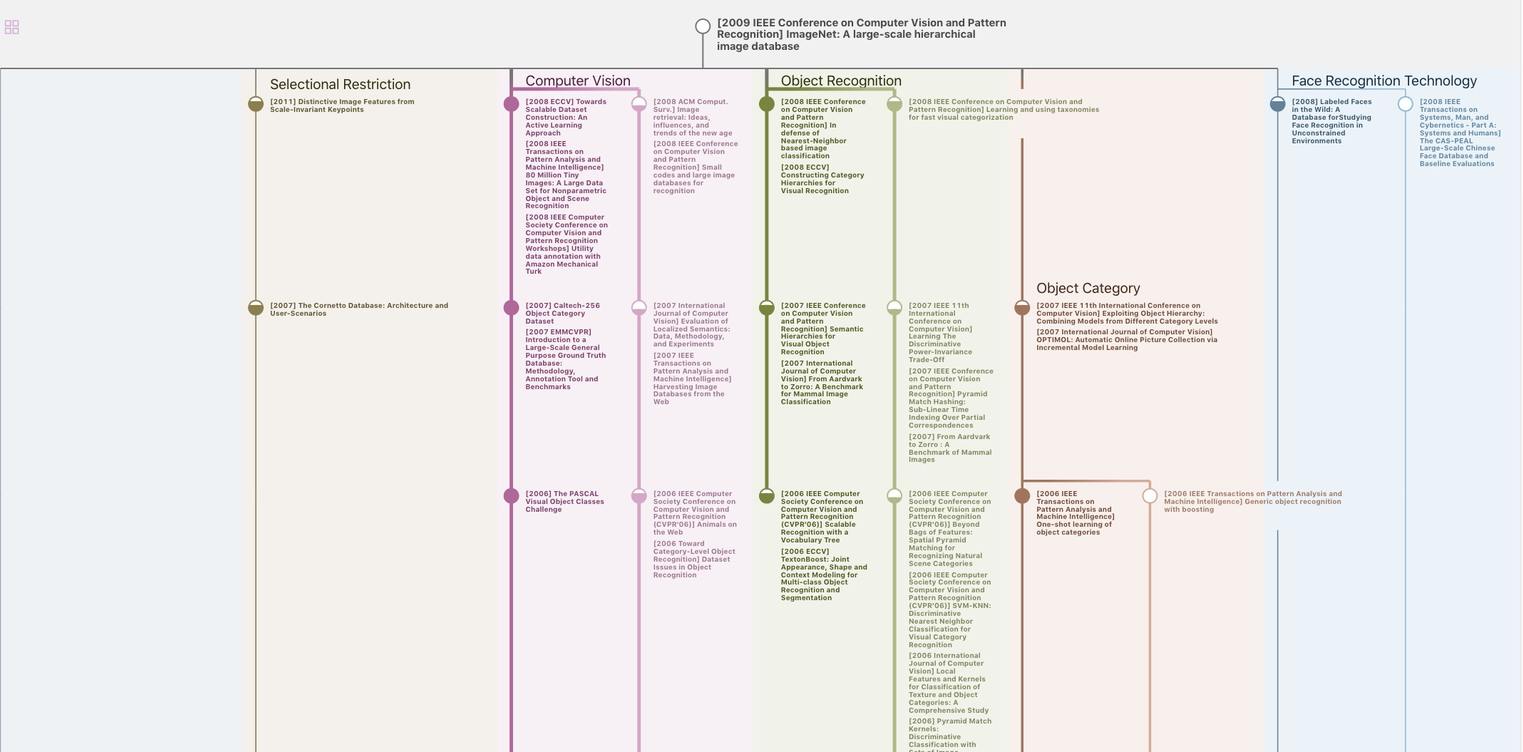
生成溯源树,研究论文发展脉络
Chat Paper
正在生成论文摘要