Visually-Aware Fashion Recommendation and Design with Generative Image Models
2017 IEEE International Conference on Data Mining (ICDM)(2017)
摘要
Building effective recommender systems for domains like fashion is challenging due to the high level of subjectivity and the semantic complexity of the features involved (i.e., fashion styles). Recent work has shown that approaches to 'visual' recommendation (e.g. clothing, art, etc.) can be made more accurate by incorporating visual signals directly into the recommendation objective, using 'off-the-shelf' feature representations derived from deep networks. Here, we seek to extend this contribution by showing that recommendation performance can be significantly improved by learning 'fashion aware' image representations directly, i.e., by training the image representation (from the pixel level) and the recommender system jointly; this contribution is related to recent work using Siamese CNNs, though we are able to show improvements over state-of-the-art recommendation techniques such as BPR and variants that make use of pretrained visual features. Furthermore, we show that our model can be used generatively, i.e., given a user and a product category, we can generate new images (i.e., clothing items) that are most consistent with their personal taste. This represents a first step towards building systems that go beyond recommending existing items from a product corpus, but which can be used to suggest styles and aid the design of new products.
更多查看译文
关键词
generative image models,semantic complexity,fashion styles,visual recommendation,visual signals,recommendation objective,off-the-shelf feature representations,deep networks,pixel level,recommender system,clothing items,recommender systems,visual features,visually-aware fashion recommendation,fashion aware image representations,image representation learning
AI 理解论文
溯源树
样例
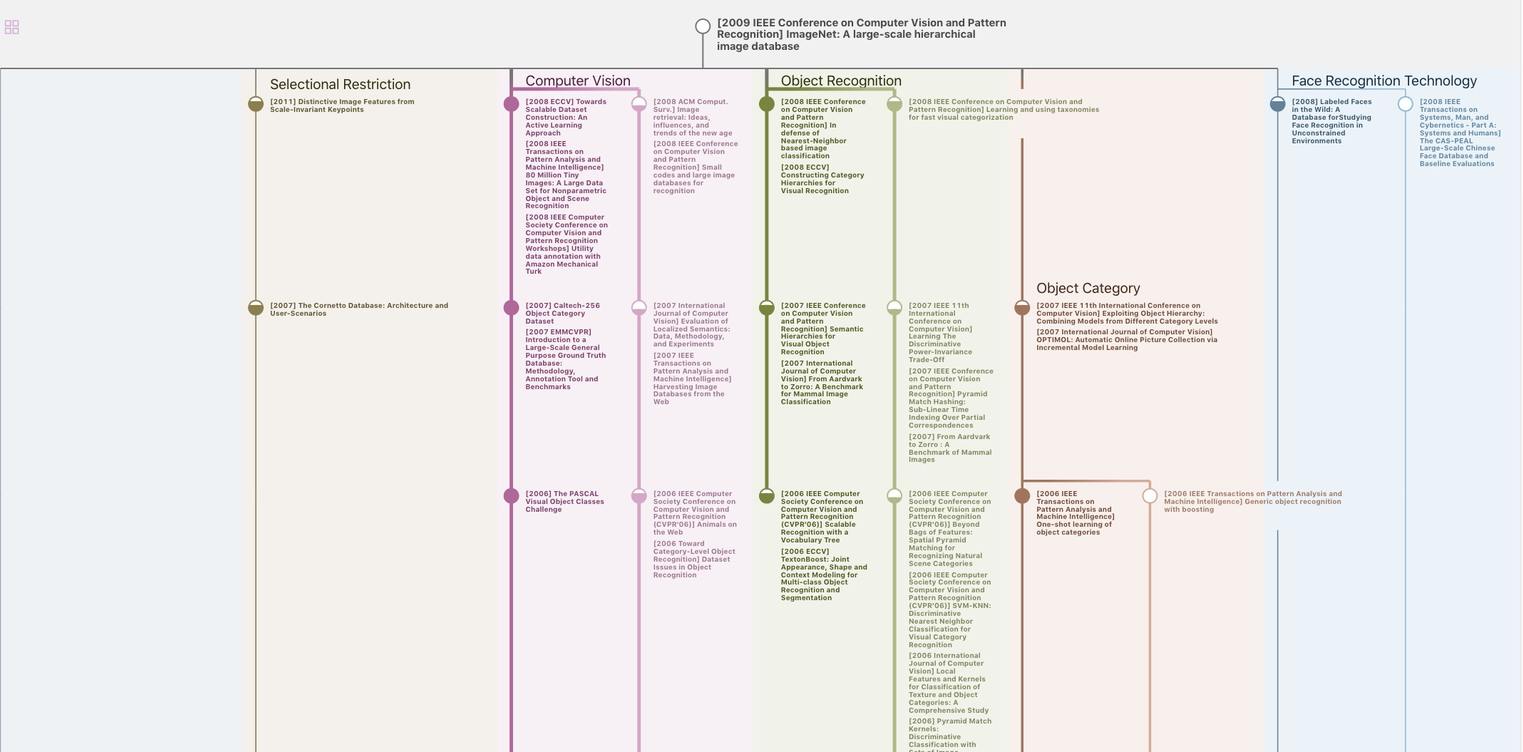
生成溯源树,研究论文发展脉络
Chat Paper
正在生成论文摘要