Lightweight adaptive Random-Forest for IoT rule generation and execution.
Journal of Information Security and Applications(2017)
摘要
The area of the Internet of Things is growing rapidly. The volume of transmitted data over the various sensors is growing accordingly. Sensors typically are low in resources of storage, memory and processing power. Data security and privacy are part of the major concerns and drawbacks of this growing domain. Sensor traffic analysis has become an increasingly important domain to protect IoT infrastructures from intruders. An IoT network intrusion detection system is required to monitor and analyze the traffic and predict possible attacks. Machine leaning techniques can automatically extract normal and abnormal patterns from a large set of training sensors data. Due to the high volume of traffic and the need for real-time reaction, accurate threat discovery is mandatory. This work focuses on designing a lightweight comprehensive IoT rules generation and execution framework. It is composed of three components, a machine learning rule discovery, a threat prediction model builder and tools to ensure timely reaction to rules violation and un-standardized and ongoing changes in traffic behavior. The generated detection model is expected to identify in real-time exceptions and notify the system accordingly. We use Random-Forest (RF) as the machine learning platform for rules discovery and real-time anomaly detection. To allow RF adaptation to IoT we propose several improvements to make it lightweight and propose a process that combines IoT network capabilities; messaging and resource sharing, to build a comprehensive and efficient IoT security framework.
更多查看译文
关键词
Internet of Things,Security,Rules extraction,Random-Forest,Active learning
AI 理解论文
溯源树
样例
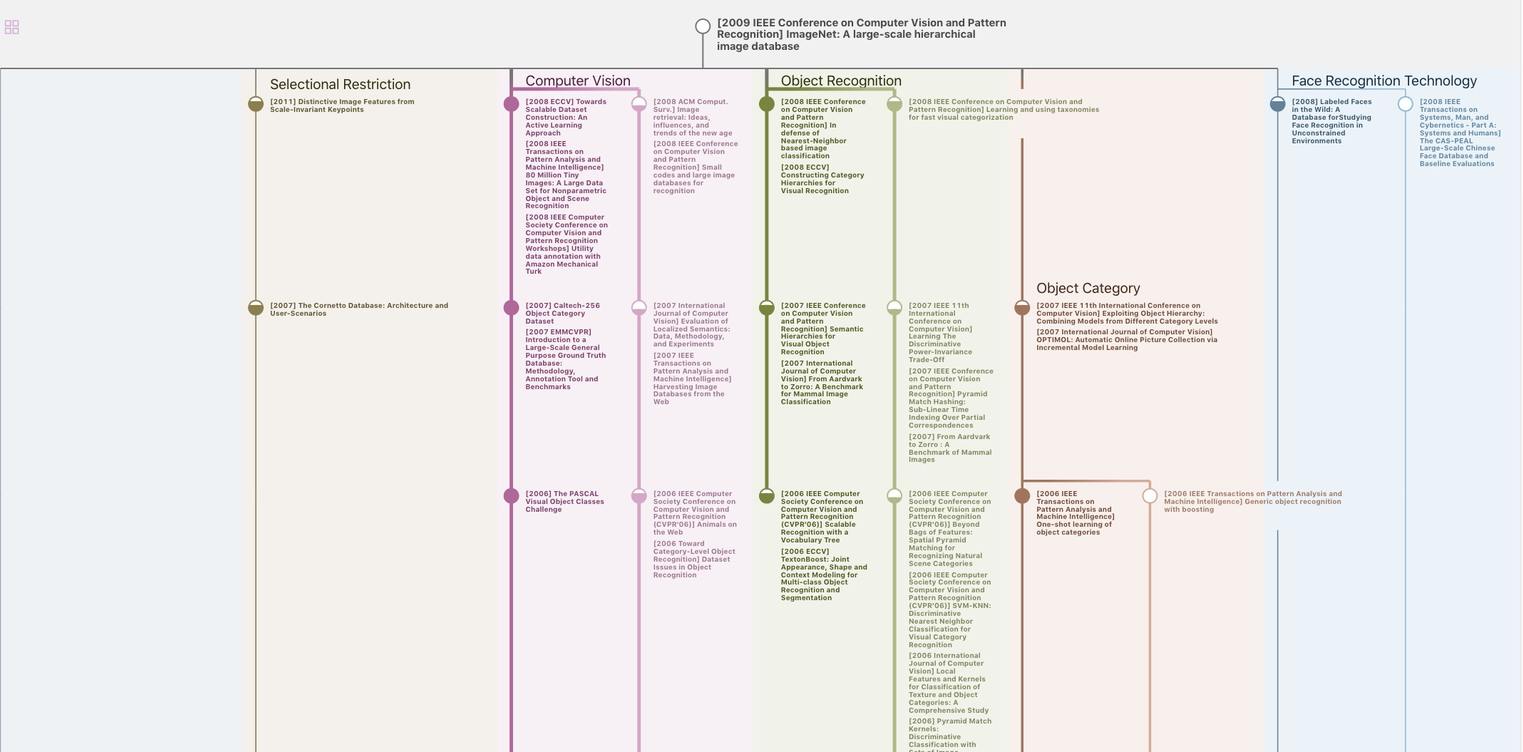
生成溯源树,研究论文发展脉络
Chat Paper
正在生成论文摘要