Second-Order Stochastic Optimization for Machine Learning in Linear Time.
JOURNAL OF MACHINE LEARNING RESEARCH(2017)
摘要
First-order stochastic methods are the state-of-the-art in large-scale machine learning optimization owing to efficient per-iteration complexity. Second-order methods, while able to provide faster convergence, have been much less explored due to the high cost of computing the second-order information. In this paper we develop second-order stochastic methods for optimization problems in machine learning that match the per-iteration cost of gradient based methods, and in certain settings improve upon the overall running time over popular first-order methods. Furthermore, our algorithm has the desirable property of being implementable in time linear in the sparsity of the input data.
更多查看译文
关键词
second-order optimization,convex optimization,regression
AI 理解论文
溯源树
样例
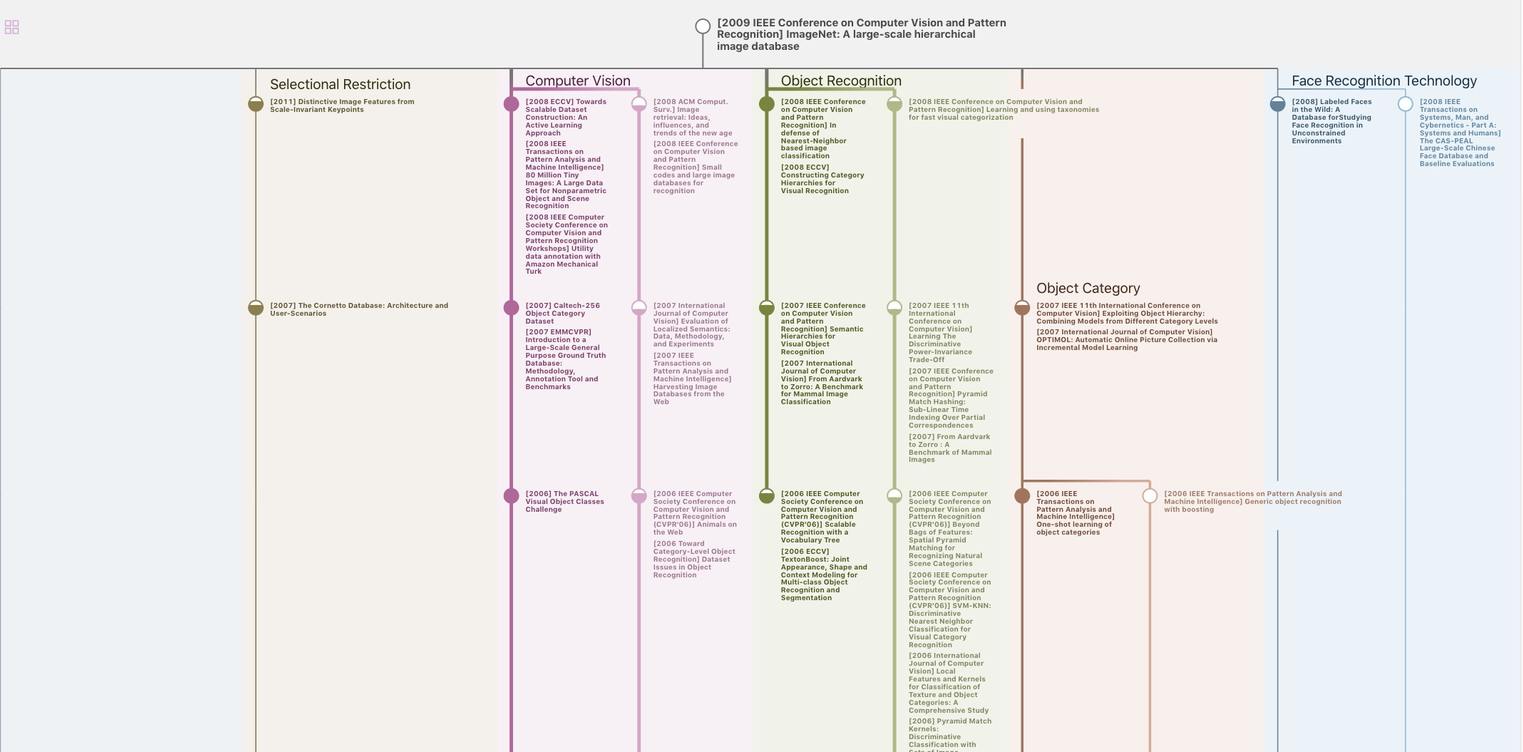
生成溯源树,研究论文发展脉络
Chat Paper
正在生成论文摘要