Challenging The Limits: Sampling Online Social Networks With Cost Constraints
IEEE INFOCOM 2017 - IEEE CONFERENCE ON COMPUTER COMMUNICATIONS(2017)
摘要
Graph sampling techniques via random walk crawling have been popular for analyzing statistical characteristics of large online social networks due to simple implementation and provable guarantees on unbiased estimates. Despite the growing popularity, the 'cost' of sampling and its true impact on the accuracy of estimates still have not been carefully studied. In addition, the random walk-based methods inherently suffer from the sluggish nature of random walks and the 'slow-mixing' structure of social graphs, thereby leading to high correlation in the samples obtained. With these in mind, in this paper, we develop a mathematical framework such that the cost of sampling is properly taken into account, which in turn re-defines a widely used asymptotic variance into a cost-based asymptotic variance. Our new metric enables us to compare a class of sampling policies under the same cost constraint, integrating "random skipping" (bypassing nodes without sampling) into the random walk-based sampling. We obtain an optimal policy striking the right balance between sampling quality (less correlation) and sampling quantity (higher cost per sample), which greatly improves over the usual skip-free crawling-based samplers. We further extend our framework, enabling one to design more sophisticated sampling strategies with an array of control knobs, which all produce unbiased estimates under the same cost constraint.
更多查看译文
关键词
sampling strategies,cost-based asymptotic variance,random walk-based sampling,sampling quantity,skip-free crawling-bsaed samplers,online social networks sampling,provable guarantees,simple implementation,statistical characteristics,random walk crawling,graph sampling,sampling quality,random skipping,cost constraint,sampling policies,social graphs,slow-mixing structure,unbiased estimates
AI 理解论文
溯源树
样例
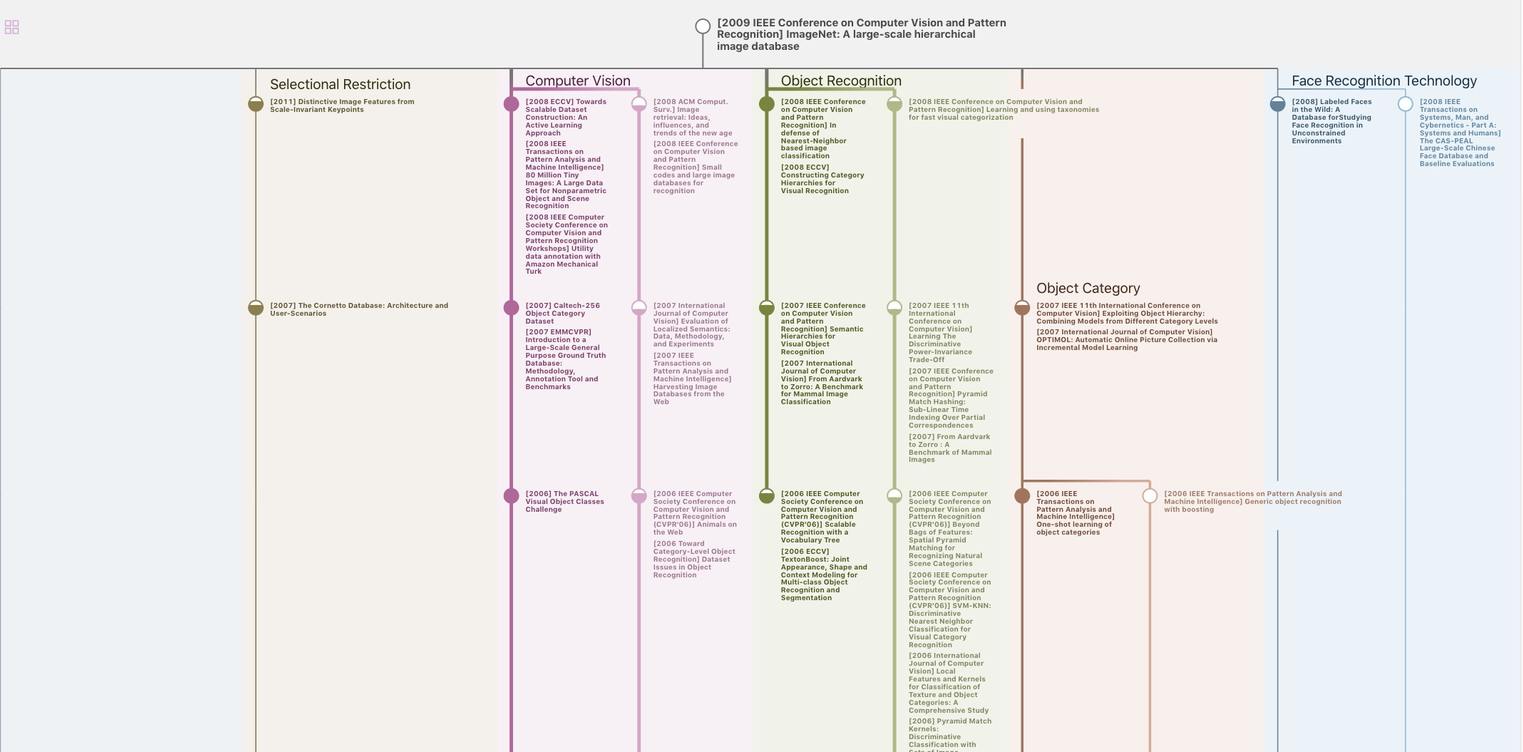
生成溯源树,研究论文发展脉络
Chat Paper
正在生成论文摘要