Position Article on Integrating Data and Model to Understand Disease Interactions
2017 IEEE International Conference on Healthcare Informatics (ICHI)(2017)
摘要
Comorbidities - cases in which patients have two or more chronic conditions - impose burden on the health care system as well as society. Causal relationships and interaction among different diseases in the comorbidity set is complex, and not yet completely understood by the medical community. Understanding the causality between diseases is an essential element of science of medicine. Patient treatment would also be more efficient if better knowledge of causality was available. There are different approaches to shed more lights on causality in medicine. In this article, we propose two approaches. One is using statistical causal inference algorithms on electronic medical data to identify potential causal relationships among diseases. In the second approach, we use qualitative modeling techniques to build models of disease mechanisms. Each one of these directions has its own pitfalls. The assumption is integrating the two approaches will minimize the drawbacks of each. The integration involves using qualitative models of underlying disease mechanisms to evaluate and explain the potential causal relationships resulted from the causal inference algorithms. This integration is complex, and require big effort from the community. In this article, we are proposing new research direction based on our preliminary work.
更多查看译文
关键词
Electronic Medical Records,Statistical Causal Inferences,Qualitative Models,Comorbidities,Disease Mechanism
AI 理解论文
溯源树
样例
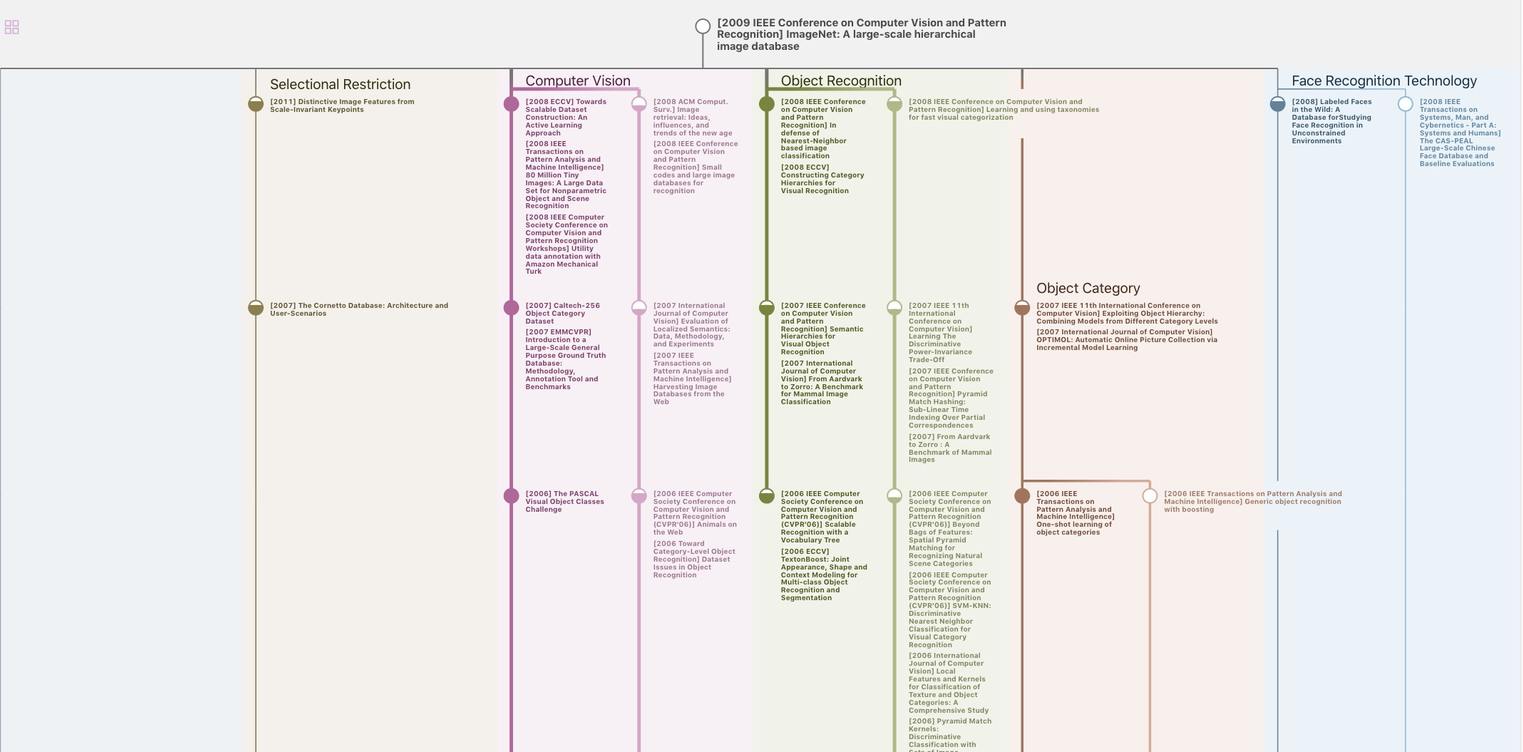
生成溯源树,研究论文发展脉络
Chat Paper
正在生成论文摘要