A modular analysis of adaptive (non-)convex optimization: Optimism, composite objectives, variance reduction, and variational bounds
ALT(2020)
摘要
Recently, much work has been done on extending the scope of online learning and incremental stochastic optimization algorithms. In this paper we contribute to this effort in two ways: First, based on a generalization of Bregman divergences and a generic regret decomposition, we provide a self-contained, modular analysis of the two workhorses of online learning: (general) adaptive versions of Mirror Descent (MD) and the Follow-the-Regularized-Leader (FTRL) algorithms. The analysis is done with extra care so as not to introduce assumptions not needed in the proofs and allows to combine, in a straightforward way, different algorithmic ideas (e.g., adaptivity, optimism, implicit updates, variance reduction) and learning settings (e.g., strongly convex or composite objectives). This way we are able to reprove, extend and refine a large body of the literature, while keeping the proofs concise. The second contribution is a by-product of this careful analysis: We present algorithms with improved variational bounds for smooth, composite objectives, including a new family of optimistic MD algorithms with only one projection step per round. Furthermore, we provide a simple extension of adaptive regret bounds to a class of practically relevant non-convex problem settings (namely, star-convex loss functions and their extensions) with essentially no extra effort.
更多查看译文
关键词
Online learning,Stochastic optimization,Adaptive algorithms,Regret bound,Follow the regularized leader,Mirror descent
AI 理解论文
溯源树
样例
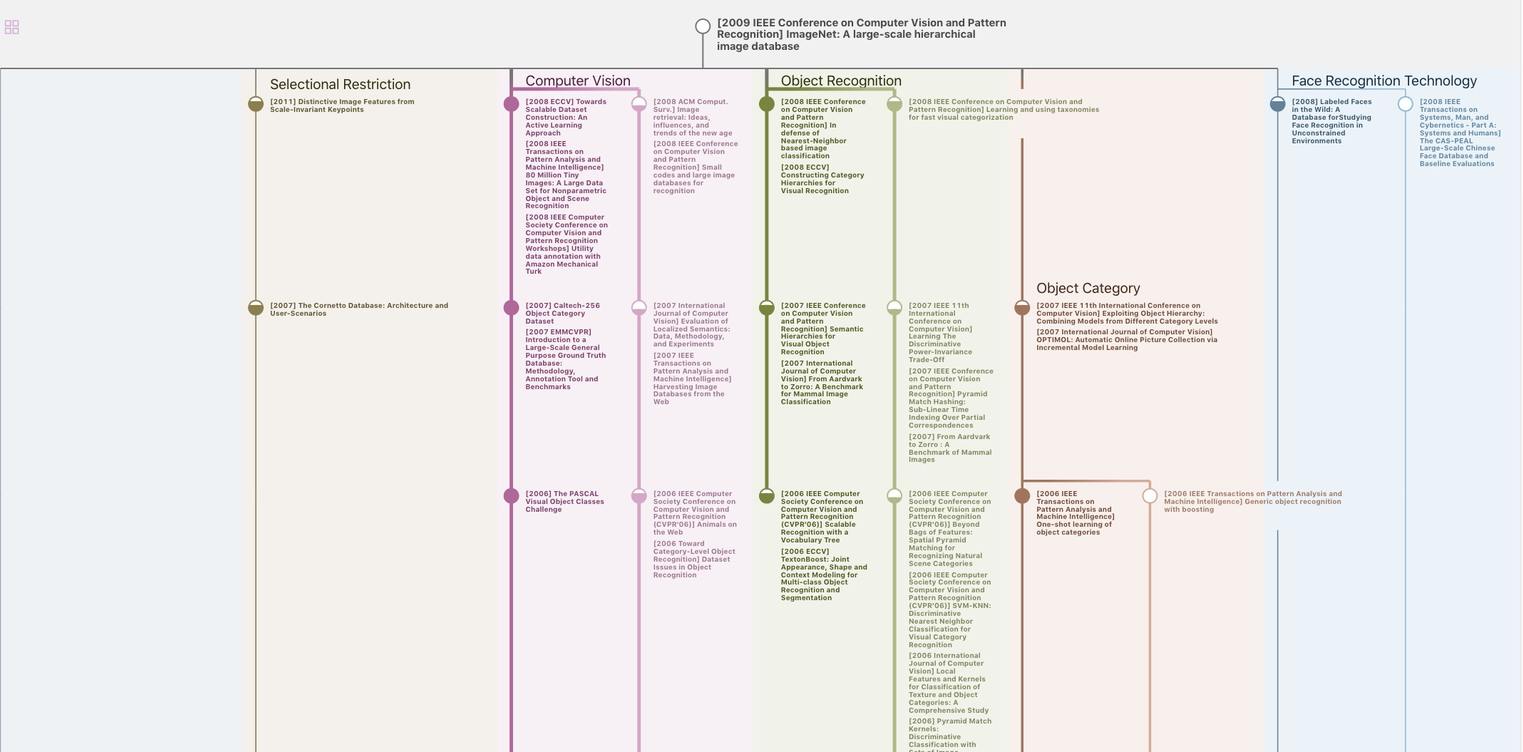
生成溯源树,研究论文发展脉络
Chat Paper
正在生成论文摘要