Predictability and Prediction of Human Mobility Based on Application-Collected Location Data
2017 IEEE 14th International Conference on Mobile Ad Hoc and Sensor Systems (MASS)(2017)
摘要
In the modern information society, accurate prediction of human mobility becomes increasingly essential in various areas such as city planning and resource management. With users' historical trajectories, the inherent patterns of their movements can be extracted and utilized to accurately predict the future movements. In this paper, based on a dataset of 100,000 individuals' actively uploaded location information collected by apps, we discover the average theoretical limits of the predictability to be as high as 93%. Since the app-collected data contains the physical context of the location, we implement a clustering method based on the contextual information that cluster the locations into three divisions, street, district and region. In order to solve the unevenly distribution and the high missing rate of the application collected location data, we firstly use the Gibbs sampling algorithm to complete the missing data of the trajectory and then employ a high-order Markov chain model to predict the most likely locations visited by each user. Result shows that our prediction algorithm can achieve accuracy as high as 67%, 78%, 87% for the three context-based divisions respectively, which are 10% higher on average than the divisions without context. In addition, the correlation coefficient between prediction accuracy and predictability reaches as high as 0.86. Finally, we investigate various factors including spatial and temporal resolution, orders of Markov models, radius of gyration, in order to explore the predictability under different circumstances.
更多查看译文
关键词
contextual information,high missing rate,high-order Markov chain model,prediction algorithm,human mobility,application-collected location data,modern information society,city planning,resource management,clustering method,Gibbs sampling algorithm
AI 理解论文
溯源树
样例
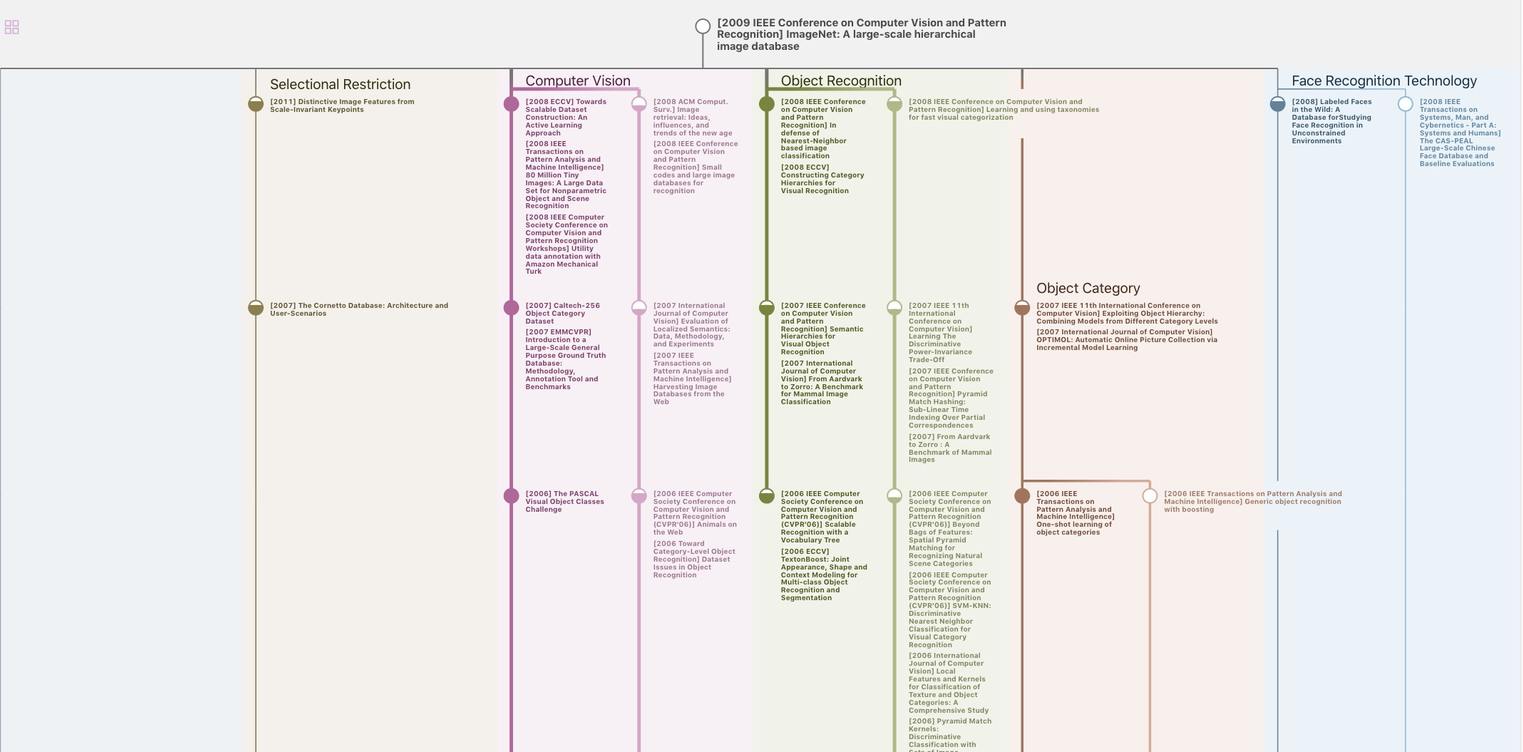
生成溯源树,研究论文发展脉络
Chat Paper
正在生成论文摘要