Analyzing Microscopic Images Of Peripheral Blood Smear Using Deep Learning
DEEP LEARNING IN MEDICAL IMAGE ANALYSIS AND MULTIMODAL LEARNING FOR CLINICAL DECISION SUPPORT(2017)
摘要
This paper presents a new automated peripheral blood smear analysis system, Shonit (TM) [1]. It consists of an automated microscope for capturing microscopic images of a blood sample, and a software component for analysis of the images. The software component employs an ensemble of deep learning models to analyze peripheral blood smear images for localization and classification of the three major blood cell types (red blood cells, white blood cells and platelets) and their subtypes [2]. We present the results of the classification and segmentation on a large variety of blood samples. The specificity and sensitivity of identification for the common cell types were above 98% and 91% respectively. The primary advantage of Shonit (TM) over other automated blood smear analysis systems [3-5] is its robustness to quality variation in the blood smears, and the low cost of its image capture device.
更多查看译文
关键词
Peripheral Blood Smear (PBS), Deep Learning Techniques, Image Capture Device, Common Cell Type, Convolution Neural Network (CNNs)
AI 理解论文
溯源树
样例
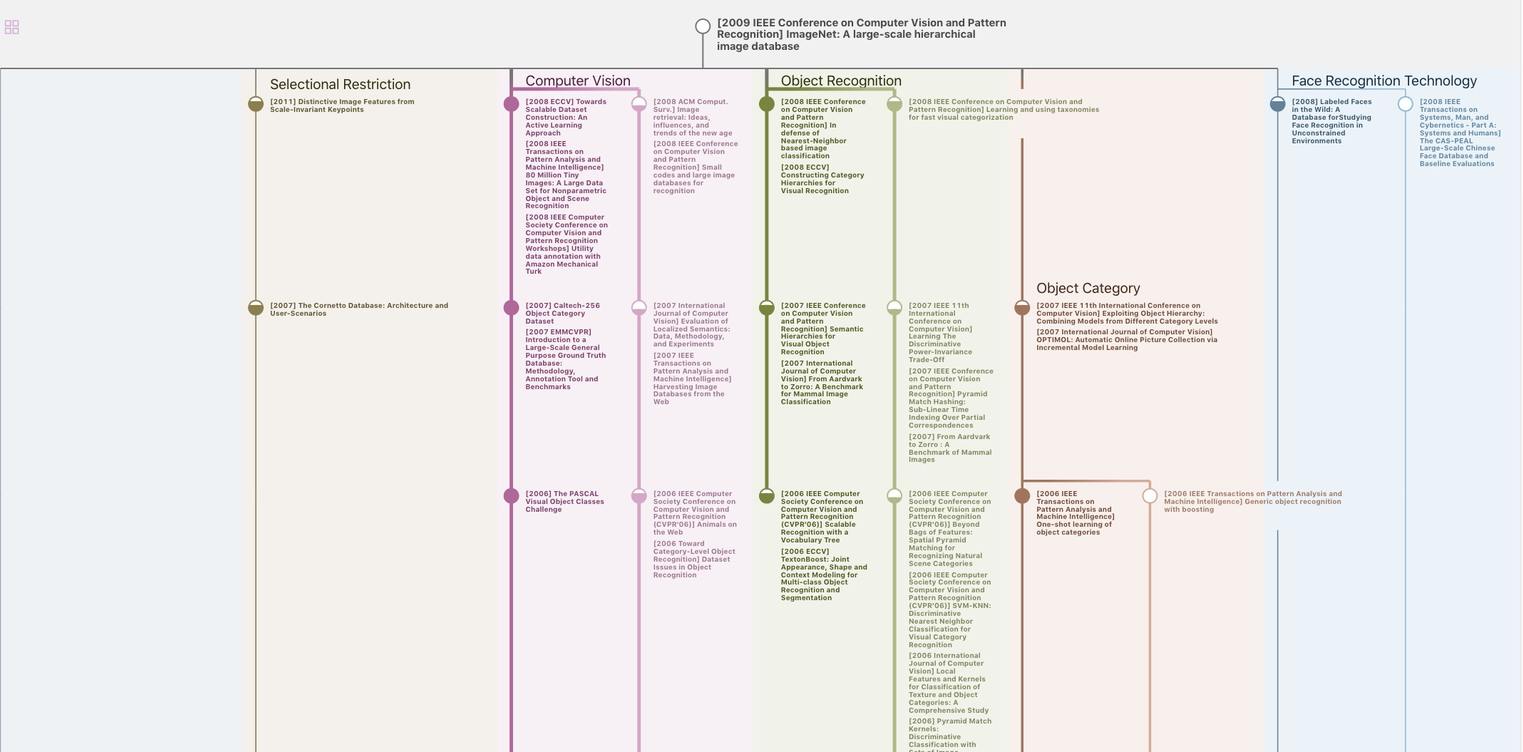
生成溯源树,研究论文发展脉络
Chat Paper
正在生成论文摘要